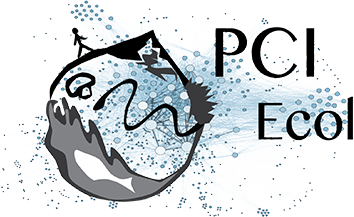
When does seasonal reproduction evolve?
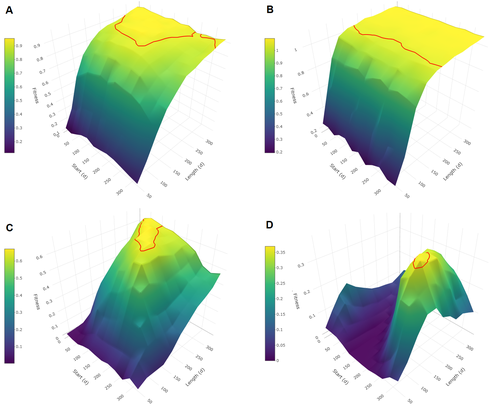
Evolutionary determinants of reproductive seasonality: a theoretical approach
Abstract
Recommendation: posted 24 May 2023, validated 24 May 2023
Coulson, T. (2023) When does seasonal reproduction evolve?. Peer Community in Ecology, 100470. https://doi.org/10.24072/pci.ecology.100470
Recommendation
Have you ever wondered why some species breed seasonally while others do not? You might think it is all down to lattitude and the harshness of winters but it turns out it is quite a bit more complicated than that. A consequence of this is that climate change may result in the evolution of the degree of seasonal reproduction, with some species perhaps becoming less seasonal and others more so even in the same habitat.
Burtschell et al. (2023) investigated how various factors influence seasonal breeding by building an individual-based model of a baboon population from which they calculated the degree of seasonality for the fittest reproductive strategy. They then altered key aspects of their model to examine how these changes impacted the degree of seasonality in the reproductive strategy. What they found is fascinating.
The degree of seasonality in reproductive strategy is expected to increase with increased seasonality in the environment, decreased food availability, increased energy expenditure, and how predictable resource availability is. Interestingly, neither female cycle length nor extrinsic infant mortality influenced the degree of seasonality in reproduction.
What this means in reality for seasonal species is more challenging to understand. Some environments appear to be becoming more seasonal yet less predictable, and some species appear to be altering their daily energy budgets in response to changing climate in quite complex ways. As with pretty much everything in biology, Burtschell et al.'s work reveals much nuance and complexity, and that predicting how species might alter their reproductive timing is fraught with challenges.
The paper is very well written. With a simpler model it may have proven possible to achieve analytical solutions, but this is a very minor gripe. The reviewers were positive about the paper, and I have little doubt it will be well-cited.
REFERENCES
Burtschell L, Dezeure J, Huchard E, Godelle B (2023) Evolutionary determinants of reproductive seasonality: a theoretical approach. bioRxiv, 2022.08.22.504761, ver. 2 peer-reviewed and recommended by Peer Community in Ecology. https://doi.org/10.1101/2022.08.22.504761
The recommender in charge of the evaluation of the article and the reviewers declared that they have no conflict of interest (as defined in the code of conduct of PCI) with the authors or with the content of the article. The authors declared that they comply with the PCI rule of having no financial conflicts of interest in relation to the content of the article.
This work was supported by the ANR grant ANR ERS-17-CE02-0008, 2018-2021 awarded to Elise Huchard. Lugdiwine Burtschell thanks AgroParisTech for the financial support.
Reviewed by Nigel Yoccoz
, 22 May 2023
The authors have answered all my previous comments, and I find the current version of the manuscript an interesting contribution to our understanding of reproductive seasonality. I have only a few minor comments.
l. 226: statistic optimisation model: you mean stochastic?
l. 228: density-dependent
l. 243: "5% as a confidence margin". Not sure what you mean here. Do you mean that 5% is used because your estimates of fitness for the different tactics are uncertain (2000 simulations only etc), or because you consider a 5% change to be small enough that tactics can be considered to be "evolutionary similar"?
l. 317: punctually - consider another word, momentarily?
