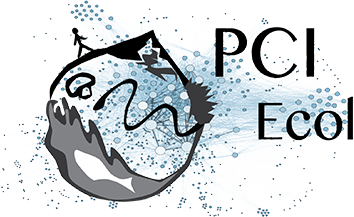
On the importance of stoichiometric constraints for understanding global change effects on food web dynamics

Stoichiometric constraints modulate the effects of temperature and nutrients on biomass distribution and community stability
Abstract
Recommendation: posted 22 January 2020, validated 29 January 2020
Thebault, E. (2020) On the importance of stoichiometric constraints for understanding global change effects on food web dynamics. Peer Community in Ecology, 100039. https://doi.org/10.24072/pci.ecology.100039
Recommendation
The constraints associated with the mass balance of chemical elements (i.e. stoichiometric constraints) are critical to our understanding of ecological interactions, as outlined by the ecological stoichiometry theory [1]. Species in ecosystems differ in their elemental composition as well as in their level of elemental homeostasis [2], which can determine the outcome of interactions such as herbivory or decomposition on species coexistence and ecosystem functioning [3, 4].
Despite their importance, stoichiometric constraints are still often ignored in theoretical studies exploring the consequences of environmental perturbations on food web stability. Meanwhile, drivers of global change strongly alter biochemical cycles and the balance of chemical elements in ecosystems [5]. An important challenge is thus to understand how stoichiometric constraints affect food web responses to global changes.
The study of Sentis et al. [6] makes a step in that direction. This article investigates how stoichiometric constraints affect the response of consumer-resource dynamics to increasing temperature and nutrient inputs. It shows that the stoichiometric flexibility of the resource, coupled with lower consumer assimilation efficiency when stoichiometric unbalance between the resource and the consumer is higher, dampens the destabilizing effects of nutrient enrichment on species dynamics but reduces consumer persistence at extreme temperatures. Interestingly, these effects of stoichiometric constraints arise not only from changes in species assimilation efficiencies and carrying capacities but also from stoichiometric negative feedback loops on resource and consumer populations.
The results of this study are a call to further include stoichiometric constraints into food web models to better understand and predict the consequences of global changes on ecological communities. Many perspectives exist on that issue. For instance, it would be interesting to assess the effects of other stoichiometric mechanisms (e.g. changes in the element limiting growth [3]) on food web stability and its response to nutrient enrichment, as well as the effects of other global change drivers associated with altered biochemical cycles (e.g. carbon dioxide increase).
References
[1] Sterner, R. W. and Elser, J. J. (2017). Ecological Stoichiometry, The Biology of Elements from Molecules to the Biosphere. doi: 10.1515/9781400885695
[2] Elser, J. J., Sterner, R. W., Gorokhova, E., Fagan, W. F., Markow, T. A., Cotner, J. B., Harrison, J.F., Hobbie, S.E., Odell, G.M., Weider, L. W. (2000). Biological stoichiometry from genes to ecosystems. Ecology Letters, 3(6), 540–550. doi: 10.1111/j.1461-0248.2000.00185.x
[3] Daufresne, T., and Loreau, M. (2001). Plant–herbivore interactions and ecological stoichiometry: when do herbivores determine plant nutrient limitation? Ecology Letters, 4(3), 196–206. doi: 10.1046/j.1461-0248.2001.00210.x
[4] Zou, K., Thébault, E., Lacroix, G., and Barot, S. (2016). Interactions between the green and brown food web determine ecosystem functioning. Functional Ecology, 30(8), 1454–1465. doi: 10.1111/1365-2435.12626
[5] Peñuelas, J., Poulter, B., Sardans, J., Ciais, P., van der Velde, M., Bopp, L., Boucher, O., Godderis, Y., Hinsinger, P., Llusia, J., Nardin, E., Vicca, S., Obersteiner, M., Janssens, I. A. (2013). Human-induced nitrogen–phosphorus imbalances alter natural and managed ecosystems across the globe. Nature Communications, 4(1), 1–10. doi: 10.1038/ncomms3934
[6] Sentis, A., Haegeman, B. & Montoya, J.M. (2020). Stoichiometric constraints modulate the effects of temperature and nutrients on biomass distribution and community stability. bioRxiv, 589895, ver. 7 peer-reviewed and recommended by PCI Ecology. doi: 10.1101/589895
The recommender in charge of the evaluation of the article and the reviewers declared that they have no conflict of interest (as defined in the code of conduct of PCI) with the authors or with the content of the article. The authors declared that they comply with the PCI rule of having no financial conflicts of interest in relation to the content of the article.
no declaration
Evaluation round #2
DOI or URL of the preprint: https://doi.org/10.1101/589895
Version of the preprint: 2
Author's Reply, 10 Jan 2020
Decision by Elisa Thebault, posted 02 Jan 2020
Dear authors,
Many thanks for your thorough revision of the manuscript. There are only a few remaining minor comments to address from one of the reviewers before recommendation.
best wishes, Elisa
Reviewed by anonymous reviewer 2, 18 Dec 2019
Sentis et al. Revised Version #1
I enjoyed reading the revised version of this manuscript from Sentis et al. I was impressed by the authors response to my queries. I have two more important points that I hope will address my remaining concerns along with some extra line comments. My two main concerns are (#1) the extinction threshold interpretation and the (#2) the assumption of e^ns = e_max. I've really enjoyed reviewing this paper--the authors are exploring an important and exciting topic in my opinion.
