
The complexity of predicting mortality in trees
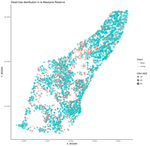
Comparing statistical and mechanistic models to identify the drivers of mortality within a rear-edge beech population
Abstract
Recommendation: posted 12 December 2020, validated 06 January 2021
DeSoto, L. (2020) The complexity of predicting mortality in trees. Peer Community in Ecology, 100070. 10.24072/pci.ecology.100070
Recommendation
One of the main issues of forest ecosystems is rising tree mortality as a result of extreme weather events (Franklin et al., 1987). Eventually, tree mortality reduces forest biomass (Allen et al., 2010), although its effect on forest ecosystem fluxes seems not lasting too long (Anderegg et al., 2016). This controversy about the negative consequences of tree mortality is joined to the debate about the drivers triggering and the mechanisms accelerating tree decline. For instance, there is still room for discussion about carbon starvation or hydraulic failure determining the decay processes (Sevanto et al., 2014) or about the importance of mortality sources (Reichstein et al., 2013). Therefore, understanding and predicting tree mortality has become one of the challenges for forest ecologists in the last decade, doubling the rate of articles published on the topic (*). Although predicting the responses of ecosystems to environmental change based on the traits of species may seem a simplistic conception of ecosystem functioning (Sutherland et al., 2013), identifying those traits that are involved in the proneness of a tree to die would help to predict how forests will respond to climate threatens.
Modelling tree mortality is complex, involving multiple factors acting simultaneously at different scales, from tree genetics to ecosystem dynamics and from microsite conditions to global climatic events. Therefore, taking into account different approaches to reduce uncertainty of the predictions is needed (Bugmann et al., 2019). Petit-Cailleux et al. (2020) uses statistical and process-based models to detect the main mortality drivers of a drought- and frost-prone beech population. Particularly, they assessed the intra-individual characteristics of the population, that may play a decisive role explaining the differences in tree vulnerability to extreme weather events. Comparing the results of both analytical approaches, they find out several key factors, such as defoliation, leaf phenology and tree size, that were consistent between them. Even more, the process-based model showed the physiological mechanisms that may explain the individual vulnerability, for instance higher loss of hydraulic conductance may increase the mortality risk of trees with early budburst phenology and large stem diameter. The authors also successfully model annual mortality rate with a linear relationship including only three parameters: loss of conductance, biomass of reserves and late frost days.
This valuable study is a good example of the complexity in understanding and predicting tree mortality. The authors carried out the ambitious commitment of studying the inter-annual variation in mortality with 14-year dataset. However, it might be not enough time to control for the dependence of temporal data to soundly model mortality rate. The authors also acknowledge that the use of two approaches increases the knowledge from different perspectives, but at the same time comparing their results is difficult because the parameters used are not identical. Particularly, process-based models tend to consider the same microclimatic conditions for every tree in the population, and may produce inconsistences with statistical models. Alternatively, individual-based modelling might overcome some of the incompatibilities between the approaches (Zhu et al., 2019).
(*) Number (and percentage) of articles found in Web of Sciences after searching (December the 10th, 2020) “tree mortality”: from 163 (0.006%) in 2010 to 412 (0.013%) in 2020.
References
Allen et al. (2010). A global overview of drought and heat-induced tree mortality reveals emerging climate change risks for forests. Forest ecology and management, 259(4), 660-684. doi: https://doi.org/10.1016/j.foreco.2009.09.001
Anderegg et al. (2016). When a tree dies in the forest: scaling climate-driven tree mortality to ecosystem water and carbon fluxes. Ecosystems, 19(6), 1133-1147. doi: https://doi.org/10.1007/s10021-016-9982-1
Bugmann et al. (2019). Tree mortality submodels drive simulated long‐term forest dynamics: assessing 15 models from the stand to global scale. Ecosphere, 10(2), e02616. doi: https://doi.org/10.1002/ecs2.2616
Franklin, J. F., Shugart, H. H. and Harmon, M. E. (1987) Death as an ecological process: the causes, consequences, and variability of tree mortality. BioScience, 37, 550–556. doi: https://doi.org/10.2307/1310665
Petit-Cailleux, C., Davi, H., Lefèvre, F., Garrigue, J., Magdalou, J.-A., Hurson, C., Magnanou, E. and Oddou-Muratorio, S. (2020) Comparing statistical and mechanistic models to identify the drivers of mortality within a rear-edge beech population. bioRxiv, 645747, ver 7 peer-reviewed and recommended by Peer Community in Ecology. https://doi.org/10.1101/645747
Reichstein et al. (2013). Climate extremes and the carbon cycle. Nature, 500(7462), 287-295. doi: https://doi.org/10.1038/nature12350
Sevanto, S., Mcdowell, N. G., Dickman, L. T., Pangle, R., and Pockman, W. T. (2014). How do trees die? A test of the hydraulic failure and carbon starvation hypotheses. Plant, cell & environment, 37(1), 153-161. doi: https://doi.org/10.1111/pce.12141
Sutherland et al. (2013). Identification of 100 fundamental ecological questions. Journal of ecology, 101(1), 58-67. doi: https://doi.org/10.1111/1365-2745.12025
Zhu, Y., Liu, Z., and Jin, G. (2019). Evaluating individual-based tree mortality modeling with temporal observation data collected from a large forest plot. Forest Ecology and Management, 450, 117496. doi: https://doi.org/10.1016/j.foreco.2019.117496
The recommender in charge of the evaluation of the article and the reviewers declared that they have no conflict of interest (as defined in the code of conduct of PCI) with the authors or with the content of the article. The authors declared that they comply with the PCI rule of having no financial conflicts of interest in relation to the content of the article.
SOM and HD were funded by the EU ERA-NET BiodivERsA projects TIPTREE (BiodivERsA2-2012-15) and the ANR project MeCC (ANR-13-ADAP-0006). CP received funding from the European Union’s Horizon 2020 research and innovation programme under grant agreement No. 676876 (GenTree)
Evaluation round #3
DOI or URL of the preprint: https://doi.org/10.1101/645747
Version of the preprint: 4
Author's Reply, 23 Nov 2020
Please find enclosed the preprint entitled “Comparing statistical and mechanistic models to identify the drivers of mortality within a rear-edge beech population”, by Petit-Cailleux et al. This is the third revision of the preprint previously assessed by Lucía DeSoto (as recommender) and three PCIEcology reviewers on 2019/08/13, on 2020/02/07, and then on 2020/07/09
We thank the recommender Lucía DeSoto for her additional comments, to which we answer in detail below, and which were mostly all accounted for in the revised manuscript (changes are highlighted in blue in the main text).