Figure 4 (and 5, 6): not sure it is very informative to give mean and sd when distributions are very skewed (unpredictability = 0). Median (already shown) and quartiles would be more relevant when comparing levels of unpredictability.
l. 500: high environmental (H1). something is missing here, seasonality.
l. 504, 508: would make more sense to have Heldstab 2021a, 2021b rather than b then a...
You have now added a sentence regarding the importance of measuring resources and not use a proxy like NDVI - if you consider for example ungulates in temperate environments, quality of food is as or more important than quantity of food, particularly for juvenile individuals (they cannot grow/survive on low quality food). NDVI would be a quite poor proxy for food quality, except if you use NDVI to estimate when food starts to grow and is therefore profitable ("greening", not "green")
https://doi.org/10.24072/pci.ecology.100470.rev21Evaluation round #1
DOI or URL of the preprint: https://doi.org/10.1101/2022.08.22.504761
Version of the preprint: 1
Author's Reply, 18 Apr 2023
Decision by Tim Coulson
, posted 01 Oct 2022
Three reviewers have now provided thoughtful and detailed comments on the preprint. All are broadly supportive of the simulations, and the way they are written up and reported. I consequently expect that I will ultimately recommend the preprint as it makes a valuable contribution to our underastanding of reproductive decision-making in variable and seasonal environments. Despite these positives, there is work to do. All three reviewers make some major comments along with more minor suggestions. All the comments can be dealt with, some via rewriting or referring to additional literature. Other comments will require revisiting the simulations or the way results are reported. In particular, all three reviewers note that using p-values to assess statistical significance from simulations is problematic, simply because you can increase the number of simulation runs until statistical significance of the output of interest is achieved. Something that explains a very tiny amount of variation then has a statistically significant p-value but is biologically unimportant. You really need to describe the difference in means and variance without recourse to p-values. You also need to better justify some assumptions, refer to a wider literature, and add clarity to some of the methods. Reviewer 3 also makes a good point -- you need to justify why you are averaging over stochastic simulations. Doesn't this simply reveal the deterministic skeleton of your model? All these alterations are achievable, and i very much look forward to seeing a revised version in due course.
Reviewed by Nigel Yoccoz
, 18 Sep 2022
I concur with the authors that our understanding of reproductive seasonality is relatively fragmentary, and that theoretical approaches are needed to complement empirical studies. The authors propose here an individual-based model derived from field studies on the yellow baboon, with energetics and resource availability being the main drivers of change in the model. The model framework is a first step that can lead to further developments.
My comments address mostly how the paper relates to the current literature and some of the underlying model assumptions. I have also to admit a “small mammal” bias.
L. 69: “and unpredictability (year-to-year variation in food availability) “ : this is not the same thing - you may have large year-to-year variation which is at least in part predictable. For example, with el Nino-la Nina, or cyclic populations (small rodents, forest pest species). It is also different in terms of temporal scale: in this paper, the measure of variability combines both short-term (between weeks, as you use NDVI values that are given with a 2 weeks interval) and longer-term (between year) variability. Environmental change may affect both components in different ways. I understand that one cannot do everything in one paper, but it helps to make the assumptions clear – you partly discuss this l. 445-446 by referring to papers by Colwell and English, but without giving any clear idea of what it can mean in practice.
L. 79: “However, only a few studies have investigated the effect of environmental unpredictability on reproductive seasonality”. Not sure what you mean here – there is for example a large literature on trophic mismatch and environmental variability, as well as the importance of timing for population dynamics (eg Li et al. 2021).
l. 103: note that small multivoltine mammals are also "far from an integer number" (ie much less than 1), but that will have very different consequences as they have multiple generations per year, and different generations may have different decisions to make (when to start reproduction vs when to stop).
l. 127: not sure this is correct even if I do not have any number to back it up. There are for example many studies on reproductive synchrony in large herbivores, and the costs and benefits of being born too early or too late. But the bias towards temperate species is clear, but not specific to reproductive seasonality.
l. 142: you use NDVI as a proxy for resource availability but that is a rather strong assumption and you do not really provide empirical support by correlating NDVI to actual resources used by baboons (it seems to have some predictive ability but it is not clear exactly how). In different papers, you write that it is related to primary productivity (this paper l. 143), greenness and plant biomass (l. 188-189 this paper), vegetation cover (Dezeure et al. 2021 Breeding seasonality generates reproductive trade-offs, l 282). These different aspects of vegetation are of course somewhat correlated, but it would help developing better proxies of resources and be more explicit about what is relevant. You would also need to check the values you have since in the papers you refer to (Dezeure et al. 2021 PRSB; eg Figure 1 ), you give values of NDVI around 0.10-0.15, whereas NDVI values so low would rather fit the environments I am more familiar with (ie high Arctic). Values of NDVI are ususall standardized and one would expect values around 0.5-0.8 in the study area.
