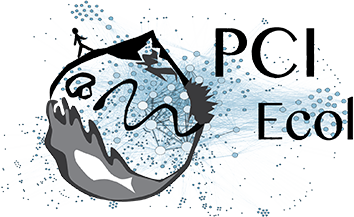
A flexible pipeline combining clustering and correction tools for prokaryotic and eukaryotic metabarcoding
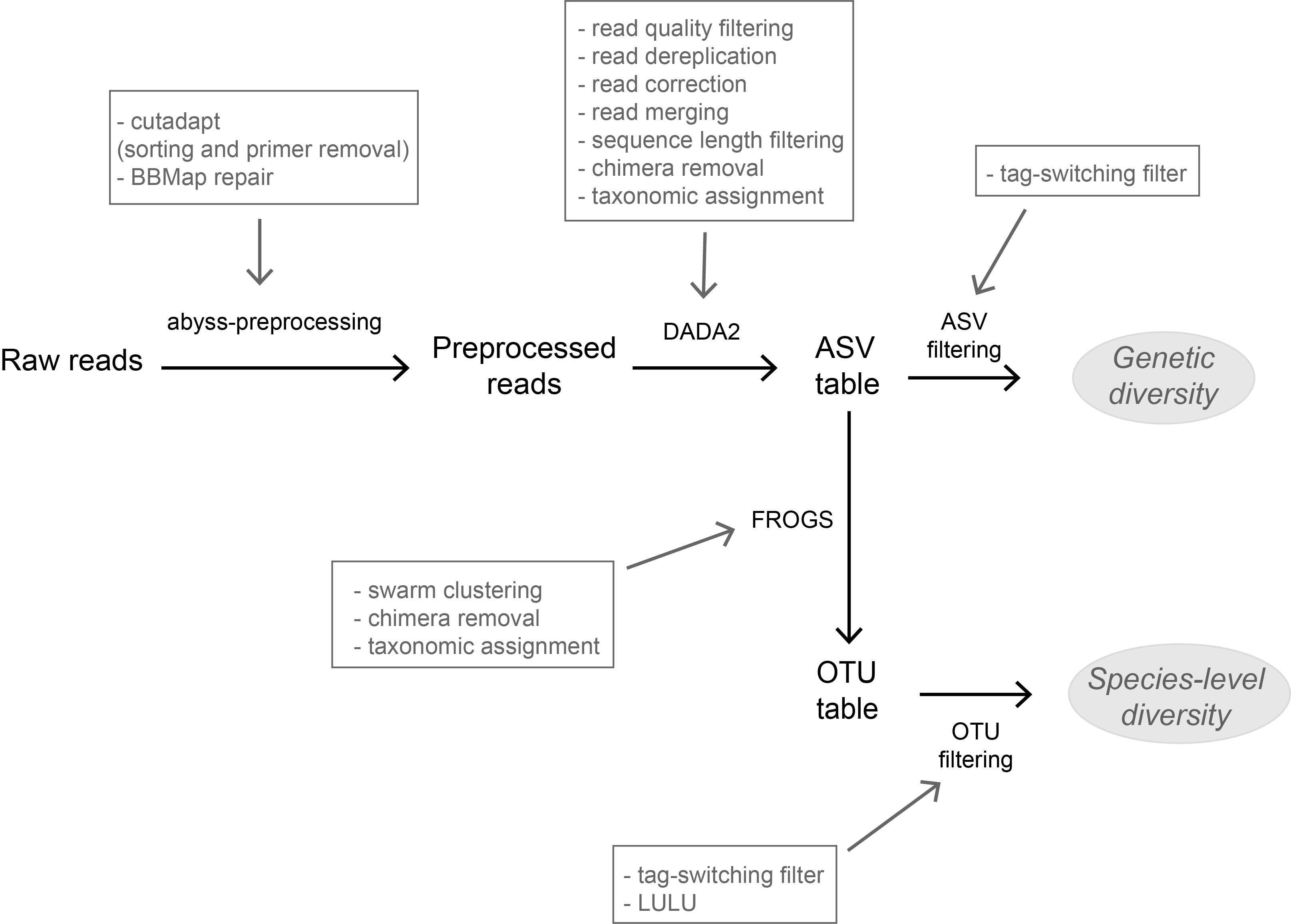
A flexible pipeline combining clustering and correction tools for prokaryotic and eukaryotic metabarcoding
Abstract
Recommendation: posted 30 January 2020, validated 05 February 2020
Kamenova, S. (2020) A flexible pipeline combining clustering and correction tools for prokaryotic and eukaryotic metabarcoding. Peer Community in Ecology, 100043. 10.24072/pci.ecology.100043
Recommendation
High-throughput sequencing-based techniques such as DNA metabarcoding are increasingly advocated as providing numerous benefits over morphology‐based identifications for biodiversity inventories and ecosystem biomonitoring [1]. These benefits are particularly apparent for highly-diversified and/or hardly accessible aquatic and marine environments, where simple water or sediment samples could already produce acceptably accurate biodiversity estimates based on the environmental DNA present in the samples [2,3]. However, sequence-based characterization of biodiversity comes with its own challenges. A major one resides in the capacity to disentangle true biological diversity (be it taxonomic or genetic) from artefactual diversity generated by sequence-errors accumulation during PCR and sequencing processes, or from the amplification of non-target genes (i.e. pseudo-genes). On one hand, the stringent elimination of sequence variants might lead to biodiversity underestimation through the removal of true species, or the clustering of closely-related ones. On the other hand, a more permissive sequence filtering bears the risks of biodiversity inflation. Recent studies have outlined an excellent methodological framework for addressing this issue by proposing bioinformatic tools that allow the amplicon-specific error-correction as alternative or as complement to the more arbitrary approach of clustering into Molecular Taxonomic Units (MOTUs) based on sequence dissimilarity [4,5]. But to date, the relevance of amplicon-specific error-correction tools has been demonstrated only for a limited set of taxonomic groups and gene markers.