1) Title. Since “combine” means come together or mix into a one unit and the authors did not join the two approaches, the title should be changed into “use” or “compare” or any other suitable verb.
Petit-Cailleux et al. : We changed “combining” into “comparing”
2) L68. Please, change “repeated drought” into “droughts”. Petit-Cailleux et al.: Done
3) L98. Please, change “phenomenological” into “observational”. Petit-Cailleux et al.: Done
4) L101. Please, remove “population- or individual-level”. Petit-Cailleux et al.: Done
4) L133-142. Please, move to Materials and Methods.
Petit-Cailleux et al.: We agree that paragraph dedicated to the description of species can usually be found either in the Introduction or in the Materials and Methods sections of manuscript. In our case, we would really prefer to keep it in the introduction first because we argue why this species is particularly suitable to investigate tree mortality driven by climate (and these arguments sound convincing for one of the reviewers, L Hulsman, in a former round of review). Moreover, we kept this paragraph rather short and non-descriptive.
5) L181. Please, change “cows” into “cattle” or perhaps better “livestock and large hervivores”. Petit-Cailleux et al.: Changed in “livestock and large herbivores”
6) L184. Please, specify, “two soil pits” per tree?
Petit-Cailleux et al.: We added: “in the protected plot”. These two soil pits were dug in areas representative of beech presence
7) L190-192. How frequent are multi-stemmed trees? Please, explain briefly here and also add that multi-stem is considered in the analyses.
Petit-Cailleux et al.: We added some clarification L189-190.
8) L305. What do you mean by “able to defoliate”? is it an actual ecological trait? did it change between years? Please, explain it briefly.
Petit-Cailleux et al.: Indeed, we consider in the model the ability of defoliate as an ecological trait which vary among individuals. It is well recognised by the scientific community that this trait vary among species, and that some “drought-avoider” species evolved the ability to lose their leaves and reduce their need for water due to transpiration (e.g. Pivovaroff et al 2016). In our model, we consider that some trees can defoliate, and the fraction of falling leaves is correlated to the percent loss of conductance which depends on the climate of a given year (so the level of defoliation can vary among years for trees able to defoliate). By contrast, some other individuals are not able to defoliate it and have to undergo the drought.
Pivovaroff, A. L., Pasquini, S. C., De Guzman, M. E., Alstad, K. P., Stemke, J. S., & Santiago, L. S. (2016). Multiple strategies for drought survival among woody plant species. Functional Ecology, 30(4), 517-526.
9) L328. What does “four” mean here? Please, clarify.
Petit-Cailleux et al.: We considered four models with the same construction (see equation 8) but differing for the variable describing the competition effect. These different variables are separated by "OR" in equation 8.
10) L340. Please, add citation.
Petit-Cailleux et al.: Done (Bartoń, Kamil. 2020. MuMIn: Multi-Model Inference. R package version 1.43.17. https://CRAN.R-project.org/package=MuMIn)
11) L341. It is not clear when you selected models using AIC and when you did not. Please review this paragraph and Result section (L409) in order to clarify it.
Petit-Cailleux et al.: We modified the paragraph L330-331) and removed the confusing sentence in the paragraph L.409
12) L353. Please, define the “G” as Generalized. Petit-Cailleux et al.: Done
13) L389. There is not inter-individual variation for CVI (grey shadow), why? Please, explain it.
Petit-Cailleux et al.: We added inter-individual variation for CVI to the plot on Figure 2
14) L431. Please, change “Individual variation in vulnerability simulated with CASTANEA” into “Inter-individual variation in vulnerability simulated with CASTANEA”. Change also throughout the whole manuscript. Also, move this section above the statistical models to keep CASTANEA results together.
Petit-Cailleux et al.: Done
15) L435-440. Please, show the posthoc analyses that support these results (differences between levels of the effects) in figure 4 (using different letters) or in a table supplementary material.
Petit-Cailleux et al.: We did not perform such posthoc analyses on CASTANEA results. Indeed, CASTANEA is a mechanistic model, without stochasticity (a given tree under a given climate will always produce the same results in terms of output variables). Our goal here was to qualify differences in the main output variables of interest, using a simple simulation design with only eight simulated individuals.
16) L440-445. Please, cite the figure or table that shows these results.
Petit-Cailleux et al.: We added the new table S2 containing the mean and the standard deviation of the GPP, NPP, LAI initial for each year and the autotrophic respiration for the trees of the second simulation series (with initial DBH of 5cm, 15cm, 30cm and 40cm).
17) L453. Please, include the studied variable, mortality rate, in the caption of figure 3. For instance, change into “Interaction effects in the logistic regression model at individual-level” into “Interaction effects in the logistic regression model for mortality rates”.
Petit-Cailleux et al.: Done
18) L461. Which is shown in figure 4, the mean values? Please, review the caption in accordance. Add PLC: percentage of loss conductance; BoR: biomass of reserves. Please, also add the standard errors (or confidence intervals) in the figure. They are not the mean value but the value of each trees.
Petit-Cailleux et al.: Setting standard errors is not possible (see also answer to question 15). Indeed, as specified L340 (previous manuscript) and in the title of the figure, there are only 8 trees representing the crossing of the characteristics of interest (size 5 or 40 DBH, ability to defoliate or not and early or average budburst). In addition to the peculiarity of each year written in the x-axis (drought, late frosts and no stress), we have added the year above the dots for clarity.
19) L496. Change “at population-level” into “in our studied population”. Be careful, you cannot use at population-level in your study because, by analysing only one population, you cannot infer that any difference you obtain is because of a population effect. Change also “at population level/scale” into “in the studied population” in caption of figure 1 (L163), L187 and L308.
Petit-Cailleux et al.: We agree with you and changed population level/scale into studied population or forest stand in the whole manuscript.
20) L518-520. What do you mean here? Please, clarify. For instance, change into “to test the individual vulnerability to climatic hazards (drought and late frost) and to biotic pressures (competition and the presence of a fungus)”.
Petit-Cailleux et al.: Done
21) L560. Figure S7 is not in the Supplementary material. Figure S6 is not cited in the main text. Please, review.
Petit-Cailleux et al.: We removed these useless figures.
22) L594-599. Please, remove. See point 19, and be careful, your study only have one spatial scale.