L. 234: how do you decide on what is small vs large differences? You appear later on to use statistical tests but this is well known to be a very crude way to assess such differences. By increasing the number of simulations (eg 2 millions rather than 2000) I guess all differences would be statistically significant. Of course the P-values and the associated t-statistic you use is a function of the mean simulation difference and the simulation variance, so the question is what it brings in addition to just investigate the mean differences and possibly the variances if they differ a lot (which could be of interest)?
L. 256: So in your model the component of adult survival (here approximated by lifespan) which is independent of energetics/resources is independent of environmental variation? But in the data, lifespan is partly determined by environmental variation, right? Just to be sure I understand the assumption here. One should not need to dig in the supplementary material to be sure.
l. 324: as if the residual variation would represent "only" the biological variation... there is alarge measurement error, 2 weeks variation is different from year to year variation... Just make this explicit.
l. 331: this would clearly be "wrong" in terms of possible values for NDVI (i.e. NDVI=Normalized DVI is normalized between 0 and 1) - why not just remove completely NDVI and just use a theoretical measure of resources, as in Sun et al. 2020. I think the use of NDVI is more a distraction rather than anything really useful here.
Detailed comments:
L. 57: This seems a bit trivial to me - hard to imagine caribous breeding in the middle of the winter in Alaska... It would perhaps be more relevant to point out that some Arctic species may breed more or less year round (eg lemming under the snow) but others (caribou) are highly seasonal, and refer to tropical species that either breed non-seasonally or seasonally (for example the Serengeti species you mention).
L. 63: even Arctic species can breed year-round, eg lemmings.
L. 89: We do not refer to delayed implantation in Langvatn et al. 2004 but to delayed ovulation at high densities.
l. 90: Hellgren 1998 is not in reference list - is this Hellgren, E. C. 1998. Physiology of Hibernation in Bears. Ursus 10:467-477? This paper is more about physiology than ecology, perhaps use a more ecological/demographic paper. There is an abundant literature on bears of course but also marmots, etc.
l. 359, figure 2: you cannot have negative values for r, perhaps find better regression lines, or cut them at 0. What is the grey colour on the figure?
Figure 3: Please remove NS and stars from the figure, provide quantitative differences with some measure of uncertainties.
l. 500-1: “intensity of reproductive seasonality was not associated with gestation length but rather with the size and number of litters per year (Heldstab, 2021)”. For rodents it was the number of litters per year and it is a bit tautological – if one has many litters per year, it is difficult to image being highly seasonal?
Nigel G. Yoccoz
References
Li, G., X. Wan, B. Yin, W. Wei, X. Hou, X. Zhang, E. Batsuren, J. Zhao, S. Huang, X. Xu, J. Liu, Y. Song, A. Ozgul, C. R. Dickman, G. Wang, C. J. Krebs, and Z. Zhang. 2021. Timing outweighs magnitude of rainfall in shaping population dynamics of a small mammal species in steppe grassland. Proceedings of the National Academy of Sciences 118:e2023691118.
https://doi.org/10.24072/pci.ecology.100470.rev11
Reviewed by Francois-Xavier Dechaume-Moncharmont
, 27 Sep 2022
This article is a simulation-based investigation of the drivers of seasonal reproduction in the yellow Baboon. It aims at disentangling the complex effects of several ecological constraints and life-history traits. Most notably, it does a nice job in separating environmental productivity and seasonality which are inextricably correlated in studies investigating the effect of latitude: higher latitudes are both marked by high seasonality and low productivity, and this correlation is confusing when one want to understand the drivers of phenology. As a non-native English-speaking writer, I won't venture to criticize English or the syntax, which seem to be fine as far as I can tell. The MS is clearly written, with great deal of details, even if some important and non-trivial parts are to be found as supplementary materials. I also appreciated the fact that the source codes are developed in open-source languages and available on public depository. It certainly helps the reader to understand the model. The model is built on the Baboon biology, which is both a strength (the parameters are calibrated on field data) and a weakness (extrapolation of the results in non-ape species may be not straightforward). My overall impression about this MS is largely positive. Yet, several points need clarification or additional explanations.
