
Two paradigms for intraspecific variability
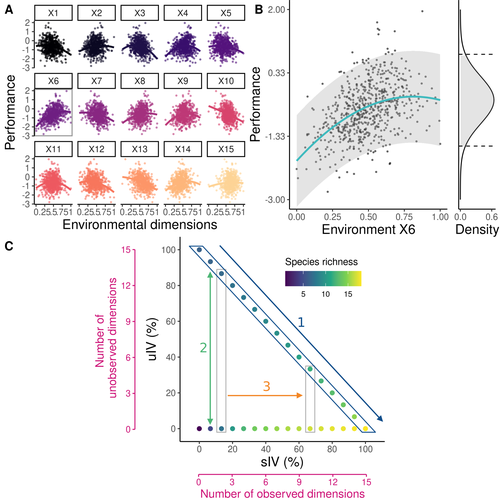
Beyond variance: simple random distributions are not a good proxy for intraspecific variability in systems with environmental structure
Abstract
Recommendation: posted 08 January 2024, validated 10 January 2024
Barbier, M. (2024) Two paradigms for intraspecific variability. Peer Community in Ecology, 100466. 10.24072/pci.ecology.100466
Recommendation
Community ecology usually concerns itself with understanding the causes and consequences of diversity at a given taxonomic resolution, most classically at the species level. Yet there is no doubt that diversity exists at all scales, and phenotypic variability within a taxon can be comparable to differences between taxa, as observed from bacteria to fish and trees. The question that motivates an active and growing body of work (e.g. Raffard et al 2019) is not so much whether intraspecific variability matters, but what we get wrong by ignoring it and how to incorporate it into our understanding of communities. There is no established way to think about diversity at multiple nested taxonomic levels, and it is tempting to summarize intraspecific variability simply by measuring species mean and variance in any trait and metric.
In this study, Girard-Tercieux et al (2023a) propose that, to understand its impact on community-level outcomes and in particular on species coexistence, we should carefully distinguish between two ways of thinking about intraspecific variability:
-"unstructured" variation, where every individual's features are like an independent random draw from a species-specific distribution, for instance, due to genetic lottery and developmental accidents
-"structured" variation that is due to each individual encountering a different but enduring microenvironment.
The latter type of variability may still appear complex and random-like when the environment is high-dimensional (i.e. multifaceted, with many different factors contributing to each individual's performance and development). Thus, it is not necessarily "structured" in the sense of being easily understood -- we may need to measure more aspects of the environment than is practical if we want to fully predict these variations.
What distinguishes this "structured" variability is that it is, in a loose sense, inheritable: individuals from the same species that grow in the same microenvironment will have the same performance, in a repeatable fashion. Thus, if each species is best at exploiting at least a fraction of environmental conditions, it is likely to avoid extinction by competition, except in the unlucky case of no propagule reaching any of the favorable sites.
By contrast, drawing each individual's preferences and performance randomly at each generation (from its own species distribution, but independently from other and past individuals) leads to stochastic dynamics, so-called ecological drift, that easily induce a large number of species extinctions.
The core intuition, that the complex spatial structure and high-dimensional nature of the environment plays a key explanatory role in species coexistence, is a running thread through several of the authors' work (e.g. Clark et al 2010), clearly inspired by their focus on tropical forests. This study, by tackling the question of intraspecific determinants of interspecific outcomes, makes a compelling addition to this line of investigation, coming as a theoretical companion to a more data-oriented study (Girard-Tercieux et al 2023b). But I believe it raises a question that is even broader in scope.
This kind of intraspecific variability, due to different individuals growing in different microenvironments, is perhaps most relevant for trees and other sessile organisms, but the distinction made here between "unstructured" and "structured" variability can likely be extended to many other ecological settings.
In my understanding, what matters most in "structured" variability is not so much it stemming from a fixed environment, but rather it being maintained across generations, rather than possibly lost by drift. This difference between variability in the form of "frozen" randomness and in the form of stochastic drift over time is highly relevant in other theoretical fields (e.g. in physics, where it is the difference between a disordered solid and a liquid), and thus, I expect that it is a meaningful distinction to make throughout community ecology.