Petit-Cailleux et al.: We have changed the term 'population' to forest stand. Indeed, we studied the individual variability influencing the individual mortality risk and we also explained the variation in the stand mortality rate. That's why we think that in this case we can keep these two scales.
23) Figure S2. Please, 200(100)mm of precipitation should be aligned with 20(10)ºC in an ombrothermic diagram.
Petit-Cailleux et al.: The ombrothermic diagram was corrected an redrawn.
24) Figure S3. Please, make transparent the beech dots. Petit-Cailleux et al.: Done
25) Please, remove “at population level” in Appendix.
Petit-Cailleux et al.: We changed population into forest stand in the Appendix.
Decision by Lucía DeSoto, posted 09 Jul 2020
Dear authors,
I received the reviews of the revision of your manuscript entitled “Combining statistical and mechanistic models to identify the drivers of mortality within a rear-edge beech population”. The author successfully addressed and changed most of the issues identified.
The manuscript has been improved after the thoroughly revision, although there are still some minor issues that need to be review. Number of lines (L) are indicated for the .pdf file “Petit-CailleuxetalPCIEcologymainversion3_markedup.pdf”.
1) Title. Since “combine” means come together or mix into a one unit and the authors did not join the two approaches, the title should be changed into “use” or “compare” or any other suitable verb.
2) L68. Please, change “repeated drought” into “droughts”.
3) L98. Please, change “phenomenological” into “observational”.
4) L101. Please, remove “population- or individual-level”.
4) L133-142. Please, move to Materials and Methods.
5) L181. Please, change “cows” into “cattle” or perhaps better “livestock and large hervivores”.
6) L184. Please, specify, “two soil pits” per tree?
7) L190-192. How frequent are multi-stemmed trees? Please, explain briefly here and also add that multi-stem is considered in the analyses.
8) L305. What do you mean by “able to defoliate”? is it an actual ecological trait? did it change between years? Please, explain it briefly.
9) L328. What does “four” mean here? Please, clarify.
10) L340. Please, add citation.
11) L341. It is not clear when you selected models using AIC and when you did not. Please review this paragraph and Result section (L409) in order to clarify it.
12) L353. Please, define the “G” as Generalized.
13) L389. There is not inter-individual variation for CVI (grey shadow), why? Please, explain it.
14) L431. Please, change “Individual variation in vulnerability simulated with CASTANEA” into “Inter-individual variation in vulnerability simulated with CASTANEA”. Change also throughout the whole manuscript. Also, move this section above the statistical models to keep CASTANEA results together.
15) L435-440. Please, show the posthoc analyses that support these results (differences between levels of the effects) in figure 4 (using different letters) or in a table supplementary material.
16) L440-445. Please, cite the figure or table that shows these results.
17) L453. Please, include the studied variable, mortality rate, in the caption of figure 3. For instance, change into “Interaction effects in the logistic regression model at individual-level” into “Interaction effects in the logistic regression model for mortality rates”.
18) L461. Which is shown in figure 4, the mean values? Please, review the caption in accordance. Add PLC: percentage of loss conductance; BoR: biomass of reserves. Please, also add the standard errors (or confidence intervals) in the figure.
19) L496. Change “at population-level” into “in our studied population”. Be careful, you cannot use at population-level in your study because, by analysing only one population, you cannot infer that any difference you obtain is because of a population effect. Change also “at population level/scale” into “in the studied population” in caption of figure 1 (L163), L187 and L308.
20) L518-520. What do you mean here? Please, clarify. For instance, change into “to test the individual vulnerability to climatic hazards (drought and late frost) and to biotic pressures (competition and the presence of a fungus)”.
21) L560. Figure S7 is not in the Supplementary material. Figure S6 is not cited in the main text. Please, review.
22) L594-599. Please, remove. See point 19, and be careful, your study only have one spatial scale.
23) Figure S2. Please, 200(100)mm of precipitation should be aligned with 20(10)ºC in an ombrothermic diagram.
24) Figure S3. Please, make transparent the beech dots.
25) Please, remove “at population level” in Appendix.
I invite you to make these minor changes pointed out above and submit a revised manuscript for the recommendation by PCI. I hope they may improve the quality of the manuscript. Please, let me know if you do not agree with any of them.
Thank you for submitting your manuscript to PCI.
Sincerely,
Lucía DeSoto
Additional requirements of the managing board:
As indicated in the 'How does it work?’ section and in the code of conduct, please make sure that:
-Data are available to readers, either in the text or through an open data repository such as Zenodo (free), Dryad or some other institutional repository. Data must be reusable, thus metadata or accompanying text must carefully describe the data.
-Details on quantitative analyses (e.g., data treatment and statistical scripts in R, bioinformatic pipeline scripts, etc.) and details concerning simulations (scripts, codes) are available to readers in the text, as appendices, or through an open data repository, such as Zenodo, Dryad or some other institutional repository. The scripts or codes must be carefully described so that they can be reused.
-Details on experimental procedures are available to readers in the text or as appendices.
-Authors have no financial conflict of interest relating to the article. The article must contain a "Conflict of interest disclosure" paragraph before the reference section containing this sentence: "The authors of this preprint declare that they have no financial conflict of interest with the content of this article." If appropriate, this disclosure may be completed by a sentence indicating that some of the authors are PCI recommenders: “XXX is one of the PCI XXX recommenders.”
Evaluation round #2
DOI or URL of the preprint: https://doi.org/10.1101/645747
Version of the preprint: 2
Author's Reply, 19 Jun 2020
Decision by Lucía DeSoto, posted 07 Feb 2020
Dear authors,
I received the reviews of the revision of your manuscript entitled “Combining statistical and mechanistic models to unravel the drivers of mortality within a rear-edge beech populations.” The three reviewers suggests some minor changes that will help to increase the quality of the manuscript. They also highlight some minor issues that should be corrected or discussed.
Additionally, one of the reviewers has several major concerns that deserve to be reviewed: 1) To make the it more readable, I also think the introduction should be shortened and focus on the objective of the manuscript. 2) I am also not convinced about the population-level approximation, because the authors only study one population, and 13 years, and consequently 13 data. Furthermore, data are autocorrelated between years. I recommend the authors to remove this model (methods and results) from de main text. It can be kept in the supplementary results if it is needed for the CVI discussion . 3) I also agree that inferred models must keep all the explained variables following the rationale of the study. Please, change it accordingly or justify it.
I therefore invite you to respond to the points raised and submit a revised manuscript for a potential recommendation by PCI.