Major comments
The introduction section should be partly rewritten. It should be more explicit about the differences between this study and previous literature. For instance, lines 79-81, one can read allusive statements: “However, only a few studies have investigated the effect of environmental unpredictability on reproductive seasonality (Dezeure et al., in press; English et al., 2012), with mixed results so far.” First, the reference in press is submitted by co-authors of the present study, but the reader cannot appreciate the content of the cited. Second, what do the authors mean by these mixed results? I recommend the authors to be more explicit about the novelty of their paper compared with existing literature. The introduction is maybe too long at some points. For instance, the authors may reconsider the comments about capital VS. income breeders (lines 82 and following paragraph) as it is weakly relevant in the understanding of the analyses conducted in the model.
The way of finding optimal strategy among simulated phenologies for a given set of simulation parameters should be detailed and illustrated at some point of the manuscript. I recommend to plot (possibly in supplementary material) the reproductive outcomes as function the phenologies for several sets of simulation parameters. Such plots allow for understanding the strength of selection pressure around the optimal phenology value. Even if a given value corresponds to the optimal phenology, the selection pressure towards this value could be very weak if the curve is rather flat around the optimal value. Mathematically the selection pressure corresponds to second-order derivative around the optimal value. Ideally, I would be very interested in finding quantitative information about theses selection pressure as a function of the environmental conditions. I expect that some set of condition leading to intermediate reproductive phenology are in fact under very weak selection pressure: following suboptimal phenology are poorly counter selected. This information is very important in the context of uncertain environments. It makes no sense to evolve highly efficient phenology leading to peaky optimal response curves because any uncertainty of stochasticity in the actual set of environmental condition would result in very suboptimal fitness gain. Finally, an additional interest of such plots is to assess the possible existence of local maxima leading to sympatric polymorphisms, or difficulty evolve from one phenology to another in absence of high-range mutation jumps.
Still on this question of finding the optimal strategy, I disagree of the method based on significant difference in simulation outcome. For instance, even the smallest differences become highly significant when the sample increase. It is only a question patience and brute force. The t-test were based on 2000 simulations. Fair enough, you just need to increase the number of simulations to finally obtain significant p-value (it is one major objection against the use of p-value calculation in science). Please consider metrics insensitive to sample size, for instance 5% decrease of effect size index or similar arbitrary criterion. The use of **statistically significant** difference is misleading and questionable in simulation context.
The way of modelling the reproductive cycle by artificially increase the number of days per year (lines 337 and followings) is rather hard to understand, and the results are difficult to interpret. I did my best to understand this modelling choice, but I am still puzzled by this analysis. I am not saying that the method is incorrect, I am just saying that, if conserved in the latter version of the MS, this non-trivial modelling choice deserves much clearer presentation.
Caveats. I recommend that the authors acknowledge and discuss several caveats about the present model. In its present form, it is a statistic optimisation model. The strategy of the female is fixed throughout her lifetime. Her investment does no vary dynamically as a function of her own reserve or age. For instance, the model does not allow for terminal investment or increase in reproduction investment toward the end of the life. Such analysis would require dynamic state modelling (dynamic programming). Similarly, the model optimizes the strategy of an isolated female in absence of any competitor. Yet, it is possible that stringent environmental conditions lead to polymorphism of strategies, some females choosing to delay or bring forward their reproduction to avoid competition for limited resources. Here, game theoretic models would be relevant to cope for frequency dependent effect. I fully understand that such developments have been neglected in the present study for sake of simplicity. Yet, these caveats should be pointed out in the discussion section.
Minor comments
Line 74: awkward wording choice “(…) disentangling environmental productivity, seasonality and their interaction”. At least the analysis could disentangle **the effect of** environmental constraints, but the environmental parameters themselves.
Line 83. Merge the two citations
Lines 87-93. This sentence is way to long and confuse. Even after several attempts, I still do not fully understand its point.
