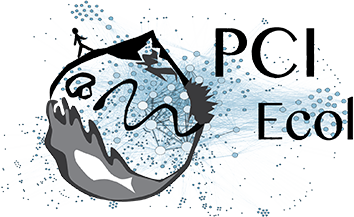
Uncertain predictions of species responses to perturbations lead to underestimate changes at ecosystem level in diverse systems
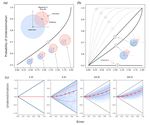
Why scaling up uncertain predictions to higher levels of organisation will underestimate change
Abstract
Recommendation: posted 19 October 2020, validated 21 October 2020
Thebault, E. (2020) Uncertain predictions of species responses to perturbations lead to underestimate changes at ecosystem level in diverse systems. Peer Community in Ecology, 100063. 10.24072/pci.ecology.100063
Recommendation
Different sources of uncertainty are known to affect our ability to predict ecological dynamics (Petchey et al. 2015). However, the consequences of uncertainty on prediction biases have been less investigated, especially when predictions are scaled up to higher levels of organisation as is commonly done in ecology for instance. The study of Orr et al. (2020) addresses this issue. It shows that, in complex systems, the uncertainty of unbiased predictions at a lower level of organisation (e.g. species level) leads to a bias towards underestimation of change at higher level of organisation (e.g. ecosystem level). This bias is strengthened by larger uncertainty and by higher dimensionality of the system.
This general result has large implications for many fields of science, from economics to energy supply or demography. In ecology, as discussed in this study, these results imply that the uncertainty of predictions of species’ change increases the probability of underestimation of changes of diversity and stability at community and ecosystem levels, especially when species richness is high. The uncertainty of predictions of species’ change also increases the probability of underestimation of change when multiple ecosystem functions are considered at once, or when the combined effect of multiple stressors is considered.
The consequences of species diversity on ecosystem functions and stability have received considerable attention during the last decades (e.g. Cardinale et al. 2012, Kéfi et al. 2019). However, since the bias towards underestimation of change increases with species diversity, future studies will need to investigate how the general statistical effect outlined by Orr et al. might affect our understanding of the well-known relationships between species diversity and ecosystem functioning and stability in response to perturbations.
References
Cardinale BJ, Duffy JE, Gonzalez A, Hooper DU, Perrings C, Venail P, Narwani A, Mace GM, Tilman D, Wardle DA, Kinzig AP, Daily GC, Loreau M, Grace JB, Larigauderie A, Srivastava DS, Naeem S (2012) Biodiversity loss and its impact on humanity. Nature, 486, 59–67. https://doi.org/10.1038/nature11148
Kéfi S, Domínguez‐García V, Donohue I, Fontaine C, Thébault E, Dakos V (2019) Advancing our understanding of ecological stability. Ecology Letters, 22, 1349–1356. https://doi.org/10.1111/ele.13340
Orr JA, Piggott JJ, Jackson A, Arnoldi J-F (2020) Why scaling up uncertain predictions to higher levels of organisation will underestimate change. bioRxiv, 2020.05.26.117200. https://doi.org/10.1101/2020.05.26.117200
Petchey OL, Pontarp M, Massie TM, Kéfi S, Ozgul A, Weilenmann M, Palamara GM, Altermatt F, Matthews B, Levine JM, Childs DZ, McGill BJ, Schaepman ME, Schmid B, Spaak P, Beckerman AP, Pennekamp F, Pearse IS (2015) The ecological forecast horizon, and examples of its uses and determinants. Ecology Letters, 18, 597–611. https://doi.org/10.1111/ele.12443
The recommender in charge of the evaluation of the article and the reviewers declared that they have no conflict of interest (as defined in the code of conduct of PCI) with the authors or with the content of the article. The authors declared that they comply with the PCI rule of having no financial conflicts of interest in relation to the content of the article.