References
James S. Clark, David Bell, Chengjin Chu, Benoit Courbaud, Michael Dietze, Michelle Hersh, Janneke HilleRisLambers et al. (2010) "High‐dimensional coexistence based on individual variation: a synthesis of evidence." Ecological Monographs 80, no. 4 : 569-608. https://doi.org/10.1890/09-1541.1
Camille Girard-Tercieux, Ghislain Vieilledent, Adam Clark, James S. Clark, Benoît Courbaud, Claire Fortunel, Georges Kunstler, Raphaël Pélissier, Nadja Rüger, Isabelle Maréchaux (2023a) "Beyond variance: simple random distributions are not a good proxy for intraspecific variability in systems with environmental structure." bioRxiv, ver. 4 peer-reviewed and recommended by Peer Community in Ecology. https://doi.org/10.1101/2022.08.06.503032
Camille Girard‐Tercieux, Isabelle Maréchaux, Adam T. Clark, James S. Clark, Benoît Courbaud, Claire Fortunel, Joannès Guillemot et al. (2023b) "Rethinking the nature of intraspecific variability and its consequences on species coexistence." Ecology and Evolution 13, no. 3 : e9860. https://doi.org/10.1002/ece3.9860
Allan Raffard, Frédéric Santoul, Julien Cucherousset, and Simon Blanchet. (2019) "The community and ecosystem consequences of intraspecific diversity: A meta‐analysis." Biological Reviews 94, no. 2: 648-661. https://doi.org/10.1111/brv.12472
The recommender in charge of the evaluation of the article and the reviewers declared that they have no conflict of interest (as defined in the code of conduct of PCI) with the authors or with the content of the article. The authors declared that they comply with the PCI rule of having no financial conflicts of interest in relation to the content of the article.
Evaluation round #2
DOI or URL of the preprint: https://doi.org/10.1101/2022.08.06.503032
Version of the preprint: 2
Author's Reply, 10 Dec 2023
Decision by Matthieu Barbier
, posted 12 Jul 2023, validated 13 Jul 2023
Dear authors,
Thank you very much for your replies and the significant improvements you made upon the text. I most sincerely apologize for the delayed response, due to health issues.
I believe the article does not need to go through reviewers again; it is not far from a potential final state, which it can reach by a mild process of removal rather than addition (or it can undergo a longer process of gathering sufficient support for the claims that I am currently less convinced by)
- Addressing my main comment 1 below might simply require removing a few instances of mentioning temporal structure.
- Regarding my main comment 2, I would put it thus: while I feel that the central message that "structured IV is different" is interesting and comes across easily enough, the specifics of how it is different and why in your particular simulations are still a bit perplexing, and so I remain hesitant to trust certain particulars in your results and discussion.
Those particulars are probably not very relevant to your main message, so I think that just being a bit more prudent, and avoiding interpretations that are perhaps ecologically sensible but potentially wrong or misleading in the context of your simulations, would be enough to satisfy me.
Sincerely,
Matthieu Barbier
MAIN COMMENTS
1) There is one point I failed to explain properly in my previous comments: uIV, as you conceptualize it, should be effectively identical to sIV with many environmental factors changing very rapidly, so that each new individual that is born encounters a new environment unrelated to those that existed before.
Therefore, it cannot always be true that your claims regarding sIV hold even when the environment can change in time, or can be transposed from spatial structure to temporal structure without further investigation. Constancy in time is likely an important ingredient for your results.
Indeed, implementing simulations with zero uIV but a temporally changing environment (+ abundance-dependent fecundity) was the most robust way I got diversity drops as large as yours in my attempt to replicate your results, see below.
2) I attempted to reproduce the simulations (see Python code below, following most of your methods except for the autocorrelation in environmental factors which should be of no consequence) and encountered at least two issues which might indicate that your simulations are perhaps not doing exactly what you meant for them to do:
2.1) least importantly: in the case of uIV, modelling \hat epsilon as an unbounded random variable (e.g. a normally-distributed one) doesn't just create IV, it also creates a trend towards higher and higher performances far beyond anything in the reference model, since we systematically kill individuals with lower values and draw new individuals that only remain in the long term if their performance is at least equal to and typically higher than other existing individuals'.
Over time, regardless of the fraction of sIV, the performances of surviving individuals are thus less and less related to the environment, and stem more and more from random numbers (hat epsilon) becoming improbably rare and high. In my simulations I was able to observe an increase toward massive values of performance (e.g. a mean of 5, starting from a max of 1).
The more extreme aspects of this issue can be solved, of course, by bounding the random variable (e.g. using a uniform distribution between -1 and 1) -- then the passage of time will typically just lead to all surviving individuals being as close as possible to the ceiling imposed by the random variable.
2.2) most importantly: I still don't understand exactly why you get extinctions (and especially as many as you do), and I don't know how much of it is *truly* about uIV.
Your Perfect Knowledge model allows many species to coexist even if there is a single environmental dimension -- it is enough that they have different optima and thus that each wins in different sites. Regardless of IV, there is no *deterministic* reason (such as "not enough niche partitioning") for any species going extinct in your model, especially not due to decreasing the number of modelled environmental factors.