The study of Brandt et al. [6] successfully builds upon existing methodological frameworks for filling this gap in current literature. By proposing a bioinformatic pipeline combining Amplicon Sequence Variants (ASV) curation with MOTU clustering and additional post-clustering curation, the authors show that contrary to previous recommendations, ASV-based curation alone does not represent an adequate approach for DNA metabarcoding-based inventories of metazoans. Metazoans indeed, do exhibit inherently higher intra-specific and intra-individual genetic variability, necessarily leading to biased biodiversity estimates unbalanced in favor of species with higher intraspecific diversity in the absence of MOTU clustering. Interestingly, the positive effect of additional clustering showed to be dependent on the target gene region. Additional clustering had proportionally higher effect on the more polymorphic mitochondrial COI region (as compared to the 18S ribosomal gene). Thus, the major advantage of the study lies in the provision of optimal curation parameters that reflect the best possible balance between minimizing the impact of PCR/sequencing errors and the loss of true biodiversity across markers with contrasting levels of intragenomic variation. This is important as combining multiple markers is increasingly considered for improving the taxonomic coverage and resolution of data in DNA metabarcoding studies.
Another critical aspect of the study is the taxonomic assignation of curated OTUs (which is also the case for the majority of DNA metabarcoding-based biodiversity assessments). Facing the double challenge of focusing on taxonomic groups that are both highly diverse and poorly represented in public sequence reference databases, the authors failed to obtain high-resolution taxonomic assignments for several of the most closely-related species. As a result, taxa with low divergence levels were clustered as single taxonomic units, subsequently leading to underestimation of true biodiversity present. This finding adds to the argument that in order to be successful, sequence-based techniques still require the availability of comprehensive, high-quality reference databases.
Perhaps the only regret we might have with the study is the absence of mock community validation for the prokaryotes compartment. Even though the analyses of natural samples seem to suggest a positive effect of the curation pipeline, the concept of intra- versus inter-species variation in naturally occurring prokaryote communities remains at best ambiguous. Of course, constituting a representative sample of taxonomically-resolved prokaryote taxa from deep-sea habitats does not come without difficulties but has the benefit of opening opportunities for further studies on the matter.
