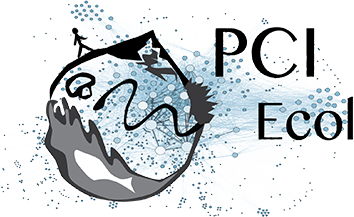
Diving into detection process to solve sampling and abundance issues in a cryptic species
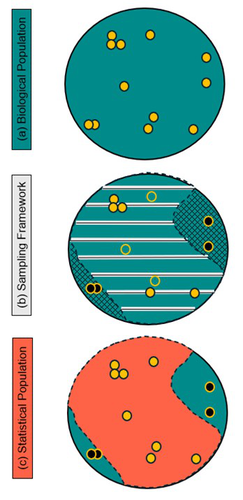
Using multiple datasets to account for misalignment between statistical and biological populations for abundance estimation
Abstract
Recommendation: posted 28 October 2024, validated 07 November 2024
Souchay, G. (2024) Diving into detection process to solve sampling and abundance issues in a cryptic species. Peer Community in Ecology, 100640. 10.24072/pci.ecology.100640
Recommendation
Estimating population parameters is critical for analysis and management of wildlife populations. Drawing inference at the population level requires a robust sampling scheme and information about the representativeness of the studied population (Williams et al. 2002). In their textbook, Williams et al. (see chapter 5, 2002) listed several sampling issues, including both temporal and spatial heterogeneity and especially imperfect detection. Several methods, either sampling-based or model-based can be used to circumvent these issues.
In their paper, Kissling et al. (2024) addressed the case of the Kittlitz’s murrelet (Brachyramphus brevirostris), a cryptic ice-associated seabird, combining spatial variation in its distribution, temporal variation in breeding propensity, imperfect detection and logistical challenges to access the breeding area. The Kittlitz’s murrelet is thus the perfect species to illustrate common issues and logistical difficulties to implement a standard sampling scheme.
The authors proposed a modelling framework unifying several datasets from different surveys to extract information on each step of the detection process: the spatial match between the targeted population and the sampled population, the probability of presence in the sample area, the probability of availability given presence in the sample area and finally, the probability of detection given presence and availability. All these components were part of the framework to estimate abundance and trend for this species.
They took advantage of a radiotracking survey during several years to inform spatial match and probability of presence. They performed a behavioural experiment to assess the probability of availability of murrelets given it was present in sampling area, and they used a conventional distance-sampling boat survey to estimate detection of individuals. This is worth noting that the most variable components were the probability of presence in the sample area, with a global mean of 0.50, and the probability of detection given presence and availability ranging from 0.49 to 0.77. The estimated trend for Kittlitz’s murrelet was negative and all the information gathered in this study will be useful for future conservation plan.
Coupling a decomposition of the detection process with different data sources was the key to solve problems raised by such “difficult” species, and the paper of Kissling et al. (2024) is a good way to follow for other species, allowing to inform the detection components for the targeted species - and also for our global understanding of detection process, and to infer about the temporal trend of species of conservation concern.
References
Williams, B. K., Nichols, J. D., and Conroy, M. J. (2002). Analysis and management of animal populations. Academic Press.
Michelle L. Kissling, Paul M. Lukacs, Kelly Nesvacil, Scott M. Gende, Grey W. Pendleton (2024) Using multiple datasets to account for misalignment between statistical and biological populations for abundance estimation. EcoEvoRxiv, ver.3 peer-reviewed and recommended by PCI Ecology https://doi.org/10.32942/X2W03T
The recommender in charge of the evaluation of the article and the reviewers declared that they have no conflict of interest (as defined in the code of conduct of PCI) with the authors or with the content of the article. The authors declared that they comply with the PCI rule of having no financial conflicts of interest in relation to the content of the article.
We conducted this study with primary assistance from the U.S. Fish and Wildlife Service, National Park Service (Wrangell-St. Elias National Park), Alaska Department of Fish and Game (ADFG), and University of Montana.
Evaluation round #2
DOI or URL of the preprint: https://doi.org/10.32942/X2W03T
Version of the preprint: 2
Author's Reply, 15 Oct 2024
Decision by Guillaume Souchay
, posted 27 Aug 2024, validated 28 Aug 2024
Dear authors,
I would like to thank you for the work done on the previous manuscript.
2 previous reviewers and myself have read this new version of your ms. All of us concur that you did a great job to improve the ms, especially to make your point clearer.