Reviewed by anonymous reviewer 1, 07 Oct 2020
My comments and issues have been solved. I think the manuscript has been improved and I am happy to recommend it.
Evaluation round #1
DOI or URL of the preprint: 10.1101/2020.05.26.117200
Version of the preprint: 1
Author's Reply, 23 Sep 2020
Dear Recommender,
We thank you and the reviewers for their thorough and helpful comments.
We have now addressed all your points and those of the two reviewers. These modifications are summarised in the attached response letter, and can also be seen in the attached pdf of the modified manuscript, where we tracked all changes made.
The new manuscript has been uploaded to bioRxiv https://doi.org/10.1101/2020.05.26.117200 and line numbers in our response letter refer to that manuscript.
We hope that you will find this new version acceptable. Best regards,
Jean-Francois Arnoldi
Decision by Elisa Thebault, posted 24 Aug 2020
Dear authors,
I have now received two reviews of your manuscript. Both reviewers and I are in agreement that this is an interesting study considering how scaling up uncertain predictions of individual properties in complex systems affects the estimation of system-level properties. The results have important implications in ecology as well as in other research disciplines. However, several issues have been identified which, in my views, require revision before recommendation. Such revised contribution would need to address all of the reviewer comments. In particular, reviewer #1 raises an issue regarding the assumptions on the specific distribution of the “error” used in the mathematical derivation. In addition, reviewer #2 highlights several points that would deserve to be further clarified and discussed (e.g. further discussion of the implications of the results for other research areas, including consequences of intraspecific variations).
In addition to the comments of the reviewers, I have a few additional suggestions to help improve the clarity of the manuscript:
Figure 2: When reading first the manuscript, I didn’t understand the meaning of the blue and red circles in this figure, and globally this figure is rather difficult to understand. This part only becomes clear when reading the next section with Figure 3. I would suggest either removing this figure, or simplifying it by summarizing more the main steps and goals of the approach taken in the manuscript (as an illustration for the end of the introduction).
Box 1 is very useful but it is cited only rarely in the text. I think further reference to this box would be very helpful to remind readers of critical steps and definitions of the approach (e.g. how change is measured at the system level in the geometrical approach).
Legend of Figure 3: in (c), please explain what corresponds to x and y in the equation and what it means (i.e. expected relationship between error and underestimation as derived from equation 4). From what I understood, the dashed red lines and the black points correspond to (mean – sd) and (mean + sd) and not to the values of the variances. This needs to be clarified. In addition, I would also explain that “underestimation” refers to the relative magnitude of underestimation as defined in equation (2).
Legend of Figure 4, “The variance around the mean expectation was accurately predicted using the IPR instead of species richness”: I would explain why more clearly in the text. Indeed, if the variance around the mean expectation was well predicted by species richness, we would have the same variance in the two studied cases of biomass distribution as they have the same number of species.
Line 442 page 21: “we still see below”
Line 470 page 23: “probability of underestimation” instead of “probability of synergism”
Examples page 25: it is not fully clear how these examples are related to what is presented in the main text, this would need to be clarified. More globally, I think the appendices could be linked a little more clearly to the main text.
Appendix page 29: This is not fully clear how the different aggregate functions are defined here. For instance, do they depend on species biomass or on other species properties? This point would deserve to be explained in the main text too.
I am looking forward to seeing your revised manuscript addressing the reviewers’ comments, along with a point-by-point response.
Best wishes, Elisa Thébault
Reviewed by anonymous reviewer 1, 20 Jul 2020
Review of “Why scaling up uncertain predictions to higher levels of organisation will underestimate change” by James Orr, Jeremy Piggott, Andrew Jackson, and Jean-François Arnoldi
In their manuscript, the authors argue that scaling up individual properties of complex systems to a system-level properties will necessarily result in an underestimation. The authors show that this effect is dimension dependent and they argue that in general the dimension should be computed as the inverse participation ratio. It is a well-written manuscript and, especially, I find the geometric approach very intuitive. I think this result deserves a recommendation in PCI.
