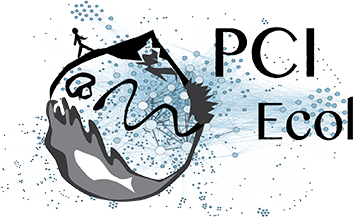
Species Distribution Models: the delicate balance between signal and noise
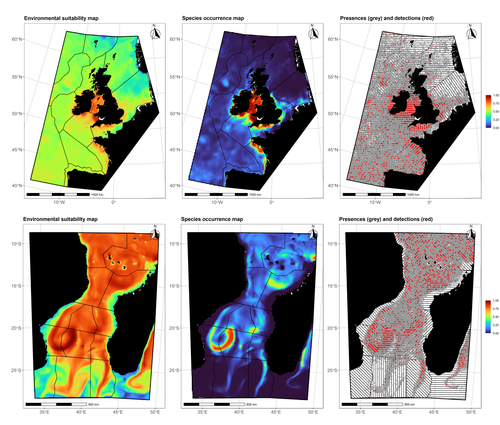
Data stochasticity and model parametrisation impact the performance of species distribution models: insights from a simulation study
Abstract
Recommendation: posted 04 April 2023, validated 04 April 2023
Poisot, T. (2023) Species Distribution Models: the delicate balance between signal and noise. Peer Community in Ecology, 100523. 10.24072/pci.ecology.100523
Recommendation
Species Distribution Models (SDMs) are one of the most commonly used tools to predict where species are, where they may be in the future, and, at times, what are the variables driving this prediction. As such, applying an SDM to a dataset is akin to making a bet: that the known occurrence data are informative, that the resolution of predictors is adequate vis-à-vis the scale at which their impact is expressed, and that the model will adequately capture the shape of the relationships between predictors and predicted occurrence.
In this contribution, Lambert & Virgili (2023) perform a comprehensive assessment of different sources of complications to this process, using replicated simulations of two synthetic species. Their experimental process is interesting, in that both the data generation and the data analysis stick very close to what would happen in "real life". The use of synthetic species is particularly relevant to the assessment of SDM robustness, as they enable the design of species for which the shape of the relationship is given: in short, we know what the model should capture, and can evaluate the model performance against a ground truth that lacks uncertainty.
Any simulation study is limited by the assumptions established by the investigators; when it comes to spatial data, the "shape" of the landscape, both in terms of auto-correlation and in where the predictors are available. Lambert & Virgili (2023) nicely circumvent these issues by simulating synthetic species against the empirical distribution of predictors; in other words, the species are synthetic, but the environment for which the prediction is made is real. This is an important step forward when compared to the use of e.g. neutral landscapes (With 1997), which can have statistical properties that are not representative of natural landscapes (see e.g. Halley et al., 2004).
A striking point in the study by Lambert & Virgili (2023) is that they reveal a deep, indeed deeper than expected, stochasticity in SDMs; whether this is true in all models remains an open question, but does not invalidate their recommendation to the community: the interpretation of outcomes is a delicate exercise, especially because measures that inform on the goodness of the model fit do not capture the predictive quality of the model outputs. This preprint is both a call to more caution, and a call to more curiosity about the complex behavior of SDMs, while also providing a sensible template to perform future analyses of the potential issues with predictive models.
References
Halley, J. M., et al. (2004) “Uses and Abuses of Fractal Methodology in Ecology: Fractal Methodology in Ecology.” Ecology Letters, vol. 7, no. 3, pp. 254–71. https://doi.org/10.1111/j.1461-0248.2004.00568.x.
Lambert, Charlotte, and Auriane Virgili (2023). Data Stochasticity and Model Parametrisation Impact the Performance of Species Distribution Models: Insights from a Simulation Study. bioRxiv, ver. 2 peer-reviewed and recommended by Peer Community in Ecology. https://doi.org/10.1101/2023.01.17.524386
With, Kimberly A. (1997) “The Application of Neutral Landscape Models in Conservation Biology. Aplicacion de Modelos de Paisaje Neutros En La Biologia de La Conservacion.” Conservation Biology, vol. 11, no. 5, pp. 1069–80. https://doi.org/10.1046/j.1523-1739.1997.96210.x.
The recommender in charge of the evaluation of the article and the reviewers declared that they have no conflict of interest (as defined in the code of conduct of PCI) with the authors or with the content of the article. The authors declared that they comply with the PCI rule of having no financial conflicts of interest in relation to the content of the article.