The introduction is clearer and the concept is better introduced and I think there are only minor changes to do before recommendation. The text is clear, easy to read. I like the new paragraph in the introduction as well as the figure 1.
Depending on whether the authors will try to publish their work afterwards (and the potential formatting of the journal), uou could consider using more subtitles to highlight the structure of the paper, especially in the Method and the data analysis sections.
Before recommanding your manuscript, 1 reviewer and 1 still have some minor details/comments for you.
==========
L.58: “available for” instead of “under observation”?
L.68-77: this paragraph could be the start of the introduction, or mixed with the first paragraph as it is the key of your introduction and your subject.
L.124: was it logistically impractical? If so, may be add “logistically” in the sentence
L.131-144: I am wondering whether setting the name of the parameter before its description could be “better” than within brackets. A general diagram of your framework could be interesting to sum up the proposed model.
L.170: model or framework?
L.176: perhaps replace “later” by “further”. If your manuscript is under review, the “later” use is already there
L.214-215: why did you change the protocol for these years? Was it a test/constraint?
L.233-243: set the reference at the beginning of the paragraph
L.245-264: maybe make one paragraph from these 2? Could you also provide some hypothesis underlying the choice of these environmental data?
L.278-304: these 2 paragraphs should be only 1. The break seems unnecessary.
L.307: insert “, following Lukacs et al. 2010” after “given presence”
L.482-484: could it be possible to show the posteriori distribution of mean r for both situations? Given the CrI, one may expect some symmetry around 0, and thus, something not really declining. Nonetheless, the decline seems stronger for the biological population than the statistical population. This may be worth noted.
L.540-543: further investigation, perhaps using simulation, could help to understand the behavior of the model
L.598-600: is there any proxy available for prey availability?
Reviewed by Michael Schaub, 02 Jul 2024
I am generally satisfied with the revision of the manuscript and/or the responses to the points I raised earlier. The presentation of the biological vs. statistical population is now clearer and I can see the value in keeping it (I had earlier argued that it might be removed). The full text is still quite wordy and long and I wondered if it could be made more concise by omitting some of the detail. However, it is difficult to make specific suggestions, so my advice is simply that the authors might try to streamline the text when they read it again.
Otherwise, I only have two specific minor points:
L 102: residual (typo)
L 499: 'all' sources of uncertainty may be an exaggeration. Perhaps better replace 'all' with 'multiple'.
Reviewed by Chloé Nater
, 23 Jul 2024
This is my second round reviewing this paper, and it has improved substantially since the last version. Most notably, well annotated code is now available for all parts of the workflow, and everything I have tested seemed to work fine (I nonetheless provide some suggestions for improving reproducibility below). The pesentation of the modelling framework is also clearer now, but some more information is required in some places and I have outlined these in specific comments below. Now that I have a better understanding of how this modelling framework is coming together, I would like to strongly encourage the authors to make a schematic/graphic overview of the framework that visualizes how the different component models are linked and how information flows within the framework (i.e. via shared parameters, estimates used as inputs, etc.). To us, who have written the models themselves, it always makes "intuitive sense" how things are connected, but that does not necessarily translate to everyone else. Especially with a multi-tiered (and somewhat unorthodox) framework like what is used here, a good graphic could really make a HUGE difference for your readers in terms of (ease of) understanding your framework.
Below are my more general and more specific suggestions for the second round of revisions.
# General: I am not familiar with how much typesetting will be done for publication in the Peer Community Journal, but one thing I would like to point out it that presently, all paragraph breaks are done with an empty line in between. I do appreciate white-space for structuring text, but in many places white space currently divides up chunks of text that belong together (i.e. are part of the same "subsection" / topic). Some readers may find this disruptive, and - depending on the degree of typesetting during the publication process - I would like to encourage you to revisit the visual aspects of text structure before the final version is published (I outline a couple of specific examples below).
# Code: I greatly appreciated having access to all code and data now. I have tried to work through most of the scripts, and this worked out well, not least thanks to decent documentation. Here are a few things I noticed nonetheless that could be improved for better reproducibility and transparency:
- MCMC pre-sets: These are currently set up for a test run (1 chain, 50 iterations, etc.). Please also add the setup for your full runs.