Line 101. While I understand what the authors mean by “close to an integer number of years”, it is unnecessary obscure way of presenting the asynchrony of reproduction schedule across year. I recommend that the authors take greater care in presenting their analysis is a clearer way. Theoretical models are sufficiently unappealing for most ecologists (Fawcett TW, Higginson AD. 20212. Heavy use of equations impedes communication among biologists. Proceedings of the National Academy of Sciences 109:11735–11739 http://dx.doi.org/10.1073/pnas.1205259109) for the authors to indulge in needlessly obfuscating formulation.
Line 102. “far from” is too vague a term. Be more specific.
Line 124-125. “This species was chosen here because it belongs to a genus characterised by phenological flexibility with one in six Papio species breeding seasonally”. This a rather weak justification for model species choice.
p.329. I was strongly surprised by this modelling choice for the very reasons finally exposed toward the end of the MS (discussion section line 459 and followings). I recommend that the authors are more explicit of this equation and its limitation in this section. Similarly, I have the greatest difficulty to appreciate the relevance of the arbitrary range of variation for $p$, $s$ and $u$ parameters listed lines 330-332. Please, elaborate on this point.
Line 334. Be more explicit: “daily **reproductive** energy expenditure”.
Line 341. What is MODIS data? Explain and give appropriate references.
Line 353. Fig. 2c instead of Fig. 2b.
Fig 2b is not called in the main text. In addition, I do understand its relevance here.
Fig. 2, 3 and 4. I do not understand the reason and meaning of the grey cells. Please explain them in the legend and the main text. In addition, the default parameter values for the simulation should be stated in the legend.
Line 425. New results, and particularly highly important ones, should not appear for the first time in the discussion section. This paragraph should be moved in the result section.
What is $\tho$ in supplementary p.28?
https://doi.org/10.24072/pci.ecology.100470.rev12Reviewed by anonymous reviewer 1, 30 Sep 2022
The authors describe a model that explores the environmental conditions under which reproductive seasonality is favoured. I think it's an interesting piece of work and the results seem plausible. I do, however, have some queries about the methods:
Major comments:
I’m not sure I understand the necessity of statistical tests for comparing model outputs. Isn’t it possible to just run more simulations and decrease uncertainty in the mean of the output to any desired degree of accuracy? Relatedly, have you tested the stability of your mean/variability estimates given different numbers of simulation runs (i.e. why 2000 runs)? There's a bit of literature on these issues see appendix B of (1) - it might be preferable to have an effect size rather than a probability based measure of significance.
I might be missing a step here, but why include stochasticity in mortality/reproduction at all? You ultimately seem to use multiple simulations to converge to the non-stochastic answer anyway (although not completely e.g. Fig 2a). Is it to see if stochasticity would overcome small differences derived from optimal breeding time?
L. 334 To test an increase in daily expenditure you increase the growth rate which subsequently impacts the gestation period. Why not just increase expenditure through the constant of energy expenditure (equation 6 appendix)? I also find the changes to the length of the year slightly odd. I think it's probably OK, but seems like a strange approach – isn’t it possible to impose a non-breeding period that shifts the cycle through the year - or perhaps this would cause other issues?
Minor comments:
L.47 ‘allows synchronizing the energetic costs...’
L.306 Slightly puzzled by this paragraph, is the ABM 3d or is it 3 dimensions of parameter space? You mention ‘3d rep is difficult to apprehend and increasing computing time’ does this mean not all levels of unpredictability were simulated? I might consider rewriting parts of this paragraph for clarity around what is in the model and what is being presented as a result (e.g. heatmaps)
L.318 the equation as written and described implies that the NDVI would be timeseries mean + daily mean which would give too much NDVI, whereas I think you calculate a daily offset from the mean (i.e. positive or negative)
L.351 I’m not sure if this sentence is correct, you see high birth seasonality with greater than the observed productivity (p=1.1 to 1.3)
L.411 and other figures: you don’t typically need to say you ‘plotted’ you can just say ‘Panel A shows ... ‘
(1)Lee, J.S., Filatova, T., Ligmann-Zielinska, A., Hassani-Mahmooei, B., Stonedahl, F., Lorscheid, I., Voinov, A., Polhill, J.G., Sun, Z. and Parker, D.C., 2015. The complexities of agent-based modeling output analysis. Journal of Artificial Societies and Social Simulation, 18(4).