My sincere apologies because of the delay of the review. I hope you will find our concerns and suggestions constructive and valuable.
Sincerely, Lucía DeSoto.
 
Additional requirements of the managing board:
As indicated in the 'How does it work?’ section and in the code of conduct, please make sure that:
-Data are available to readers, either in the text or through an open data repository such as Zenodo (free), Dryad or some other institutional repository. Data must be reusable, thus metadata or accompanying text must carefully describe the data.
-Details on quantitative analyses (e.g., data treatment and statistical scripts in R, bioinformatic pipeline scripts, etc.) and details concerning simulations (scripts, codes) are available to readers in the text, as appendices, or through an open data repository, such as Zenodo, Dryad or some other institutional repository. The scripts or codes must be carefully described so that they can be reused.
-Details on experimental procedures are available to readers in the text or as appendices.
-Authors have no financial conflict of interest relating to the article. The article must contain a "Conflict of interest disclosure" paragraph before the reference section containing this sentence: "The authors of this preprint declare that they have no financial conflict of interest with the content of this article." If appropriate, this disclosure may be completed by a sentence indicating that some of the authors are PCI recommenders: “XXX is one of the PCI XXX recommenders.”
Reviewed by anonymous reviewer 2, 09 Dec 2019
In this article, the authors investigate the causes of mortality of European beech, using a large dataset of yearly measurements of more than 4000 trees in an unmanaged forest located at the rear edge of the distribution range of the species.
They use two types of models
- regression models to assess the relative contributions of drought, competition, growth, size, phenology, defoliation events, pathogenic fungi to individual-level probability of death.
- a process-based model to investigate :
i) at the population level, how modelled, climate-driven carbon reserves, loss of conductance, late frosts contribute are correlated to observed mortality (at the population level). They find that none of the three variables is, but that their combination is well correlated to observed yearly mortality.
ii) at the individual (tree) level, how differences in size, phenology, ability to defoliate would affect these response traits (and mortality) depending upon climate
This is a revised version of an earlier manuscript.
I find it much clearer than previously and I think that the referees’ comments were adequately addressed.
I have 1-2 minor points but am otherwise really happy with this manuscript (which has really improved upon revision):
- I am not quite sure that equation6 is homogeneous.
- One perspective to include late frosts as a climatic variable, would be to use the variable NLF (as computed from CASTANEA) as a predictive variable in the statistical model.
- for the compound vulnerability index, it might be useful to design a multiplicative index (something like
PLC * [NLF/max NLF allowing survival] / [BoR / max BoR ensuring survival])
Reviewed by Lisa Hülsmann
, 08 Jan 2020
I would like to thank the authors for their extensive modifications of the manuscript and the additional clarifications that make the findings more accessible. The authors now point out the novelty of their study more clearly and have better explained how the two model types can be combined. The new overview figure and results table (why in appendix?) and modified introduction lay out the approach and findings more comprehensibly. Finally, the authors have addressed some, but not all, statistical and conceptual issues (see further comments below).
I am still not fully convinced that the approach presented is the best possible: As suggested during the first review round, the authors have fitted a survival model using individual-level mortality data (see Appendix 4). Unfortunately, they use the same set of predictors but include no climatic variability into the model, although jointly testing for individual tree characteristics and climate would be - in my opinion the - biggest advantage of such an approach. I cannot fully understand why the authors conclude that climate cannot be incorporated. Doing so will probably also solve the issue of non-proportional hazards – e.g. when using interactions with climate. Note that I am aware that climate is equal for all trees and that not all individual-level variables are available for every year. A solution could be to simply keep these variables constant or to interpolate between years where applicable. I agree that this is not an ideal solution but still think this is not a reason for collapsing the response data a priori (see previous review round). I think an individual-based model with climatic effects (also in interaction) could make the whole study more meaningful, but I leave it to the authors if such a model should be part of this manuscript.
Further, I see two issues that could be improved in the new version:
(1) The focus and the research questions of the study could be more precise. This applies in particular to the abstract but also to the end of the introduction. It may also be helpful to slightly streamline the introduction to better match the research aims (e.g. I don’t think that the carbon starvation versus hydraulic failure discussion is that important for the findings). In general, the introduction could be more concise, while the end of the discussion may profit from a few concluding sentences including the main findings and their relevance.
(2) The focus on population- versus individual-level processes is my opinion not an ideal way of structuring the findings. Mortality rates are only the result of individual level processes and even if one must collapse the data to a lower resolution (here mortality rates of a population) to identify mortality drivers, this is not an advantage but a compromise. Therefore, I would find it more compelling to structure the findings around the different types of mortality drivers itself rather than the level at which they become effective.
Statistical feedback:
I appreciate the new population-level model that tests for predefined influences and thus suffers less from data dredging. However, I would like to mention that every model selection (both manual or automatic) is in general not a good idea if the aim is inference and not prediction, even if more data is available. I know that this is a common approach in ecology, but the conclusions from reduced models are wrong. This is particularly true for p-values which become smaller and more significant in model selection. In case of the individual-level model, p-values are very small so even in the full model the effects are likely significant. Another problem of model selection is that effect sizes and directions may be wrong, if important confounders are removed from the model during the selection process. In conclusion, model selection should be avoided in case of inference and is recommended for prediction only. For more details see e.g. Georg Heinze, Christine Wallisch et al., Variable selection – A review and recommendations for the practicing statistician. Biometrical Journal 60 (2018), S. 431-449 and D. J. Lederer, S. C. Bell et al., Control of Confounding and Reporting of Results in Causal Inference Studies. Guidance for Authors from Editors of Respiratory, Sleep, and Critical Care Journals. Ann Am Thorac Soc 16 (2019), S. 22-28.
Time series are typically characterized by autocorrelation, e.g. the value of this year depends on the one of last year. One assumption of calculating correlations and regression models is that the observations are independent, which is not true for time series. Ideally, this should be accounted for when correlating mortality rates and PBM outputs and fitting the population-level mortality model. Note that this is not done with using climate as a predictor. Nevertheless, I agree that fitting autocorrelation is probably difficult considering the rather short lengths of the time series.
Specific comments:
Line 35: I would argue that some of this is already known but their remains high uncertainty. So maybe relax this statement a bit.
Line 36: After the intro and research gap sentence, I typically expect a sentence that defines the research aim of the study.
Line 38: Which decline is meant here?