- Model codes: It would really help with understanding & running the model to have the parameter definitions at the start of the model code (for all variables that appear in the code)
- Absolute paths: You are currently using absolute paths for your computer to set the working directory. I recommend using relative paths instead. You can do this either by adding an Rproject to your repositories' root directory or by setting the working directory manually to "several directories up" from within each script. The former may be the easiest solution. The bare minimum I would recommend is changing the setwd() statement to something like setwd(".../DataCode_BRMU_Kissling_et_al") and clearly write out in comment that people will have to adjust the path to match their folder structure. Irrespective of how you chose to approach it, adding documentation and clear instructions about that would greatly enhance reproducbility.
# Line 18: "census" is not enough to be informative. How about "complete population census"?. Also, one could argue that even a complete census will still need "sampling". This is the second sentence in your abstract and you need to get your readers interested here, so I recommend considering less ambiguous wording.
# Lines 30-31: Still on the topic of capturing/retaining attention: Could you relate that back to getting an appropriate estimate for biological population size?
# Line 43: "suitable" instead of "feasible"? (Fitting wrong models is totally feasible)
# Line 58-59: See comment above. I think this distinction between "census" and "sampling" is very confusing. If you want to stick with these terms, you may need to specify that you are comparing "complete census" to "partial sampling", or something similar.
# Lines 68-77: I like this new paragraph, it helps better understanding the concept. I feel like it might be better placed even earlier in the introduction though. Both because it's fundamental, and because in it's current position, it "breaks" the introduction of p_p.
# Line 102: "residual"
# Line 116: See comment above about "feasibility" of models.
# Lines 245-264: Did these two paragraphs get switched around? The second has the introductory sentence to environmental data and seems to refer to the first using "below"... I would recommend adding a little "flag" for environmental data, just as you have for "Boat surveys" and "Telemetry surveys".
# Line 276: To come to the conclusion that you do not need it, you will have had to compare something though. What did you compare? UD of the same individual across years? UDs of several individuals in the same year? You need to explain to the reader how you reached the conclusion of p_s = 1.
# Line 292: Here is an example of where the blank space line break really threw me off. The paragraph on line 293 starts with re-defining p_p and makes it look like this is about another parameter than the previous paragraph. But it is not, right? Would really help the reader here to have a more obvious "bridge", e.g. the motivation behind testing the different time windows.
# Line 306: I can see that you cite a previous study for the details of the "boat-based dive behavior trials", but I think your readers would appreciate one additional sentence about what these trials were and how they helped you reach that conclusion. No need for details, but we need to know the "gist of it" for this to look robust and make sense.
# Line 313: Please add a reference for "conventional distance sampling". Also, perhaps in a bracket just give us what you mean by "conventional" (e.g. line transects, half-normal detection, etc.).
# Line 321: Another example where the blank line break can be misleading. This pertains to the distance sampling model, right? The way it looks now, it might be that, or it might be everything.
# Line 312-330: Here, you present the distance sampling approach to estimate p_d. You state that you looked at AICc, which tells me that this was a frequentist model. In the code, the distance sampling likelihood only appears in the code for abundance estimation, in a Bayesian framework. What am I missing? Was the first model a frequentist "pre-analysis" (if so, what was its purpose)? Or was distance sampling data used only in the joint framework (if so, why have two separate introductions)?
# Line 380-381: "Moreover, no parameters were shared across years and therefore, we would not have gained anything by running the model with all years simultaneously." As you have set up your models, this is true and there is nothing wrong with it. In a dynamic model (= multi-year) model, however, you would have the possibility of sharing information across years (e.g. by using year random effects instead of independently estimating yearly rates) and I imagine that will start making quite a difference. I think it would be nice to add a sentence or two reflecting on this in the discussion (e.g. around line 529). You mention that you have an IPM paper on the way... don't be shy to hype us up a bit about that.
# Line 383-405: This seems very out of place here. What is the reason why this is not presented together with the model for estimating p_p (i.e. around line 305)? The other thing that needs clarifying is what you mean by "predicting" as opposed to "estimating"? The way this reads now, you could have done any of the following two analyses:
1) Re-run model for estimating p_p from data with covariates.
2) Correlated the posterior samples for p_p (as estimated from data with your previous Bayesian model) with potential environmental covariates.
By looking at the code, I can see that it was the former, but this should be clarified in the text, too. Could you also provide a reference for and clarify the aim of your cross validation? Finally, I think it would make it easier for the reader to follow if this extra investigation into environmental effects was presented together with the "base model" for p_p.