This leads to the suspicion that a lot of what you see in IK models is due to having the wrong functional form in Eq 2, ending up with an artefactual site-independent competitive hierarchy because different species get different intercepts beta_0,j and this becomes really impactful when n_obs is small compared to K (otherwise, why would adding uIV ever increase diversity?)
In my simulations, simply replacing sIV by uIV but without the model misspecification, I was unable to reproduce such large diversity drops for anything like the parameters mentioned in the main text and SI -- in particular, when I tried Fixed Fecundity, no species ever died out over such a large landscape with so little mortality and so many propagules. With 1% mortality, at most 6 individuals die each time step, while 200 propagules are released, so stochastic effects are pretty weak. In your setup I would expect every species to be best in ~30 sites out of 600, thus dying out with fixed fecundity and low uIV would basically require a species being absent from all these 30 sites at the same time while better species were present in all 10 other sites where it is sending its propagules.
For me, abundance-dependent fecundity was necessary for species to go extinct at all, and it had to be combined with totally random mortality to reach low species numbers (e.g. 5). Thus it's not impossible to get these numbers, but it requires having almost gone to a neutral model, and then the fraction of uIV vs sIV doesn't matter much.
Thus, while I am willing to agree with your general message, the quantitative details are perplexing and suggest a larger role of model misspecification than of sIV versus uIV (the importance of misspecification is potentially a valid point to make, but not one that really serves your message!), so I don't know exactly what is robust among the detailed analyses you provide in results and discussion.
I think it is fine to present these results as observations (= you decided on a certain simulation plan, it gives such and such results, that's a fact) but stay cautious about interpretations (e.g. in terms of niche partitioning and how it interacts with IV) and general statements.
3) Finally, a very minor comment that sprang from Simon Blanchet's mention of drift for small populations: it is clear that you are setting aisde genetic drift (or genetic anything) here, but it is worth mentioning the notion of *ecological* drift i.e. small populations going extinct due to chance events, which is probably the main cause of exinctions here except model misspecification. Such drift is initially more likely when you start with one individual per species (you will automatically lose one out of 20 species at the first time step if your mortality is larger than 5% for instance), but it is not clear that these transient effects are of much import here, I would guess not in most of your simulations.
WRITING COMMENTS
This is quite optional, more comfort than necessity, but parts of the discussion still feel like they might fare better in the (very short) Results section instead. The paragraphs starting with "Compared to the reference communities simulated ", "When structured IV accounted for less", "When the proportion of structured IV increased, ", "Our results suggest that improving" ... tended to break the flow of the broader discussion, by mostly describing results and only briefly including a few additional thoughts and interpretations (some of which I am not necessarily convinced, see above)
Eq1: d_i,j,m instead of d_m,j
The sentence "Using the Imperfect knowledge models with nobs = K, we were able to test if the error due to this assumption was large" seems out of place, since I assume the assumption it refers to is the incorrect quadratic model Eq2; I would move it and/or clarify that the shape of Eq2 is the assumption in question.
It feels a bit confusing to have Eq3 and Eq4 one after the other with the same notations and without any text to separate them and explain what is what; I would put Eq3 after the first sentence of the preceding paragraph and Eq4 after the second (and ending the seocnd with something like "as modelled by a randomly drawn contribution to performance \hat epsilon_i,j,m").
It is a bit confusing that Eq4 is exactly like Eq2 with a hat, when in one case epsilon is an estimated residual, and in the other, epsilon hat is a random variable drawn each time an individual is born in a simulation.
My recommendation would be to leave \hat epsilon_i,j,m ~ N(0,V_j) in eq 4, as this equation truly indicates a random variable drawn from a distribution, while Eq 2 could instead have something like V_j = var(epsilon_i,j,m) to explain that it is an "empirical" quantity estimated from the residuals.