Lines 42-43: To me it is not clear why it is important to emphasize that this is a feature of the population level. The processes are expected to occur on the individual level, and I don’t think it is helpful to emphasize the difference here as it may make the reader to assume that the processes are not important at the individual level. I think the model type is more important than the level of observation.
Lines 74-85: This part may be shortened.
Line 110: stand size?
Line 111: “level” is missing after “individual-“.
Line 127: I think the decrease was not dramatical. “can decrease” is maybe more correct.
Line 174: Do you mean patterns or drivers?
Line 180: “When and how” sounds very vague to me.
Lines 180, 182 and 185: I think “questions” is better than “issues”.
Line 436: I think this is also relative mortality probability!
Line 501: ideally, one should report mortality rates together with the size threshold.
Line 512: The conclusions about hydraulic failure and carbon starvation are not based on empirical evidence but rather reflect the believes about these processes and how they were implemented in CASTANEA. I don’t think that only because the composite index is slightly correlated with mortality rates this is enough to say that both processes indeed cause mortality.
Line 538: From what do you know conclude that the trees die from drought and frost? How about competition?
Lines 590-591: What is meant with “high precision local mortality predictions”?
Lines 591-592: “A weaker ability to generalize” is also mentioned as a disadvantage of PBMs in the intro. This is a bit confusing…
Line 601: You may add a reference to Cailleret, M., Bircher, N., Hartig, F., Hülsmann, L., and Bugmann, H.. 2020. Bayesian calibration of a growth‐dependent tree mortality model to simulate the dynamics of European temperate forests. Ecological Applications 30(1):e02021. 10.1002/eap.2021
Lines 613ff: If “disaggregate” means comparing the contribution of different factors, I would argue that this is not possible with the results of this study. To me this seems possible only if the lower level (individual level) model includes also climate variables.
Line 633: I think the discussion should end with a few conclusions.
Table S2: This could also be an element of the main article. Be more precise about the effects of drought on the mortality rates. What does “when and how” means here? NLF, PLC, BoR and CVI and their correlation with mortality rates could also be a third question, e.g. “Which indicators of tree decline that can be derived from PBM are linked to tree mortality rates?”
Reviewed by anonymous reviewer 1, 03 Dec 2019
This is a revised manuscript. The authors have improved the manuscript based on the review comments and answered all our comments. Figure 1 and Table 1 are important additions to the manuscript to clarify the measured variables and used approaches. Also simplifying the statistical analysis at population scale made the manuscript more clear. However, I think the manuscript still needs some rather minor improvements before it is ready for publication.
Detailed comments:
Line 38: I am not sure what “decline on mortality” means? Maybe just “mortality”.
Lines 42-43: I think it is important to express it clearly in the abstract that mortality rate at population level was associated with all processes simulated with the PBM: conductance loss, carbon reserve depletion and occurrence of late frosts. In the current form, the reader might get a wrong impression that these tree processes were identified among many others to be the most important ones.
Line 160: Rephrase “the right text in square boxes”
Lines 164-166: You make two simulations with CASTANEA: one at population level using a simulation of a stand with 100 trees (and compare the results with measured mortality), and another at individual level investigating the differences in physiological responses between individuals with different characteristics. I think this is not clear from Figure 1 and its figure caption. Also, I would consider including Table S2 into the manuscript. This would clarify things even further.
Line 171: Percentage loss of conductance (delete the extra “of” throughout the manuscript).
Lines 215-216: The division of variables into endogenous and exogenous is not clear to me. For example, stem diameter is affected by both endogeneous (i.e. age) and exogeneous (i.e. competition) factors. How do you define this division, and do you really need such a division?
Table 1: In the mean, min and max, you use varying amount of decimals. Please be consistent.
Line 244: What does an “ordered variable” mean here?
Line 264: “all indices reached a ceiling after this distance value” is odd language. Please rephrase.
Lines 334-335: It is mentioned here that CASTANEA was validated based on ring width patterns and reference to Appendix 2 is given. I think the results of the validation should be summarized here. It should be said that although growth simulated with CASTANEA correlated with observed growth, CASTANEA overestimated growth strongly, and simulated a decreasing trend in growth over time that was not visible in observations.
Figure 2. Use the same axis range for the mortality rate in all subfigures (it now varies from -1 to 3.5, to 0 to 4.5 depending on the figure).
Lines 418-425: This section is about statistical analysis on population scale, and has been greatly condensed since the first version. This decision is good. However, in case any statistical analysis on population scale is maintained, some results should be shown in the manuscript. Now there is nothing (only in the appendix).
Lines 439 and 448: subscript Nstem
Line 486: Either “shed new light” or “gave new insight”
Line 502: remove “automatically”
Line 508: remove two extra commas
Line 517: replace “or” with “and”
Line 533: high mortality in 2007
Line 569: What do you mean by mechanical increase of water need?
Lines 597-599: If you compare measured mortality with variables simulated with CASTANEA, how do you there finely integrate the statistical and simulation approach? Please rephrase.
Evaluation round #1
DOI or URL of the preprint: https://doi.org/10.1101/645747
Author's Reply, 05 Nov 2019
Decision by Lucía DeSoto, posted 13 Aug 2019
Dear authors,
I have solicited three reviews of your manuscript entitled “Combining statistical and mechanistic models to unravel the drivers of mortality within a rear-edge beech populations.” Reviewers agreed that the topic of tree mortality is interesting and the study of its causes as you carried out had merit. However, reviewers also stated that the paper should be clarify because of the complexity of the study. They thoroughly reviewed your manuscript and made noteworthy suggestions to improve its quality.
Based on these concerns, I think your preprint needs improvements. I invite you to respond to each point raised by myself and the reviewers and submit a revised manuscript for a potential recommendation by PCI.
My specific comments highlighting mine and reviewers’ concerns are detailed below:
1. All reviewers raised that methods should be revised in order to better analyse data and fit the models. Also description of the variables used should be reviewed to make them well-defined. For instance, in Table 1 the time (and frequency) when the variables were recorded should be included.
2. One of the reviewers was concern about the population-level models and suggest the authors to focus on individual models, I agree with her and also think that the study lacks of replicates for population. Authors achieved results that may be only reliable for their specific population, and thus locally appealing.
3. Results are difficult to follow because of the large number of variables used. For, instance, other reviewer made the helpful suggestion of using a table that should be not dismiss.
4. All reviewers agree that the introduction is fine but asked for more detailed discussion of the conceptual framework. Particularly, I found that introduction and discussion are vaguely interconnected.