# Line 407-408: Could you elaborate on this a bit? The statistical population is a subset of the biological population, meaning that for calculating the biological population by correcting for the different detection aspects, you need the estimates of the statistical population. With that being the case... it appears like modelling the statistical population twice would be the waste of computation, not modelling them in one model?
# Lines 425-426: It would help to be a bit more specific here. What you did do - according to your code - was using the mean and sd of abundacne estimates from your previous models as "input-data" for the state-space model. This is equivalent to using an informative prior, except for the scaling of the error. This should be stated more explicitly.
# Line 446: In your reply to previous comments, you provided your reasoning for your mixed reporting of standard deviations and credible intervals. While I cannot see how practical aspects of plotting should affect what is reported in the text, I won't dwell on this further. The one thing that IS important, however, is that you reporting "SE" in the text is likely not correct. The outputs from JAGS - the very numbers you report - are mean and standard DEVIATION (SD, called "sd.vect" in your R objects). SE = SD / sqrt(n), where n is the sample size. So unless you are doing a post-hoc correction for sample size, you are likely reporting standard deviation, not standard error.
# Line 501-502: I recommend re-defining p_p here. Some readers may skip over substantial parts of methods/results to check conclusions, and they won't know what p_p is.
# Line 510: Could you add one sentence here reflecting on some other species/situations in which having a model like yours would be super useful? I would quite like to leave this paragraph having a good idea of the value of your framework beyond your study population of murrelets.
# Data and code availability statement: Please add a sentence about where code can be found, too.
Evaluation round #1
DOI or URL of the preprint: https://doi.org/10.32942/X2W03T
Version of the preprint: 1
Author's Reply, 20 Apr 2024
Please see attached cover letter and revised manuscript with Track Changes.
Decision by Guillaume Souchay
, posted 01 Mar 2024, validated 10 Mar 2024
Dear authors,
Thank you for considering PCI Ecology to recommend your work.
I personally apologize for the delayed response due to underestimated fieldwork when I received reviews.
I have now received 3 reviews for your manuscript, and I have also read it. All reviewers and I agree about the importance of your work. To assess availability of individuals to detection into the detection process to estimate population size is a critical point in ecology.
Before recommending your ms, I consider that some clarifications could improve the quality of the manuscript.
Based on their review, I suggest you to revise your manuscript according to 2 main points:
- you introduced a strong opposition between the statistical and the biological populations. It can be sometimes confusing and/or misleading.I am not sure “population mismatch” is the proper concept here. Is it really a mismatch or some inadequacy in the sampling method? Is it really an opposition or 2 components of the population? The question would be more how a portion of a population is representative of the entire population. Such “mismatches” can occur in count sampling but it can also be real with other kind of surveys. It could improve the manuscript to reconsider the importance of this opposition to introduce your study.
- The methods section. Despite all reviewers agree on how the tools were used, they were all concerned by possible confusion and/or lack of explanation on the models, the parameters and the definition, in particular the use of “integrated model” when all models are used separately. In your revision process, keep in mind that there is no word limits in PCI Ecology. So, when needed, do as long as necessary to help the readers to really understand what and why you are doing this way.
Reviewers also provide minor comments to consider.
Guillaume Souchay
==========
Introduction.
I understand the point about the discrepancy between the global population and the sampled population / the fraction of the population we are able to sample. However, I am a bit uncomfortable with the opposition between the “biological” and the “statistical” populations. They are presented as different but the latter is a portion of the former. Thus, I’m a bit concerned about the use of “mismatch” instead of directly questioning the representativeness of the sampled population. Such issue can arise when estimating population size, but also when marking individuals, which could lead to bias demographic parameters. This could in turn result in divergent population projections, whether derived from a matrix population model or from counts trends.
Method section.
Boat surveys. Is there a reason why only 1 boat was used on 2009?
Telemetry survey. I am wondering whether the schedule of the capture could induce some bias. Could it be possible that only some kinds of individuals are available for capture, e.g. only “early breeder”?
Data analysis.
L.256. Is it really an integrated model? Usually, integrated model refers to model incorporating all sub-models, and estimating all parameters at the same time, thus allowing to include all uncertainties and also all dependencies between the parameters. As presented here, it seems that the components were estimated independently and then used together. Perhaps, providing a graph of the integration of all submodels could help to understand.
L.270. Are these individuals transients?
L.290. Is it part of the current study? If so, this result could be reported in the result section.