==========================================================================================================
import numpy as np, pandas as pd
mortality=['deterministic','random'][0]
fecundity=['fixed','dependent'][1]
deltaE=0. # How much the environment changes in each patch per turn
tmax=10000
J=20 #nb species
K=15 #nb env dim
M=625 #nb sites
uIV=0.9 #Fraction uIV
nprop=10 #nb propagules (initial, and each turn for fixed fecundity)
fecund = 0.5 # nb of propagules per capita (for abundance-dependent fecundity)
death=0.01 #Fraction of dead individuals per turn
E=np.random.uniform(0,1,(K,M)) #environment values
Eopt=np.random.uniform(0,1,(J,K)) #species environmental preferences
alld = np.sum( (Eopt.reshape((J,K,1)) - E.reshape((1,K,M)) )**2, axis=1 )**.5
mud,sigd=np.mean(alld),np.std(alld)
#Which species occupies each site
Occ=np.zeros(M,dtype='int')-1
#Initial condition
initial_pos=np.random.choice(np.where(Occ<0)[0],size=J*nprop,replace=True)
for j in range(J):
Occ[initial_pos[j*nprop:(j+1)*nprop]]=j
#Computing performance for a new individual
def calc_perf(E,Eopt,unstructured):
d=np.sum( (E-Eopt.T )**2, axis=0 )**.5
return - (d-mud)/sigd *(1-unstructured) + np.random.uniform(-1,1,d.shape)* unstructured
#Performance at each site
Perf=calc_perf(E, Eopt[ Occ ],uIV)
Perf[Occ<0]=np.nan
table=[]
t=0
while t < tmax:
print(t)
## For temprally changing environments, compute individuals' changes in performance
if deltaE>0:
prev= calc_perf(E, Eopt[ Occ ],0)
E=np.clip(E+np.random.uniform(-deltaE,deltaE,E.shape), 0,1)
next=calc_perf(E, Eopt[ Occ ],0)
Perf=Perf+(next-prev)
#Death
occupied=np.where(Occ>=0)[0].astype('int')
if mortality=='deterministic':
#The worst x% die
worst = occupied[np.argsort(Perf[occupied])[:int(M*death)]]
elif mortality =='random':
#x% die at random (just a test, not in the paper)
worst=np.random.choice(occupied,size=int(M*death))
Occ[worst]=-1
Perf[worst]=np.nan
#Fecundity
if fecundity=='fixed':
# Fixed fecundity
propagule_sp=np.array([ j for j in range(J) for prop in range(nprop) if (Occ==j).any() ])
elif fecundity == 'dependent':
# Abundance-dependent fecundity
propagule_sp=np.array([ j for j in range(J) for prop in range( int(np.sum(Occ==j)*fecund ) ) ])
if len(propagule_sp)==0:
break
propagule_pos=np.random.choice(np.where(Occ<0)[0],size=len(propagule_sp),replace=True)
propagule_perf = calc_perf(E[:,propagule_pos],Eopt[propagule_sp],uIV)
for pos in np.unique(propagule_pos):
candidates=np.where(propagule_pos==pos)[0]
best=candidates[np.argmax(propagule_perf[candidates])]
Occ[pos]=propagule_sp[best]
Perf[pos]=propagule_perf[best]
# Summary outcomes
x=np.array([np.sum(Occ==j) for j in range(J) ])
p=x/np.sum(x)
dic={'diversity':np.sum(x>0),'shannon':np.exp(-np.sum( p[p>0]*np.log(p[p>0]))) , 'occupation':np.mean(Occ>=0),'mean_performance':np.nanmean(Perf)}
table.append(dic)
t+=1
results=pd.DataFrame(table)
########################
# RESULTS
import matplotlib.pyplot as plt
plt.figure()
plt.subplot(221),plt.title('Occupation')
plt.plot(results['occupation'])
plt.subplot(222),plt.title('Diversity')
plt.plot(results['diversity'],label='number')
plt.plot(results['shannon'],label='shannon')
plt.legend()
plt.subplot(223),plt.title('Performance')
plt.plot(results['mean_performance'])
plt.subplot(224),plt.title('Final landscape occupation')
x=Occ.copy().astype('float')
x[x<0]=np.nan
plt.colorbar(plt.imshow(x.reshape((25,25)) ,cmap='jet'))
plt.show()
Evaluation round #1
DOI or URL of the preprint: https://doi.org/10.1101/2022.08.06.503032
Author's Reply, 26 May 2023
Decision by Matthieu Barbier
, posted 10 Oct 2022
Dear Authors,
Thank you for sharing this manuscript with us.
Based on the two reviews and my own read through, I believe this study asks a very interesting question, and I want to recommend it as a proof of concept that this question can matter. I also believe, like the reviewers, that the manuscript should be more precise about what it demonstrates exactly, and I would therefore request some effort at clarification.
I think all the issues that we collectively raise can be addressed with a moderate amount of work, essentially changes in writing, but that this work is important for the manuscript.
I would not consider it to require major revisions, but I ask that you seriously consider the suggested changes, and do not hesitate to contact us for further discussion if you disagree or have questions.
I would boil down the issues to two main aspects (but please also address reviewer comments that are not necessarily emphasized here)
1) one that is more a question of presentation (which would mainly require changes in the abstract, intro and bits of discussion),
2) another that is about better understanding the results themselves (and in particular which structure matters in structured IV).
------
1) Presentation and scope:
The study makes some restrictive assumptions, at different levels, that may be intuitive for forest dynamics but may not be very representative of other systems.
As a proof of concept, I think it deserves to be out there and it certainly does not have to account for every notion of coexistence or IV in the literature; but it will certainly get a warmer reception if it explicits its choices (see reviewer comments).