5. As authors aimed “to investigate patterns of mortality within a population located at the warm and dry ecological margin of European beech”, I wondered about the expected results of the study and its explicit hypotheses.
6. Finally, the size of the numbers legends of the figures should be larger.
Sincerely,
Lucía DeSoto
Additional requirements of the managing board:
As indicated in the 'How does it work?’ section and in the code of conduct, please make sure that:
-Data are available to readers, either in the text or through an open data repository such as Zenodo (free), Dryad (to pay) or some other institutional repository. Data must be reusable, thus metadata or accompanying text must carefully describe the data.
-Details on quantitative analyses (e.g., data treatment and statistical scripts in R, bioinformatic pipeline scripts, etc.) and details concerning simulations (scripts, codes) are available to readers in the text, as appendices, or through an open data repository, such as Zenodo, Dryad or some other institutional repository. The scripts or codes must be carefully described so that they can be reused.
-Details on experimental procedures are available to readers in the text or as appendices.
-Authors have no financial conflict of interest relating to the article. The article must contain a "Conflict of interest disclosure" paragraph before the reference section containing this sentence: "The authors of this preprint declare that they have no financial conflict of interest with the content of this article." If appropriate, this disclosure may be completed by a sentence indicating that some of the authors are PCI recommenders: “XXX is one of the PCI XXX recommenders.”
Reviewed by anonymous reviewer 1, 19 Jun 2019
Review for Combining statistical and mechanistic models to unravel the drivers of mortality within a rear-edge beech populations.
This manuscript uses statistical and process-based models to study mortality of Fagus sylvatica at individual and population scale. The main results are that drought and late frost events were the major environmental causes of mortality at the population scale, whereas loss of hydraulic conductance and carbon starvation were the physiological stresses connected to mortality. In individual level, high mortality was related to low growth rate, high crown defoliation, infection by a fungi, high competition, high loss of hydraulic conductance, higher frequency of late frosts, and to early (statistical model) or late (process-based model) budburst. The topic is important and the approach is very promising. However, it is also challenging to report in a clear and structured manner due to its complexity. This still needs improvement before the manuscript is ready for publication. Also, there are several (small) technical mistakes and the language should be improved to be fluent.
I have one more general comment on the paper, and the detailed comments you find at the end of this letter. Statistical models were used to quantify the effects of climate, competition, tree size and health on mortality, and process-based models to find the physiological mechanisms among carbon reserves, hydraulic conductance and late frosts that explain these effects. I like the idea, but my largest confusion is that why did you not write open and discuss the processes that the PLC, carbon starvation and frost damages affect in the simulation model, and what is their role in the simulated mortality (not only measured mortality)? I think this needs to be clearly presented. Also, I would be interested to see the number of late frost days also in the statistical model as it is mainly a climatic variable (although taking into account the timing of bud burst).
Detailed comments:
Page 2, lines 36-37: “…mortality decreased with mean growth, and increased with crown defoliation…” Please write it open that mortality decreased with increasing mean growth and increased with increasing crown defoliation?
Page 2, line 40: Please explain why the frequency of late frost days is studied with the process model? Were frost damages caused by late frost days included in the processes of the simulation model, i.e. did late frost days decrease photosynthetic capacity of the tree due to frost damages or something similar? In general, I think it is important to explain what are the main processes in the model affected by these variables (frost days, PLC and carbon starvation) – even if it could be found from earlier publications. This is essential to understand the studied mechanisms behind the mortality.
Page 2, line 40: “earlier trees” does not mean anything, please write “individuals with earlier bud burst” or something similar.
Page 3, line 73: Remove the word “vessel”
Page 5, lines 128-132: I suggest not to use the term “iconic” here.
Page 5, line 151: You have listed “decline” as a monitored variable. But decline of what?
Page 8, line 225: I think the index that was counted only for trees >3m away from the target tree is Competintra not Competintra+. Please check this.
Page 8, table 1: In the table caption, you refer to tables 1A and 1B, but there is no subtables A and B. So add A and B to the table or remove from the table caption.
Page 8, table 1: How can Competintra minimum and maximum values be larger than those of Competintra+, although the latter one includes more trees (also trees closer than 3 m from the studied tree)? Please check this.
Page 8, line 240: You use altogether seven R packages (If I calculated correctly). This shows clearly the complexity of the paper.
Page 9, line 254: Don’t you have one to three variable per category (the equation below)? Please check this. Also all the equations should be numbered (eq. 1); please correct this throughput the whole manuscript.
Page 9, lines 259-263: Check the abbreviations of all variables in this paragraph, there are confusions. In the equation, you don’t have MeanTDriestQ, but you have MeanTColdestQ. Also, you do not have SPEI_maxn in the equation at all. The sentence is also confusing, please rewrite.
Page 10, lines 278-279: You have competition index and DBH both in the same model. Competition index is derived from DBH, so they are highly correlated. This could have been avoided by using e.g. height as a variable describing tree size.
Page 10, line 303: You need to write what is Oddsnormal in the equation; it is not given.
Page 11, line 308: Write “coarse roots”
Page 11: I do not find information on what are the input parameters for the simulations? There are some climate variables, but which? Please write open clearly.
Page 12, line 352: It is difficult for me to understand why you need such an index variable for a process-based model, when you already include all the variables in the model that constitute the index. If there is a combination effect of these stresses, it should be taken into account in the model with interactions between processes.
Page 12, line 366: Please refer here to fig. 1. Based on the figure, the mortality rate is as high in year 2007 as it is in year 2010. Also in year 2004, the mortality is nearly as high.
Page 12, line 370: Be careful with the variable abbreviations. Check from page 9 which abbreviations you used, and use them consistently (SPEI3JJAn instead of SPEIJJA; PDriestM instead of PdriestM).
Page 13, line 372: What does it mean in practice that the relative mortality rate was decreased by 1.15 and 3.03 times? The likelihood of mortality increased 1.15 and 3.03 times per how big change in SPEI_JJA and PDriestM?
Page 13, line 397: The combined index did not correlate at all with observed mortality in year 2007. On that year, the mortality rate was high, but the index did not catch it. This should also be stated and discussed.
Page 14, Fig. 1: The mortality rate on the second y-axis is not percentages, but shares instead (multiply with 100 to get percentages). Also, use the same scale for the mortality in all sub-figures (now the second figure has different scale than the others). Also, I wonder why it is not shown how the different physiological stresses correlated with the simulated mortality (it only shown how they correlated with measured mortality)? I think it would be informative to show also this as it would show how severe is the effect of each physiological stress to the simulated mortality following the processes and their connection built-in in the simulation model.