Line Comments:
Line 34-35: Can you state the direction of the effect rather than referring to a generic change?
Line 42-160: I would argue for a further reduction in the text here to focus in on the key mechanisms your model addresses. For example, you discuss (e.g. Line 97, 116) drivers and effects that you model is not currently considering. This is my opinion, but not a binding request if the authors feel this extra content is important.
Line 90: Extra citation
Line 128-132: This is exactly what I had in mind. It makes it clear to me upfront what your contribution is. Very helpful!
Line 173-175: I would argue this justification is extra, since a Type II functional response already an expected part of the RM model and you have already argued why you are using the RM model (Lines 141-144). Don't remove it on my account if you have another reason to keep it in, but it might help with flow.
[Main Concern #1] Line 331-333: Why did you decide that the extinction threshold you set in the methods was not the right metric? You conclusion, that the RM model has a higher probably of extinctions across your gradients, is based on assuming the extinction threshold doesn't matter. It would help me understand your claim if you state why we should not consider the extinction threshold. I think you need one more step here to make it clear why you are using an extinction threshold and what it means.
Line 344-347: I really like the addition of this proof. I have a concern about one step (see comment Line 922).
Line 397-415: I suggest moving this to the methods section.
Line 402-405: OK, I follow your explanation now. Thanks for the extra clarity!
Line 466-479: I really like this paragraph.
Line 490: A good citation here would be Menge et al. (2012: PLoS One). I think relaxing this assumption would be a really exciting addition to this framework, but I agree that it is beyond the current scope.
[Main Concern #2] Line 922: You make the assumption that e^ns = emax in your proof. This assumes that somebody fitting the RM model would parameterize e^ns as the maximum possible assimilation efficiency rather than the expected assimilation efficiency given the standing heterogeneity in resource quality. I do not think this is a reasonable assumption. Both a literature parameterization of e^ns and a model fit to empirical data (assuming the parameters are identifiable), should settle on a value for e^ns that is the average value for a population experiencing heterogeneity in resource quality. This would mean that e^ns < emax.
Line 940: Can you provide the year for the Yodzis paper in Table S1 and add it to the reference list?
https://doi.org/10.24072/pci.ecology.100067.rev21Evaluation round #1
DOI or URL of the preprint: https://doi.org/10.1101/589895
Author's Reply, 25 Nov 2019
Decision by Elisa Thebault, posted 15 Oct 2019
Dear authors, I have now received two reviews of your manuscript. Both reviewers and I are in agreement that this is an interesting study considering how flexible stoichiometry of primary producers affect the dynamics of primary producers and consumers in response to both nutrient enrichment and climate warming. However, several issues have been identified which, in my views, require revision before recommendation. Such revised contribution would need to address all of the reviewer comments. In particular, as outlined by reviewer #2, several points would deserve to be further clarified and discussed (e.g. better justification of the use of different extinction thresholds, robustness of the results to different sets of parameters, consequences of abiotic pools such as detritus).
In addition to the detailed suggestions of the reviewers, I have a few additional comments: - Lines 352-358: The definition of the static and dynamic effects are at first not fully clear. Is the part on consumer energetic efficiency (lines 358-378) related to the static effect while the following part (lines 382-393) relates to the dynamic effect? I better understood the difference between the static and dynamic effects later on (line 395), when these two effects are directly compared. I would suggest modifying this section (from lines 347 to 414) to make the distinction and the comparison between the static and dynamic effects clearer to the reader.
Lines 387-389 “When consumer population increases, this decreases resource population growth leading to a negative feedback on consumer population growth rate”: isn’t it also the case in the RM model? Please explain more precisely why this feedback is different in the SRM model.
Discussion on the effects of temperature on assimilation efficiency (lines 481-493): It could be interesting to discuss a little further which mechanisms lead to reduced assimilation efficiency of the consumer (in relation to reduced resource nutrient quota) at high temperature in the model. This result could potentially also be related to existing experimental and empirical literature on the effects of temperature on the stoichiometry of primary producers (although the mechanisms involved might be indeed different in the model).
I am looking forward to seeing your revised manuscript addressing the reviewers’ comments, along with a point-by-point response.