Here is a suggested set of clarifications:
- A certain type of structure: since there are no interactions between neighbors nor limitations on dispersal range, spatial structure -- in the classical sense of who is next to whom, and spatial autocorrelation in the environment -- is irrelevant here.
You could have unequal distributions of environments without any autocorrelation and get the same results.
This is a perfectly acceptable starting point, but a very particular choice when talking about coexistence in space and about structured IV. It rules out many potential structural features and coexistence mechanisms.
- A certain type of coexistence: since there are no density-dependent interactions nor any role of spatial structure, coexistence rests entirely on a) environment-driven variation in local competitive hierarchy, and b) a little bit of chance (see point 2 below). Again this automatically rules out many coexistence mechanisms (including others that are *also* known or interpreted as niche partitioning, e.g. stabilizing/density-dependent effects, even though they act in entirely different ways).
- A certain type of IV: I am interested in the idea that the only driver of individual performance could be a fine-grained high-dimensional external environment, but it is a very strong assumption, practically as strong as e.g. Hubbell's premises in neutral theory. Thus, just like that other work, I think a clear framing of "let us explore this extreme idea and see how far it goes" would be an easier sell.
A more specific comment on that last point: different readers will have different opinions on whether we don't know many important abiotic factors (depending on the system), but we could imagine a high-dimensional "environment" also due to biotic factors (e.g. local presence of pathogens). However, that environment would then not only be high-dimensional, but changing in time, and performance would be more context dependent. If my reasoning in point 2) below is correct, then you are not testing a general effect of structure in IV so much as the effect of local performance being constant in time (once determined on the basis of species identity and unchanging external factors), which would rule out many other aspects of a microenvironment.
2) Clarification of the results:
To me, the results' interpretation suffers from an ambiguity between three contributions:
a) possible differences between the Perfect Knowledge Model (eq 1) and even the best fit using eq 2. Having used different equations there seems like a counter-productive and risky decision, since you might be conflating effects of structure with effects of model mis-specification.
However, I don't think this choice invalidates your results (n_obs=15 gives different results from perfect knowledge, but not dramatically different), so I am fine with letting that go (rather than ask you redo your simulations entirely with the n_obs=15 eq2 as the ground truth!)
b) the specific deterministic structure in IV
c) "frozenness" in time, i.e. the fact that in models without uIV, all future individuals of one species that may come to occupy a given site will have the same performance.
My current intuition is that this third ingredient, rather than anything else, is crucial to explain your results.
I now try to explain this intuition:
First, notice that without any dynamical simulation, you could make predictions about diversity in a purely deterministic case: you draw a performance value for each species in each site, and the fittest species lives there forever. Lack of coexistence would only happen due to some unlucky species being the fittest nowhere, which becomes a simple question of probabilities.
You could already have asked at that level whether the way you create a deterministic structure (distance to some niche center) guarantees more or less coexistence than drawing each species' fitness at random for every site. The case of totally random performance draws is easy: given S=20 species, each of which has probability 1/S to be the best in a given site, and M=625 sites, then the probability of a species going extinct would be
(1-1/S)^M ~ 10^-14
You can of course make things more unequal between species, so that some have a lower probability of being best anywhere, but then the key is the level of inequality, not any particular assumption about structure, and it could only reduce coexistence. Note also that this totally random situation should be at least roughly equivalent to having infinitely-many environmental dimensions in a perfectly deterministic model.
Unless I am missing something, any result of your model that goes against this basic result must therefore have to do with three ingredients:
- any bias introduced by mortality and fecundity mechanisms (the only one I could see really matter is the rich-get-richer effect of abundance-based fecundity)
- the random distribution of propagules among empty sites, which also impacts the Perfect Knowledge model
- temporal fluctuations in the model with uIV, since each individual is now another chance for a species to perform well or badly in a given site. I strongly suspect that this is the main source of stochastic extinctions.
These three are very "neutral-like" ingredients in your model. Since your results are not terribly different in the case of fixed fecundity, we can likely ignore the rich-get-richer part (though I am not surprised that it seems to make things even worse), and random effects are probably the key player here.
In other words, rather than actual structure or not in the IV, what matters might simply be that species performance per site are drawn once and for all in the Perfect Knowledge Model, and changing over time in models with uIV, creating random drift which allows for extinctions.
An easy test could be to run a simulation with independently drawn random performance values for each species x site pair, held constant in time (with some arbitrary level of inequality between species averages if you want). I would expect at least as much coexistence as the Perfect Knowledge model. If this falls within your definiton of structure -- and that could make sense, as I believe a reviewer is pointing -- then this would benefit from being clarified.