References
[1] Porter, T. M., and Hajibabaei, M. (2018). Scaling up: A guide to high-throughput genomic approaches for biodiversity analysis. Molecular Ecology, 27(2), 313–338. doi: 10.1111/mec.14478
[2] Valentini, A., Taberlet, P., Miaud, C., Civade, R., Herder, J., Thomsen, P. F., … Dejean, T. (2016). Next-generation monitoring of aquatic biodiversity using environmental DNA metabarcoding. Molecular Ecology, 25(4), 929–942. doi: 10.1111/mec.13428
[3] Leray, M., and Knowlton, N. (2015). DNA barcoding and metabarcoding of standardized samples reveal patterns of marine benthic diversity. Proceedings of the National Academy of Sciences, 112(7), 2076–2081. doi: 10.1073/pnas.1424997112
[4] Callahan, B. J., McMurdie, P. J., and Holmes, S. P. (2017). Exact sequence variants should replace operational taxonomic units in marker-gene data analysis. The ISME Journal, 11(12), 2639–2643. doi: 10.1038/ismej.2017.119
[5] Edgar, R. C. (2016). UNOISE2: improved error-correction for Illumina 16S and ITS amplicon sequencing. BioRxiv, 081257. doi: 10.1101/081257
[6] Brandt, M. I., Trouche, B., Quintric, L., Wincker, P., Poulain, J., and Arnaud-Haond, S. (2020). A flexible pipeline combining clustering and correction tools for prokaryotic and eukaryotic metabarcoding. BioRxiv, 717355, ver. 3 peer-reviewed and recommended by PCI Ecology. doi: 10.1101/717355
The recommender in charge of the evaluation of the article and the reviewers declared that they have no conflict of interest (as defined in the code of conduct of PCI) with the authors or with the content of the article. The authors declared that they comply with the PCI rule of having no financial conflicts of interest in relation to the content of the article.
Evaluation round #1
DOI or URL of the preprint: https://doi.org/10.1101/717355
Version of the preprint: 1
Author's Reply, 30 Dec 2019
Decision by Stefaniya Kamenova
, posted 14 Nov 2019
Dear authors,
All my apology for the delay - finding suitable reviewers accepting to evaluate your work proved difficult, especially during the summer period. Nevertheless, we managed to collect two high-quality reviews, whose comments and suggestions you can find here below as well as directly within the manuscript. Both reviewers and I find that your study adresses an interesting and very relevant topic pertaining to the analysis and interpretation of DNA metabarcoding datasets from biodiversity inventories. Overall, papers's methodology is sound with very high quality of writing. However, further clarification of methods as well as a better justification of the bioinformatic pipeline parameters choice are required in light of existing literature. Additional suggestions made by the reviewers (with few comments from my side) should help improving the overall quality of the manuscript.
I would recommend to incorporate the revisions suggested and re-submit your article. My feeling is that there will be no need for a second round of reviews but I will have to assess this upon the reception of your revision.
Looking forward to see the revised paper! Best Stefaniya Kamenova
Download recommender's annotationsReviewed by Tiago Pereira, 17 Sep 2019
The study by Brandt et al. (A flexible pipeline combining bioinformatic correction tools for prokaryotic and eukaryotic metabarcoding) brings new insights into data analysis of metabarcoding datasets covering both prokaryotes and eukaryotes as well as mitochondrial (e.g. COI) and nuclear genes (e.g. 18S and 16S), particularly with the inclusion and testing of new methods/bioinformatic tools (e.g. DADA2 and LULU). It is an interesting and well-written paper, likely to be very useful to many biologists/ecologists dealing with these types of datasets. The reviewer has done minor comments/changes in the pdf file (see attachment). Additionally, the authors should consider the following major points:
- Expected relative abundance: multi copy nature of rRNA genes, PCR bias, etc., might confound our expectations. How close is it good enough?
- Intragenomic/intraspecific polymorphism: is this a real problem? Can we alleviate by using phylogenetic methods?
- General trend/patterns: although the different methods produced different results (e.g. alpha/beta diversity), how strongly did they impact the overall pattern?