Best wishes, Elisa Thébault
Reviewed by anonymous reviewer 1, 06 Oct 2019
The manuscript investigates the influence of resource quality and temperature on consumer persistence in aquatic systems. For their theoretical investigations the authors extend the Rosenzweig-MacArthur model to include flexible stoichiometry at the resource level and temperature dependent resource and consumer rates. The authors follow published approaches and parameterizations. The study is well designed, the methods sound. The results are nicely explained and illustrated. As stated by the authors the presented study is a first step towards realistic theoretical approaches that are able to provide realistic predictions on the influence of climate warming and changes in nutrient regime on community dynamics and abundance patterns. The insight from this adapted resource consumer model with flexible stoichiometry and temperature dependent rates is very interesting. Due to its simplicity the changes in dynamics when moving from fixed to flexible stoichiometry for different temperature regimes provides a mechanistic understanding of the underlying feedback mechanisms that lead to the observed shifts in coexistence, defined by nutrient availability and temperature, and consumer to resource ratios. The results highlight the necessity of including flexible stoichiometry and temperature dependence in ecological models that aim for predictions on community dynamics and species persistence along with climate change. What these results really imply with respect to management decisions will have to be investigated with more complex food web models. Apart from little typos I have no major remarks. Please find highlighted typos/small comments in the attached file.
Download the review https://doi.org/10.24072/pci.ecology.100067.rev11Reviewed by anonymous reviewer 2, 30 Sep 2019
Sentis et al. have examined the interactive effect of temperature and nutrient stoichiometry on stability in the Rosenzweig-MacArthur Model. I enjoyed the manuscript, but was left with 9 concerns outlined below. I hope that these comments will help the authors as they revise their manuscript.
1) Most importantly, the authors need to clearly explain how their work differs from the work on Uszko et al. 2017. Uszko et al. also analyze the impact of stoichiometry (they account for both C and P) and temperature on a similar Rosenzweig-MacArthur model. They make many of the same assumptions (functional responses, temperature responses) or even consider multiple cases. The also assess questions of stability, limit cycles, and variation across the two constraints. Since the authors use many of the same parameters and as Uszko et al., it is especially important that they indicate how their work is different. I envision a few sentences telling the reader how this work builds upon this and other earlier work.
2) I found the introduction to be repetitive. The authors have excellent content, but have at least 3 paragraphs making the point about the lack of studies with temperature x stoichiometry--this need only be said once. It could be collapsed into ~4 paragraphs instead of the current 7.
3) In most systems, the majority of nutrients are held in abiotic pools and biotic pools that have long turnover times (e.g. wood). Since you assume that there is a fixed amount of nutrients (Ntot) that is shared between the resource and consumer, the only source of new nutrients for the resource (i.e. plants) is from dead consumers. I think this is a questionable assumption for most ecosystems, wherein consumer mediated recycling might be important but is certainty not the dominant source of nutrients accessed by autotrophs. In an aquatic system, the other nutrient pool would be dissolved organic (i.e. detritus) and inorganic nutrients. For example, Uszko et al.(2017) consider dissolved nutrients.
4) I do not understand the extinction threshold. The authors set an extinction threshold in the methods section. They say that considering data below this threshold lead to unrealistic results (Line 280). However, in the results section they use that threshold to contrast the SRM versus RM. I think these two approaches are in conflict. Can the authors resolve this?
5) The excitation threshold is also very low (10^-9). Therefore, I believe that the statement in the methods (that low populations should be considered extinct) is correct. The authors say that they simulated the SRM model (Line 271), but do not tell us how. Depending on the solver they used, these simulations could have different levels of precision. However, often the precision is only good to 10^-5 (R package "deSolve"), so the analysis of results below 10^-9 might not be acceptable. The authors can clear this up by providing information on the solver they used and its precision.
6) The biomass ratios of the RM model are a function of parameters, so the RM could predict a biomass ratio of below 1 with different parameters. This leads me to ask: how general are the parameters used and how different would they need to be for the RM model to predict a biomass ratio below 1? If the assimilation efficiency, attack rate where low or the mortality or handling time were higher, the biomass pyramid would probably be inverted. SRM likely predicts a biomass ratios below 1, because the nutrient and temperature ratios chosen adjust these parameters to values that make consumers less abundant than their resources. I am left wondering, do the models always produces these differences, or is it based on parameter choices? (I also suggest calling these biomass ratios, because pyramid suggests more than two species.)
7) Figure 1: Since the number of species is discrete, I suggest using a discrete color scheme.
8) I do not understand how the effective RM and SRM are different. Can you clarify this explanation?
9) You say that your model demonstrates that assimilation efficiency is temperature dependent in contrast to other evidence (Line 484-486). However, assimilation efficiency is temperature dependent in your model because of the structural assumptions you make. Consequently, your model hypothesizes what dynamics would look like if assimilation efficiency were temperature dependent. I think it is circular to suggest, in the absence of empirical data related assimilation efficiency and temperature, that your model demonstrates temperature dependence.
https://doi.org/10.24072/pci.ecology.100067.rev12