I have one major comment that the authors should first address. I do not think that the result applies to any type of “error”; they should be an implicate assumption about the “error” that the authors have to make explicit. I arrive to this conclusion as in general in probability theory we cannot switch between taking the expectation of a random variable and an arbitrary function, i.e. in general f (E(x)) is not equal to E (f(x)). For example, let us assume x ~ Uniform distribution between -1 and +1 and f(x) = x^2. Clearly, E(x) =0, but E (x^2) > 0 = (E(x))^2. So equations (4), (5), (7) and the mathematical derivation in the appendix work only for specific assumptions on the distribution of the “error”. Stated as it is, they are simply wrong. The authors have to find under which assumption their mathematical derivation works and make it explicit in their manuscript. I guess the assumption is an independence assumptions between the “error” between, i.e., they may have to be i.i.d. distributed. I also find the authors should provide more mathematical reference justify their mathematical derivation.
Reviewed by Carlos Melian, 21 Aug 2020
Authors develop a framework to quantify the underestimation of the magnitude of a system level change when scaling up from species-level to ecosystem function (i.e. aggregated biomass). Authors argue that underestimation -- and uncertainty -- grow with the system dimensionality, with dimensionality not meaning more constituent species, but more diversity (i.e., diversity metrics like the inverse participation ratio or Hill numbers) -- The explanation from authors is based on the geometric observation that in high dimensions there are more ways to be more different, than ways to be more similar. Authors provide a linear and nonlinear approx. to proof this statement. They go deeper to explain that nonlinearility controls the sensitivity to underestimate upscaled predictions. Authors make a connection to stressed ecosystems: there will be bias towards synergism when multiple stressors predictions are scaled up to higher levels of organization.
Authors apply underestimation at two levels in ecology -- biomass to diversity. Could the message be that the higher the dimensionality, for example from species to intraspecific or even to intraorganismal level for large populations or communities, the higher the underestimation of system change at these high dimensionality levels? What do authors think about generalizing (or discussing) their method to any number of levels? As authors notice in Box 5, this topic is important in many disciplines. I is relevant to any field of science that contains two or more levels each containing variance, and variance at intraorganismal and intraspecific levels might contain additional dimensions, like the number of traits or trait arquitecture of individuals. Overall, with the increasing resolution of data in ecology, usually containing individual level data, accounting for uncertainty to quantify the bias in the mean field approaches is key, especially in the context of management of large ecosystems. Authors should emphasize more how the nature of ecological and other's disciplines data is challenging our understanding of uncertainty when accounting not only for 2 but for many levels. This relates to Box 2 -- All these disciplines contain individuals varying in phenotypes, strategies and so on. Yet these heterogeneities within species are just ignored across disciplines. Are authors assuming all diversity metrics are based in mean field phenotypic distributions containing low variance? Why is this so?
Comments
Authors refer to "multidimensional system" to a system containing a species-rich ecosystem -- do authors implicitly assume that each species increases ecosystem dimension in one? Why? Does this imply that all species living in a species-rich ecosystem make a perfect partition of one dimension per species? Please clarify.
Authors emphasize their method predicts the generation towards non-additive synergism -- They use a geometric method to proof this statement yet the processes underlying diversity metrics can be different while the diversity metrics per se might remain similar -- For example -- rapid negative frequency dependent resource selection increases fitness of rare types, increasing the number of coexisting types within a species. This mechanism also balance species abundances and increases diversity. The mechanism of positive frequency dependent resource selection has the opposite impact, reducing intraspecific diversity while the mean types and the diversity metrics can be the same than in the previous case. How do authors think two opposite processes at intraspecific level change dimensionality (and uncertainty) at the diversity metrics level? Do these two processes provide alternative bias towards synergism and antagonism? For example, can this be tested exploring two different selection regimes using the simulated communities of Fig 5? Please clarify.