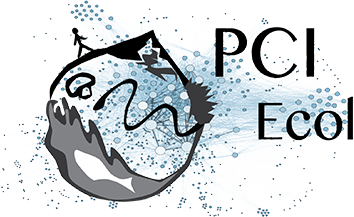
Spatial patterns and autocorrelation challenges in ecological conservation
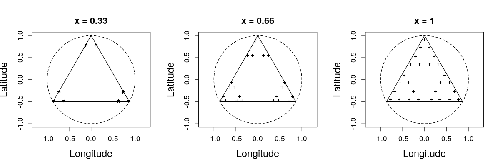
Efficient sampling designs to assess biodiversity spatial autocorrelation : should we go fractal?
Abstract
Recommendation: posted 02 January 2024, validated 03 January 2024
Goberville, E. (2024) Spatial patterns and autocorrelation challenges in ecological conservation. Peer Community in Ecology, 100536. https://doi.org/10.24072/pci.ecology.100536
Recommendation
“Pattern, like beauty, is to some extent in the eye of the beholder” (Grant 1977 in Wiens, 1989)
The recommender in charge of the evaluation of the article and the reviewers declared that they have no conflict of interest (as defined in the code of conduct of PCI) with the authors or with the content of the article. The authors declared that they comply with the PCI rule of having no financial conflicts of interest in relation to the content of the article.
Agence Nationale de la Recherche (ANR), grant n° 19-CE32-0002-01
Evaluation round #2
DOI or URL of the preprint: https://doi.org/10.1101/2022.07.29.501974
Version of the preprint: 3
Author's Reply, 01 Dec 2023
Decision by Eric Goberville
, posted 05 Nov 2023, validated 06 Nov 2023
Dear Dr. Laroche,
Thank you for your submission entitled "Efficient sampling designs to assess biodiversity spatial autocorrelation: should we go fractal?" to PCIEcology. We have received feedback from the two referees, and I would like to express my appreciation for their comprehensive and insightful reviews.
Both reviewers commend your meticulous work in addressing their previous comments and recommendations. They highlight significant improvements, including the transformation of complex code into an informative Rmarkdown document and the innovative approach to defining 'rugged' environmental variables. However, there are some minor suggestions related to code structure, conditional chunk evaluation, and the need for clearer figure annotations.
Furthermore, they note limitations in the example visualizations, which could be expanded to cover a broader range of 'as' values. The importance of specifying the grid mesh size is also emphasized. The 'Spanning path length' analysis, while valuable, is seen as somewhat of an afterthought and should be included in the methods section for better contextual understanding.
One reviewer underscores the absence of essential information in the methods section, particularly regarding repetitions and averaging, urging greater clarity in this regard. They also recommend distinguishing between regular grid and random data points in hybrid models and using subscript in plot titles for figures 4 and 6. Figure 10 is seen as somewhat unclear. The suggestion is made to define the ratio of sampling area to the number of sampling points for better interpretation. Additionally, a few minor points are addressed.
In conclusion, the requested revisions represent a relatively modest effort compared to the substantial improvements you have already made to your study. I believe that these adjustments will contribute to the final acceptance of your article in PCI in Ecology.
Sincerely,
Eric Goberville
Reviewed by Nigel Yoccoz
, 01 Nov 2023
The author has carefully revised the paper, adding much relevant information and additional simulations. I look forward to applications of some of the ideas developed in the paper, and the scripts provide the necessary tools.
https://doi.org/10.24072/pci.ecology.100536.rev21Reviewed by Charles J Marsh
, 04 Nov 2023
Review for Laroche - Efficient sampling designs to assess biodiversity spatial autocorrelation: should we go fractal?
The as values span from 0.01 – 100, but the examples of as in figs 4 and 6 are really limited showing only 0.09 – 0.33 (ranks 7-12 out of 28 total). This also doesn’t include the grid mesh size which is the ‘switching point’ of behaviour (which I believe should be 0.38). When I have created the figures with a wider range of values it produces some interesting patterns not apparent from the current figures, and also makes much more sense of figure 8.
Evaluation round #1
DOI or URL of the preprint: https://doi.org/10.1101/2022.07.29.501974
Version of the preprint: 2
Author's Reply, 09 Oct 2023
Decision by Eric Goberville
, posted 15 Jul 2023, validated 17 Jul 2023
Dear Dr. Laroche,
Thank you for submitting your article titled "Efficient sampling designs to assess biodiversity spatial autocorrelation: should we go fractal?" to PCIEcology. We have now received feedback from two referees, and I would like to express my gratitude to both referees for their thorough and insightful review of your manuscript. I share their opinion regarding the relevance of your study and its significant contribution to the existing literature, as well as the importance of providing access to the code for ensuring reproducibility of the analyses. However, before your study can be considered for publication, some revisions and clarifications are necessary.
The first referee noted that your article addresses a pertinent subject but highlighted a lack of references to recent research in the field. It is strongly recommended to include credible sources to support your arguments and enhance the credibility of your article. Additionally, the referee encourages you to delve deeper into specific aspects of your analysis by providing concrete examples or case studies to substantiate your viewpoints.
The second referee acknowledges the commendable aspects of the article, including the methodology of defining hybrid and fractal patterns and the use of the Pareto front method to examine trade-offs. Overall, the referee provides valuable feedback regarding the need to consider multiple variables/species, the practicality of sampling designs, and the choice of environmental variables. Further exploration of these aspects is encouraged to improve the practical applicability of the study.
We kindly request that you take these comments and suggestions into consideration during the revision of your article. Please submit a revised version of your manuscript, incorporating the referees' remarks. Additionally, we would appreciate a detailed response letter addressing how you have addressed the referees' comments.
Thank you for your valuable contribution to our scientific journal, and we look forward to receiving your response.
Best regards,
Eric Goberville
Reviewed by Nigel Yoccoz
, 27 Jun 2023
Despite the recognized importance of sampling design, at least for researchers with an interest in statistical questions, it is remarkable that so few empirical studies in ecology are in fact designed according to well-defined objectives and some forms of random or systematic sampling. If one takes the example of species distributions, most studies use “available” data which are most often derived from opportunistic sampling or some form of hybrid designs (e.g. random design initially but with some nonrandom selection of final units linked for example to accessibility or observer availability). Many approaches have then been developed to account for this lack of design, but their robustness is often unclear. Clearly it would be preferable to start with a good sampling design.
This paper investigates different designs – random, grid, fractal (multiple scales) – and their efficiency when autocorrelation can be seen either as a “nuisance” and a parameter of interest. It is based on extensive simulations, and using a model-based approach for estimation. The conclusions are that fractal designs are seldom efficient. The scripts for running the simulations are available, but I did not run the simulations to check the results.
This is an interesting contribution for researchers working on sampling design, as it explicitly addresses different objectives (i.e. not “just” estimating population size, or an environmental effect). I could add that a specific difficulty with autocorrelation from a statistical point of view is that it may be hard to distinguish between a “real” autocorrelation due for example to intrinsic processes such as dispersal and the effect of a spatial covariate having an autocorrelation with the same range (i.e. it is not just an issue of bias but also of identifiability). As one often does not know what are the effects and range of environmental covariates, it is not obvious how sampling should be done. This paper addresses some of the issues associated with autocorrelation and estimating effects of covariates, and perhaps the author should emphasize the importance of making simulations to assess different designs depending on study objectives. Simulations are useful not just for assessing different design as is well done in this paper, but also because it forces the researchers to specify objectives, both in terms of ecological questions and in terms if what can be realistically expected in terms of precision/bias.
https://doi.org/10.24072/pci.ecology.100536.rev11