Page 15, line 410: You can remove “(i.e. dead individuals truly observed dead)” as this is obvious.
Page 15, lines 412 and 413: See my comment related to page 13, line 372.
Page 16, lines 438-440: How about years 2009 and 2016 that were also good years?
Page 17, line 444: It is difficult to understand why large trees would have lower reserve of carbon than small trees. Can you explain this a bit more?
Page 18, line 484: It does not make sense to give so many decimals in the mortality rates by Archambeau et al. (2019).
Page 18, lines 507-508: Please check the end of this sentence; there is something wrong.
Page 19, lines 522-528: Please rewrite this part. It is really difficult to follow.
Page 19, line 535: I think plants often defoliate to prevent xylem embolism, not because of it (or probably both). Shedding the leaves helps to avoid cuticular transpiration.
Page 21, line 589: I don’t understand what is meant by saying that due to so many recurrent dry years the benefit of defoliation becomes negligible. Can you please write it open?
Reviewed by Lisa Hülsmann
, 31 Jul 2019
In this manuscript, Petit-Cailleux et al. explore and quantify drivers of tree mortality in Fagus sylvatica at the individual and population level using two complementary approaches: (1) fitting statistical models with inventory data from a rear-edge beech population, and (2) applying the mechanistic model CASTANEA to the same site.
As the authors nicely synthesize in their introduction, disentangling the multiple interacting factors that cause tree mortality and appropriately integrating them in dynamic vegetation models are key issues for forest ecology and climate change adaptation. This makes their study an interesting and timely contribution to the field of tree mortality research.
I have, however, methodological and conceptual concerns that restrict the validity of the current conclusions and would like to make several suggestions how the authors can increase the overall significance of their manuscript.
The following choices are, in my opinion, critical and can cause unreliable results: The authors (1) fit a beta-regression model for the population-level mortality rate that has only 14 observations but 28 predictors, while the data points are not even independent because of temporal autocorrelation, (2) carry out strong model selection (for both empirical models) and then interpret p-values, which is statistically inadmissible, and (3) unfortunately collapse their - in fact very valuable - dataset in two ways: on the one hand to population-level mortality rates and keep the temporal variability, and on the other hand to long term mortality probabilities but keep individual-level variability.
Regarding the conceptual framework, I would like to encourage the authors to discuss in more detail how they think mechanistic models should be employed to disentangle the drivers of tree mortality, taking into account that models can only reflect those processes that are implemented in the simulation framework and that the uncertainty for model parameters is typically very large.
In the following, I point out in more detail why I believe the analyses should be improved, what the authors could do instead and where more context and discussion is needed.
Statistical mortality models
Problems of the beta-regression model of annual mortality rates for 14 years:
The model can test for climate and competition effects only, while it is known that large trees may react differently to drought than small trees.
The number of observations available for the population-level model is very small (n=14). The result is that the authors carry out a very strong model selection on 28 predictors. For predictive models, this may be a reasonable approach, but for inference this is a clear example of p-hacking. In this case, the discrepancy between available observations and tested predictors is so large that the resulting model is basically useless.
The model doesn’t account for temporal autocorrelation.
Problems of the logistic regression for individual tree status at the end of the observation period:
The authors don’t make use of the 6 months resolution of the mortality surveys. This is unfortunate because, in my opinion, the high temporal resolution is the biggest strength of their dataset. This would also allow to disentangle the various drivers of mortality, which is actually what the authors promised in their introduction.
The model can test for the effect of tree characteristics and competition only, but not of climate.
To improve the reliability and significance of their empirical models, I suggest that the authors (1) fit only one mortality model that keeps both levels of variability (individual and temporal) using half-annual mortality probabilities of individual trees as a response variable, (2) jointly test for the effect of climate, competition and tree characteristics including also seasonal (winter/summer) effects and lag effects of drought and frost, (3) adequately control for temporal autocorrelation, and (4) choose a well-defined set of predictors based on ecological hypotheses so that model selection is unnecessary.
Mortality at the tree or the population level
The authors carry out both approaches at the tree and the population level. While I agree that population-level mortality rates are intuitive and helpful for reporting trends, I doubt that they are an ideal level for analyzing mortality drivers in inhomogeneous populations. Basically all mortality drivers may have different effects on small versus large trees. In addition, mortality rates can be derived from individual-level mortality probabilities. I suggest that the authors concentrate on individual mortality probabilities in both approaches.
The only case where I find the focus on mortality rates helpful is when comparing observed mortality rates against the proxies of physiological stress. I would see this as a rough test that the model can reflect those stresses that are relevant for observed mortality.
Using mechanistic models to disentangle the drivers of mortality
The authors state that they aim to “decipher the respective roles of the drivers and mechanisms underlying tree mortality and understand their variability among individuals or years” (see discussion). To this end, they apply the process-based model CASTANEA and analyze three stress indicators: percentage of loss of conductance (PLC), biomass of reserve and number of late frost days. To test if these stress indicators are more or less realistic, they correlate them against the mortality rate. I think this should be okay, but the authors could do better in explaining their approach, the underlying assumptions and discussing its limitations.
Specifically, I am missing some critical notes on the limited number of mortality causes that are implemented in process-based models in the introduction and discussion. Using models to identify drivers of tree mortality can only reveal those processes that have been implemented in the models, while processes that are missing, e.g. competition and pathogens, cannot be reflected. The authors should also comment on how reliable the model parameterization is, i.e. how much they trust the simulation results.
I am not very familiar with the details of CASTANEA. From how the authors set up the analyses of the model simulations, I thought CASTANEA doesn’t explicitly simulate tree death, but physiological indicators only. In Line 562 however, the authors mention that CASTANEA cannot simulate “background mortality”. Maybe this refers to the inability to account for stresses due to resource competition? If CASTANEA also provides mortality probabilities, I think it would be great to make a similar individual-based mortality model for the model output, to compare how mortality is driven in the model compared to the observations.
Specific comments
Line 28: Consider rephrasing: “an exceptional annual monitoring of 4327 individual European beech trees”. Is it annual or every 6 months?
Lines 29ff: Consider rephrasing: “We combined two types of approaches to analyze beech mortality: (1)…, and (2)…”
Line 36: “In the statistical models”
Lines 38-39: Consider rephrasing: “The interaction between tree size and defoliation was significant, indicating a stronger increase in mortality probability due to defoliation for small than for tall trees.”