-------------
To conclude, I would be careful to state explicitly what you mean by "structured IV" -- you focus on one aspect of IV (performance), its spatial structure is of no import here, and the most central factor in your results might be constancy in time (of how good a site is for all individuals of a given species) rather than being explicitly structured by a certain number of enviromental factors.
Of course, it would be legitimate to interpret this temporal constancy as what it means for IV to be structured by a fixed external microenvironment, rather than by genetics and development, fluctuating abiotic and biotic factors, density-dependent interactions, chance dispersal events, etc. which would all introduce individual or temporal variations.
(I would however hesitate to talk about "spatiotemporal structure" in explaining what is important here)
If you can address or correct the points made by all three of us, and the text is amended to avoid confusion regarding what you are showing precisely, I see no reason not to recommend what I think is an interesting starting point for asking a worthwhile question.
Sincerely,
Matthieu Barbier
======================
MINOR COMMENTS ON WRITING:
ABSTRACT
"Also, comparing communities simulated with the same level of knowledge of the environment, but adding unstructured IV or not, we found that the effects of incorporating unstructured IV depended on the relative importance of structured versus unstructured IV. In particular, increasing the proportion of unstructured IV into the model moved from a positive to a negative effect on community diversity and similarity in composition with the full knowledge model. "
This whole part is a bit long-winded and hard to read, especialy the end of the last sentence.
MATERIALS AND METHODS
l72 "additive inverse": a bit cumbersome
in various places: "thrive" I'd rather say "reside" (thrive implies success)
l90 I was also a bit confused by the use of "triangular" here, though I see what you mean
l141: "Hence" does not really agree with the previous sentence (but rather refers to the step of randomly distributing the propagules), so it is a bit confusing.
DISCUSSION
I also think, like one of the reviewers, that the Discussion is too long and a bit redundant (wihtin itself and with other parts of the manuscript); being more to the point won't be a deciding factor in a recommendation but it would make for an easier read.
Reviewed by Simon Blanchet, 06 Oct 2022
Dear Authors,
Thanks a lot for letting me the opportunity to read this interesting comment. I'm not a theoretician, so I'm unable to judge the technicam (methodological) part of the MS, and I'll therefore provide more conteptual/general comments.
I think this MS is timely and that it tackle an important question related to the way intraspecific variability (IV) is incorporated into theoretical models. I think this is an important questions and that you're MS is providing novel and relevant insights. Nonetheless, I have some critics regarding the way you envisage.
My main critic is that I have the feeling that you consider that fitness traits (performance) of an individual can be entirely capture by environmental parameters, which results that IV is highly determined by the environment, as soon as the enviroment is properly described. This is therefore a deterministic view of IV. You seem to suggest that if IV is not well explained by field ecologists, this is because "most of the environmental variation that actually influences individual's attributes is not properly monitored in ecological studies". This is a strong statement with which -as a field ecologist- I disagree. My personnal opinion is that IV (in most species) is actually driven by both deterministic and neutral processes (as most biodiversity facets actually). For instance, in fish, inter-individual differences in body size (for a same cohort, same parents, in the same environment) in juveniles is indeed partly governed by microhabitat use, but also by prior effects (i.e. fish are not hatching at the exact same time, which determine for instance dominance ranking, etc...). Even within shoal of fish of the same age, inter-individual differences in body size exist whereas they use the exact same micro-habitat. There are plenty of examples like that in the natural world that suggests that not all differences are governed deterministically. Other examples include patterns of "maladaptation"; there has been plenty of studies that recently demonstrated that organisms can actually be maladapted to their local environment (eg because of source-sink dispersal), and I doubt that these patterns emerged only because field biologists failed to properly measure the appropriate parameters. If we would to estimate (across several phyla) the part of IV observed in natural populations that is explained by environmental parameters, we would ne probably around 30-70%. I personnally think that most of the unexplained variance is due to neutral processes (dispersal, drift, random mortality...) that are hard to capture. I'm actually surprised that you did not cite empirical papers having quantified the link between IV and environmental parameters, and that you don't use this flourishing litterature to actually rank your models within this existing data. The only paper you cite to tell that IV is highly structured is one of your paper (Girard-Tercieux et al. 2022); this is fine but I think your arguments should be built on other papers that actually address this issue from empirical data (btw, the example you use in the Introduction about the clones rather suggests that a non-negligible part of IV is not deterministic as inter-clone variation seems high). Based on this comment/critic, I can make several concrete suggestions:
-Tone down the fact that IV is determined only by the environment and that ecologists are not measuring properly the multiple dimensions of the environment. Perhaps the later is true but this is unlikey, as we know that most resources are actually not limiting: the niche of most species can be predicted based on a relatively low number of dimensions. The empirical reality demonstrates that part of IV is determined by the environment, and that another part is determined un-derterministically. Stick on this empirical reality to explore to which extent modelling IV according to this gradient (from purely deterministic to random, or unstructured, as you prefer) affect the outputs of community assembly in theoretical models. And please, avoid the sentences as the one I quote above; in general empriciral ecologists know relatively well their biological models and there are plenty of tools that are now used to estimate the environment very precisely according to several dimensions. This can be improved, but I'm not sure this is the core of the problem (and the goal of your study).