- In the pipeline, what seems to be the crucial step (e.g. clustering methods/thresholds or taxonomic assignment) in order to produce realible/accurate findings with respect to biodiversity and ecological patterns?
Finally, the reviewer recommends the preprint to be published after minor revisions.
Download the reviewReviewed by anonymous reviewer 1, 02 Nov 2019
Brandt et al., present a study on bioinformatic processing of metabarcoding data that implements two currently wide applied tools (DADA2 and Swarm) in combination with a post-clustering tool (LULU). By proposing to combine DADA2 and Swarm, their study allows another perspective on the debate whether ASVs or OTUs should be used for metabarcoding datasets. However, this combination (and the further post-clustering process with LULU) opens up some major issues, which have to be addressed before I see this manuscript ready for publication. Especially the choice of some parameters is not justified properly. I will focus in my review on these major issues.
That being said, large parts of the manuscript read very well and there are few corrections needed on the language. My review of the language will therefore be very short and does not include typos (but there are some!). I would suggest, though, to re-structure the order of some paragraphs, which might improve the reading experience of the manuscript even further.
Major concerns:
i) The authors present their study -and especially the implementation of LULU- as a novel approach for studying metazoan diversity. However, a quick literature search returned another study from 2018 by Stefanni et al., that also targeted the COI and 18S gene for analyzing metazoan metabarcoding data with LULU (Stefanni et al., 2018; Multi-marker metabarcoding approach to study mesozooplankton at basin scale. Scientific Reports 8:12085). Stefanni et al., made some different choices regarding their bioinformatic pipeline, but their work and results should at least be discussed in the context of the current manuscript here. In general, I have some doubts regarding the extent of novelty presented by Brandt and colleagues. Using LULU in combination with DADA2 was originally tested by Frøslev et al., 2017 on plant data. I am not convinced that simply applying the same combination on metazoan, eukaryotic and prokaryotic data is enough for a study that proposes a ‘flexible pipeline combining bioinformatic correction tools’, because neither tool was developed by the authors, nor is said combination a novel idea of the authors. Maybe the authors refer to the combination of DADA2 and Swarm for being the proposed novel flexible pipeline. If that is what they are aiming at, they may want to consider putting the combination of DADA2 and Swarm (and LULU) in the focus. Momentarily it reads as the focus is on DADA2 and LULU.
ii) Several parameters were chosen in the bioinformatic pipeline that are currently not justified in the text. The most prominent example is Swarm’s d value, which is set to 4 for 18S data, 6 for COI data and 1 for 16S data (lines 261-262). I am aware of only few studies that do not use Swarm’s default of d=1, most likely because the results become harder to interpret. Allowing a difference of one nucleotide between two sequences in one OTU can easily be justified by naturally occurring sequence variation or artificially introduced sequencing errors. Every value beyond d=1 is harder to justify and may be just as arbitrary as the clustering thresholds the authors try to avoid. In fact, I was surprised that the authors use the avoidance of arbitrary sequence similarity clustering thresholds as an argument for Swarm (lines 54-55 and 113-115), but then try to set d to a value that mimics a 1% sequence divergence threshold, which is just the invers of a 99% sequence similarity threshold (lines 349-351). The situation gets even worse, because Swarm OTUs clustered with a different d value are pooled and analyzed in the same context. In my opinion, OTUs that are analyzed together should always be treated as similar as possible. I suppose the size of the 18S V1/V2 region is nearly as long as the 16S V4/V5 region; why were then so different thresholds chosen for the clustering of the respective OTUs? The authors need to justify these decisions and if they cannot come up with scientifically sound justifications, they should consider sticking to those values that are justifiable.