Line 40: Unclear what “earlier” trees are.
Lines 75-76: Consider rephrasing: “which can eventually become depleted, particularly…”.
Line 97: Remove second time “reflush”.
Lines 108ff: Typically, empirical models are not for mortality rates (also not those cited here), but for mortality probabilities. Please correct.
Lines 118ff: I don’t really understand, why non-linearity is a problem, at least not as an inherent problem of empirical mortality models. Empirical datasets from inventories are typically quite large and allow testing rather complex functional forms including non-linear relationships. It is true that in some studies this possibility is not sufficiently explored, but in my opinion, this is not really a disadvantage of empirical mortality models. There are other more important limitations: low temporal resolution of data, reason of death is often not known (btw: is information on this recorded for the site used in this study?).
Lines 128ff: The next two sentences sound contradictory. I get the point, but the phrasing can probably be improved.
Lines 132ff: To what kind of individual effects to you refer here? Be more precise.
Line 139: Maybe it would be helpful for the non-European reader to specify where the rear-edges of beech are?
Line 142: Consider rephrasing of the “have been associated in beech to decreased growth”.
Line 147: Remove “of”.
Line 148: I really liked your introduction of Fagus sylvatica and why it is interesting to study it!
Line 151: You could mention the location of the site here already.
Lines 163-164: “South of beech distribution area” sounds a bit strange.
Line 166: Are the temperature values absolute, daily or monthly minima/maxima?
Line 170: “removed”
Lines 173ff: At which spatial resolution were these additional variables recorded?
Lines 190: Consider renaming to “DBH2002”.
Line 194: There may be several problems here. I think the equation is not correct. First, the radius should be squared. Second, relative basal area is typically a relative measure with respect to the basal area at t = 0: BA1-BA0 / BA0. The equation here is only annual basal area increment. Finally, how do you deal with trees that died between 2002 and 2012? DBH measurements of dead trees are very unreliable, e.g. because the bark can fall off.
Line 205: I don’t understand the meaning of “ratio ordered” in this context.
Line 219: Consider rephrasing “We computed this competition index in three ways:”.
Line 220: Same “coppice” or same “species”? It may be helpful to introduce the idea and your definition of “same coppice” before using such definitions.
Line 226: Do you mean the “indices” or the “estimated slopes” reached a plateau?
Table 1: Why are the values for COMPETintra+ smaller than those of COMPETintra?
Lines 234-235: Consider rephrasing “… since 1976 and 1960 for temperature and precipitation/mean relative humidity, respectively”.
Lines 242ff: Check sentence structure.
Lines 244-245: Does the number of days with negative temperature occurring after beech budburst account for the budburst date of individual trees?
Line 262: Remove second “is”.
Line 265: I guess you mean predictors not factors.
Line 275: What do you mean with qualitative explanatory variables? Categorical variables? I find this terminology a bit uncommon.
Lines 294-296: True, but then you wouldn’t do model selection at all.
Line 297: I think that odd ratios can only be compared if the continuous predictors in the model are scaled.
Line 324: I am missing a sentence on the aim of using CASTANEA including which information is reported from the models and why. Or this should be outlined in “Simulation design”.
Lines 330ff: Check the suitability of the verbs used. Estimate, calculate, compute and simulate don’t mean the same thing… I would also start with the equation of PLC and then explain its components. Starting with leaf water potential after the sentence on PLC and defoliation seems not intuitive.
Line 349: What do you mean with “stresses without a priori”?
Lines 356-357 and 359: Consider rephrasing “randomly drawn from a normal distribution” and “randomly drawn from a uniform distribution”.
Line 367: Reference to Fig. 1 would be helpful.
Line 371: It is Table 2 not 1.
Lines 373ff: Why should the effects of these predictors only apply to some years?
Lines 385ff: The results you present here are not only at the population level. You also report the range of individual PLC estimates. I think this is no problem, but it indicates that your differentiation between individual and population is not ideal.
Line 385: If you talk about an increase, you should mention from which level PLC has increased. The same for carbon reserves and late frost days in Line 388 and 391.
Lines 393-394: Consider rephrasing to “with 5 to 9 days and 5 to 7 days of late frost, respectively”.
Line 396: Do you correct for temporal autocorrelation in the test?
Line 408: Significance cannot be interpreted when model selection was carried out beforehand (either automatic or manual).
Line 410: “dead individuals truly observed dead” sounds like the true positive rate. If this is the case, you should also report the true negative rate. If this is variance explained, your explanation in brackets is maybe not fully correct.
Line 412: Check again if continuous predictors were scaled.
Lines 426: It is not the interaction that is true for low mean growth, but the difference in mortality probability between small and large trees was only evident for low mean growth.
Lines 437-441: It is unclear to which results you refer here. A reference to a figure or table is missing.
Figure 3: The combination of color, symbol and filling is very confusing. Reduce degree of information or make more subpanels for key comparisons. You may also replace years with “severe drought”, “no stress” and “late frost”.
Lines 475-476: It is not surprising that a lot of variation is explained because the model assumptions are violated (temporal autocorrelation) and the number of observations is very small. In addition, the dataset is very homogenous compared to Greenwood et al.
Line 480: Mortality rates always need to be reported in the context of the dbh threshold, because if more small trees are included the mortality probability will likely increase. This makes comparisons of rates with different dbh thresholds very difficult. You should account for this when comparing your mortality rates with previous publications.
Line 497: Please specify what you mean with positive interaction.
Lines 506ff: Carbon starvation and hydraulic failure are typically debated in the context of drought. It is not clear how frost goes in here… You may reconsider the wording of “stresses vulnerability”.
Line 512: Why couldn’t you account for the temporal dynamics across years?
Line 521: Be specific about which approach revealed a result. “Confirmed” is actually very strong. We know that empirical studies often don’t replicate.
Lines 519ff: I am missing some ecological explanations here.
Line 534: When talking about an effect, it is helpful to always mention on what the effect takes place (here mortality I guess).
Line 545: Use “individuals with early budburst” instead of “early individuals” throughout the whole manuscript.
Line 546: Vulnerable to what?
Line 575: You may exchange “interest” with “potential”.
Lines 581ff: To me, this complementarity is still vague. How do you suggest both approaches should be combined? Doing both and then compare results? Or something more integrative?
Lines 591-592: The results of the population-level empirical model are not at all reliable. I am still wondering, how you can test the effect of early budburst in the population level model?