-the terms used to name the models might be changed. As an example, the "perfect knowledge model" is a strange naming. It seems that it is the perfect model that mimick the reality. It is always taken as the "reference". No, this is not a reference, this an extreme model from a gradient going from deterministic to random IV assembly. It represents a situation that probably not exist in the wild (how can we believe that the performance of an individual is purely deterministically determined?, nothing in the wild is purely deterministically determined, otherwise variation would not exist anymore). For instance, you mention in the Discussion that the perfect knowledge model represents the "reality" (l. 276): no, it represents one extreme case along a gradient. You actually don't know what is the reality (and me neither). So I would simply named it "Purely environmentally-driven IV", or "Deterministic model", or any other term more "neutral" than perfect model. The same for the other terms used to name the other two models. Additionnaly, rather than proposing that field ecologists should better monitor the environment (which is already what we are trying to do), I think it is more important to quantify -based on existing data- what is the part of IV that is explained by the environment, and what is the part that is unexplained. I think a meta-analysis could be performed based on existing data, which would be highly valuable for theoreticians to know the extent of IV that should be modeled as structured or not.
Apart from this main comments, I have a few specific ones:
-It was hard to me what you mean by IV. In my mind, IV is the amount of variation observed for one species within a site. It can be quantified using the CV for a trait or the allelic richness for genetic diversity. If I well understood, this is not really what you mean in your models. For instance, under the perfect knowledge model, I would expect that the CV (in term of performance) for a given species at a given site would be extremely low, whereas it would be higher for other models; am I right? If yes it means that what you are manipulating is rather inter-individual variation rather than IV per se. I think you can keep the term IV as there are not clear consensus about definition (but see https://onlinelibrary.wiley.com/doi/full/10.1002/ece3.7884 and https://onlinelibrary.wiley.com/doi/abs/10.1111/brv.12472 for some attempts), but just try to be extremely clear about what you mean, clearly define the terms.
-in the same way, I was expecting you to manipulate traits, not performance (because as a field ecologists we rarely have access to performance!). Again, be extremely clear about that. In the discussion you have a section about niche vs hierarchical traits; perhaps this should be extended into the INtroduction. Or more generally try to introduce how IV is generally modeled in other models (for non theoreticians).
-In figure 1, arrow 1 is not clear; are you comparing the two extremes situation (dots)?
-In equations 2 & 3 the Pîj are not defined. I'm not sure I understood this part. If I well understood, you , first retrieve the parameters of the linear model linking Pij to environmental dimensions. Then you used these parameters to infer Pîj (adding or not a random error to account for uIV); am I right ? Please try to improve a bit this section.
-initialization is done with 10 individuals per species. This is not a lot...As a population geneticist I expect drift to be the major process affecting these populations. Can you tell us if the initial N as an importance for final results?
-l. 132; if mortality is proportional to the performance of an individual, this is not stochastic isn't it? I'm not sure you really modeled a stochastic process of mortality. This is correct but this should be told.
-The Discussion is tool long, there are some repetitions, and some sentences are out of the scope. I would work further on this part of the MS by being more succinct and straight to the point.
Good luck
Simon Blanchet
Reviewed by Bart Haegeman, 07 Oct 2022
This paper deals with the way intraspecific variability (IV) is generated in modelling studies on the role of IV on community diversity. The authors distinguish two types of IV, which they call structured (sIV) and unstructured IV (uIV), and argue that these two types of IV can lead to very different model outcomes.
I think the paper possibly makes an important point, but I'm confused about what exactly it establishes. My confusion starts with the authors' focus on IV generated by the variation in different local environmental conditions conspecifics experience. Is this type of IV considered to be dominant in nature? While in the introduction and in the discussion other types of IV are briefly mentioned (in particular, IV generated by genetic differences between conspecifics), I am missing justification for the focus on environment-driven IV.
Secondly, the modelling approach is largely based on starting with a model that only contains sIV (i.e. no uIV), replacing part of this sIV by uIV, and then exploring the effects of this replacement. As a consequence, the precise implementation of this replacement seems to be key, but the authors do not give much justification for it. For example, when in the model part of the sIV is replaced by uIV, this latter IV is also structured in space and time, in the sense that the variability in performance hat{p}_ij, Eq (4), within species i is still generated by conspecifics being located in different sites j. Hence, applying the authors' definitions of sIV and uIV, I would say that this replacement of part of the initial sIV is still sIV, and not uIV as claimed in the paper. This seems an important issue to me. More generally, even if the replaced sIV would clearly classify as uIV, it should be justified that this particular replacement is appropriate for the purpose of the paper, i.e. able to capture the effects of a specific type of IV independently of the particular implementation.