The authors declare that they have received no specific funding for this study
Evaluation round #1
DOI or URL of the preprint: https://doi.org/10.1101/2023.01.17.524386
Version of the preprint: 1
Author's Reply, 21 Mar 2023
Decision by Timothée Poisot
, posted 12 Mar 2023, validated 12 Mar 2023
Dear authors,
I have received two reviews on your preprint, both of which are positive about the work, and emphasize that the text of the preprint is clear despite the complexity inherent to presenting the results of many models and complex analyses.
Both reviewers offer suggestions (on framing, additional concepts and associated literature) and corrections that will improve the quality of the preprint. These are VERY minor revisions, and I do not anticipate needing to send it for review after the revisions are made.
Montréal, March 12, 2023
Timothée Poisot
Reviewed by Alejandra Zarzo Arias, 02 Mar 2023
This manuscript explores the differences when building Species Distribution Models for two virtual species using 5 different spatial resolutions of the environmental conditions, and with two different sampling types.
I enjoyed the manuscript a lot, with so many different models and comparisons it is often hard to organize the results, but they appear clear and well sumarized. I think the whole manuscript is well written and the ideas are clear, thus, I only have some minor comments:
First of all, I recommend avoiding green+red combination in maps and figures, as color-blind people often cannot distinguish them (e.g., Figure 3, 9, S1-4).
I think a figure explaining the different sampling methods (segment-based vs. areal-based) would be nice to fully understand the methods.
Line 4 Abstract: change "wide array OF environmental conditions"
Line 14: "outputS"
Line 48: "distanT"
Line 49: not a single citation in the entire paragraph. You can give examples of studies using those methodologies.
Line 52: "process" not "processed"
Line 171: Paragprah "Single variable approach": I believe a further explanation of parameters and distribution of the residuals would be needed for the reader to fully understand model construction.
Line 172: the reference should explain GAM models, I think you mean Wood, 2006.
Line 226: remove A in "highlighted A large"
Line 454: remove WAS in "As was observed"
Line 503: "at which the model will be built"
For the take home message paragraph I would include more details on what information could be useful and the maximum change in scale admissible to trust some of the results when applying models to the real life (i.e., environmental variables response, suitability maps), which represent very important information for example for conservation purposes, or to select new potential sampling sites.
Finally, in the main results Figures (also in Supplementary Figures), consider including the original map/graph for the "real" original non-modified models so it makes it easier to compare the results visually. For example, a black curve of the original response in the variable curves graphs.
I would recommend the authors to check publications from Vítězslav Moudrý's research group, I believe they will find them interesting.
Reviewed by anonymous reviewer 1, 09 Feb 2023
This is an interesting and mostly well-executed work. The authors create "virtual species" by defining what is essentially a fundamental niche. They then use a Poisson point-process to generate presences in the region of choice. Their "virtual species" are defined only by their abiotic niche (i.e., biotic interactions and dispersal are disregarded), which is not too much of a problem, although it would be good to be explicit about this: the authors are modeling niches and applying them to geography, rather than modeling the actual biology of a species (including interactions, and movements). Finally, they sample, realistically over the universe of points created by projecting favorable conditions, and proceed to do ecological niche modeling using GAMs, and AIC for goodness of fit. They compare modeling results for different sampling methods, resolutions etc. The results are clearly presented and explained.
I wish the authors refrain from refering to what are projections of niches in geography as "Species Distribution Models" since in reality they are *potential* distribution models, but this is mostly a personal preference, since in the literature the distinction between a *potential* and an *actual* distribution is seldom made. But this is a "virtual species" paper, and one can only imagine that their species has unlimited dispersal capabilities and no significan biotic limitations. Maybe the authors can state this explicitly in the introduction.
I think the preprint should be recommended, despite the fact that, as with many similar attempts, the complexity of the analysis is great, and one is always left with the question of whether there are general lessons, or the results are artifactual, and how general they are. Nevertheless, the work deserves publication if only because of the stress in the importance of scale of the variables. I believe the authors are basically illustrating that the "Modifiable Areal Unit" problem, that has bothered geographers for the last hundred years, matters when modeling niche-based distributions (see Jelinski DE, Wu J. 1996, The modifiable areal unit problem and implications for landscape ecology. Landscape ecology.11:129-40).