Other more or less arbitrary values for which I found no explanation or justification were the maximum error rate for primer removal in CUTADAPT (lines 231-232), the truncation length, maximum expected error rates (line 243) as well as the minimum overlap for paired-end assembly (line 247) in DADA2, the very low identity (70%) cutoff for BLAST (line 254) and the minimum match values for LULU (line 280). All of these parameters have a severe effect on downstream data processing and ultimately on the results. Maybe the authors chose the values for a good reason or they followed default values from the literature. But without further explanations, the readers cannot understand their decisions and I would not recommend using a bioinformatic pipeline that does not inform about such important steps.
iii) In abstract and introduction, the authors make a point about the importance of multiple marker metabarcoding approaches. However, they conclude that DADA2 is not fit for analyzing metabarcoding datasets of metazoan organisms (lines 504-507). In contrast to this finding, there are at least two publications that analyzed metazoan metabarcoding datasets with DADA2 and did not report the problems presented by the authors here. One of the publications used the 18S V4 marker region and was cited by the authors (Xiong & Zhan 2018), the other publication used the 18S V9 marker region and was not cited by the authors (Leff et al., 2018; Predicting the structure of soil communities from plant community taxonomy, phylogeny, and traits. ISME Journal 12:1794-1805). These studies show that i) the conclusions about metazoan metabarcoding data drawn by the authors on base of the COI region cannot be generalized to all gene regions and ii) the authors may have targeted a less suited gene region for their approach. In any case the results of the current study should be discussed in the context of these previous studies.
Although I admit that it is a tedious topic, I was also surprised about the author’s choice of the 18S V1/V2 region instead of the more commonly used V4 or V9 region. Can the authors please comment on why V1/V2 was chosen? Much more reference data seems to be available for V4 and V9. Since correct taxonomic assignments were an important topic in the current study, using a marker gene for which more reference data is available would have been beneficial for the authors’ study design.
Minor comments:
- Two sentences I struggled the most with:
‘As metabarcoding with multiple markers, spanning several branches of the tree of life is becoming more accessible, bioinformatic pipelines need to accommodate both micro- and macro biologists.’ (lines 2-4).
‘The results also confirm an important variation in the amplification success across taxa (Bhadury et al., 2006; Carugati, Corinaldesi, Dell’Anno, & Danovaro, 2015), supporting the present approach combining nuclear and mitochondrial markers to achieve more comprehensive biodiversity inventories (Cowart et al., 2015; Drummond et al., 2015; Zhan, Bailey, Heath, & Macisaac, 2014).’ (lines 542-546).
Could you please rephrase to make it clearer to the reader what you want to express?
- The numbering of the manuscript sections is askew. Introduction should be ‘1’, but Methods ended up being ‘1’ and so on.
- Reference style is not uniform. For instance: ‘Bista et al., 2015‘ next to ‘Deiner, Fronhofer, Mächler, Walser, & Altermatt, 2016‘ (line 36).
- Singletons consist of only one read. If the OTU consists of two reads, it is a doubleton (line 68). By the way, DADA2 is very effective in removing singletons (see Callahan et al., 2016). Thus, if you think that singleton removal ‘…is arbitrary and potentially hinders the detection of rare species.’ you should not use DADA2.
- Though different important topics are mentioned in the introduction it is not getting absolutely clear what the authors aim to achieve and how they want to do it. Especially the late mentioning of Swarm and how this algorithm will be connected to what had been said before is confusing.
- What do the authors mean by amplicons obtained from negative controls (lines 317-318)? They cannot possibly refer to negative controls of the PCR that yielded amplicons? I am sure there must be another explanation, but could not find it in the manuscript’s methods section. There is just the cryptic sentence ‘Negative extraction controls were included in each extraction run.’ (line 152). Could you please explain what exactly these controls are, what you used them for and why they had been pooled with the rest of the amplicons?
- Do more abundant species in the mock communities lead to more ASVs/OTUs?
- Table 1: Maybe the comparison of the pipelines’ results could also be presented as a figure. All these numbers separated by a slash are hard to read and may look more impressive e.g. in barplots.
- Table 2: Could also be a ‘real’ colored heatmap.
- I struggled with the order of the paragraphs and would ask the authors to disentangle the results of the mock community approach from the results of the ‘true’ samples. One possibility is to restrict oneself first to the mock community results, because they allow for setting the further results in a context. Then present the alpha- and beta-diversity results of the ‘true’ samples.