Finally, I would have liked more discussion about the implications of the model results. Would it be possible to illustrate concretely how the findings of this paper change the interpretation of previous modelling studies? Do previous model results change qualitatively when substituting uIV by sIV? Such examples could make the current paper more convincing. It would also be useful to provide guidelines for future modelling studies on IV. How should IV by modelled to get relevant and robust results? And more generally, I would suggest the authors to focus their discussion more on modelling work. For example, how should one adapt the unifying framework of Stump et al in light of the current paper, or how could incorporating genetically-driven IV in the current study modify, or not, the results (beyond simply noticing that genetic differences between individuals would lead to spatial/temporal structure).
In short, to me this paper indicates that modelling work on IV should carefully consider the type of IV and the details of how it's implemented. However, it is unclear to me what the paper establishes beyond this general warning. I think more justification of the chosen approach and more discussion of the implications of the results are needed.
More detailed comments:
* Figure 1: I like the idea of presenting graphically the paper's approach. However, I found it hard to understand certain aspects of this figure before having read the methods section. Panel A: Not clear to me what the clouds of points are representing. If only one variable is varied while keeping fixed all others, then performance vs this one variable should give a curve, right? Panel B: Again not clear to me what is represented here. Is this illustrating the fitting procedure of Eq. 2, with only one unobserved environmental variable? Panel C: Is the %sIV on the x-axis the same as (number of observed environmental variables)/15? If so, it is probably better to just put the number of observed variables. Same for y-axis. Maybe use the arrow labels to refer to the two questions of the introduction and mentioned several times in the main text, rather than introducing three arrows/labels here.
* Methods, paragraph line 56: Details are missing here, e.g, for the conditional autoregressive model. Please provide everything needed to replicate your work. Why not start with a distribution on the interval [0,1], rather than rescale the normal variables?
* Methods, Eqs (1) and (2): The shape of Eq (1) is called triangular, and contrasted with the quadratic of Eq (2). I suppose the triangular shape is referring to dependence of d_ij on x when x is close to x^*? And hence the triangular shape would disappear when removing the square root in the definition of d_ij, Eq (1)? This raises a number of questions. Why choosing a triangular shape? The empirical performance curves (performance vs environmental variable) I know behave as a quadratic function close to their optimum. Taking instead a triangular-shaped optimum here looks artificial. Moreover, this triangular shape cannot be fitted well by a quadratic function, so this choice will (artificially) increase the difference between perfect and imperfect knowledge models. How would your results change when defining d_ij without the square root in Eq (1)? Maybe use "~" or something similar instead of "=" in Eq (2), because this is a fitting model, and not an equality as in Eqs (3) and (4).
* Methods, line 97: "second question", maybe also briefly recall the procedure for the first question.
* Methods, line 101: "estimated from", do you mean "generated as"?
* Methods, line 131: "probability to die is proportional to performance", should this be "inversely proportional"?
* Methods, line 144: "in the imperfect knowledge model colonization depends on ...", so the performance hat{p}_ij of Eqs (3) and (4) is used here instead of p_ij? I suppose the same is true for the performance-dependent mortality? That is, where p_ij is used in the perfect knowledge model, hat{p}_ij of Eqs (3) and (4) are used in the imperfect knowledge models?
* Methods, line 177: "with the perfect knowledge model", I suppose the same is true when quantifying site sorting for the imperfect knowledge models?
* Figure 2: Maybe use pink for the y-axis label on the right, so that it's clear that the right-hand y-axis refers to the pink points/curve/ribbon. In the legend: replace "an configuration" by "a configuration ExS"
* Figure 3: In panel A, why is there a remaining difference between the case of 15 observed environmental variables and the perfect knowledge model?
* Figure 4: In the legend: replace "one the one hand" by "on the one hand"
* Discussion, line 215: "unrealistic communities", this seems to refer to communities that are different from those of the reference model (the model with only sIV). Are these communities unrealistic in the sense of not corresponding to empirical data? If not, I think it's better to avoid the term "(un)realistic" (used several times in the text).
* Discussion, line 242: "a neutral mechanism", given the variable interpretations of this term among ecologists, maybe describe more explicitly what you mean by this.
* Discussion, line 263: "this was however only due to", where is this shown?
* Discussion, line 269: "as expected ..." until end of paragraph: Unclear to me what this is referring to.