L.321. It is quite difficult to retrieve the different detection probabilities in the code provided in Appendix 4, as it seems that different notations are used.
Result section.
L.401-403. About 1/3 of individuals left the area (or died?). This is quite important. Do you have any idea of the potential reason? Does it include non-breeders individuals leaving the area before the breeding season?
Discussion
L.498-508. Was it really the same telemetry dataset? For the current study, 1/3 of individuals were not included as either dead or absent after the 1st of July. It questions the representativeness of the captured individuals also, and inferences drawn only based on telemetry dataset. As stated previously in the text, the use of an integrated population model could be very interesting.
L.595-598. Promotion of IPM could also help to avoid such bias, as the Bayesian network is quite flexible.
Reviewed by anonymous reviewer 1, 06 Feb 2024
Reviewed by Michael Schaub, 31 Jan 2024
The manuscript deals with the estimation of different components of detection probabilities, which are essential for estimating abundance and its change over time. The motivation for the work is a dataset on Kittlitz's murrelets. These murrelets are difficult to study because of their spatial dynamics (movements) and the challenging environment in which they live. Different types of data were used to estimate the probability of presence in the sampling area, the probabilities of detection and of correct identification if present. Abundance estimates differ depending on whether the probability of presence is included. If it is included, the size of the 'complete' population is estimated (the biological population); if it is not included, the part of the population present in the sampling area at the time of sampling is estimated (the statistical population). The difference between the biological and the statistical population is a hook of the story. The study is well done, using state-of-the-art statistical models, and is generally well written. I have one major comment and a couple of minor ones.
Major comment:
The hook of the story is the distinction between the biological and the statistical population. I found this unconvincing and unnecessary. It is unconvincing because the definition of the statistical population is not clear - the authors themselves used different ones (see minor point below). We can see from the results that the biological population is estimated taking into account the probability of presence in the sampling area, whereas the statistical population is estimated without taking into account the probability of presence, so the latter is the population size of birds that were actually present in the sampling area at the time of sampling. Why should this be called biological versus statistical population? I see no benefit in using this construct - in fact it seems unnecessary. I suggest calling them what they are: the biological population is the total population (defined as individuals that are at least temporarily present in the sampling area) and a fraction of that population is actually present in the sampling area at the time of sampling (the statistical population). If, for example, the presence and absence in the sampling area were due to breeding (presence) and breeding skippers (absence), nobody would call the estimate of breeders the 'statistical population size'.
Therefore, in my opinion, the introduction of the concepts of biological and statistical population adds to confusion rather than to understanding. I therefore suggest reconsidering whether this concept is really needed for this study.
Minor comments:
L17: This is a rude entry, I'm not sure there is a clear understanding of what a statistical population is. I suggest adding a sentence to make the context clearer.
L 41: consider deleting ‘true’.
L 63ff: I'm a bit confused by these descriptions. In reality, there is only one (biological) population from which samples are taken. On the basis of the sampled data, we make inferences that are valid for a hypothetical statistical population. Ideally, the biological and hypothetical statistical populations are the same. But because of sampling problems or an inadequate statistical model, the two may not be the same, and so our inference is not valid for the biological population. But as described in the manuscript, it gives the impression that you can sample from a statistical population, which (to my understanding) is not possible. There also seems to be a contradiction in the definition of a statistical population. Here you claim that the researcher defines the statistical population, but in the first paragraph the statistical population was a construct to infer what we want to know.
L 87: what is a statistical driver?
L 301: I do not understand what you mean by ‘we truncated the upper 5% of distance data’.
L 328: should be pp,il instead of pp,I, otherwise it does not match the following text.
Use Courier font for the code, this makes it much easier to read.
Reviewed by Chloé Nater
, 12 Feb 2024
The preprint «Integrating multiple datasets to align biological and statistical populations for abundance estimation» picks up an important topic in statistical ecology: mismatches between biological populations that we would like to draw inference on and the – often not representative – portion of it for which we are able to collect data and hence build models. Kissling et al. present a modelling framework to address such mismatches. Their approach makes use of several sources of data to decompose the detection process into components that describe whether individuals are observable at all (i.e. part of the statistical population that is represented by monitoring data) and components that describe detectability of individuals given that they are available for detection. The manuscript is written in an accessible and concise manner, and uses a case study of Kittlitz’s murrelet, a cryptic seabird, to showcase how their modelling framework is used in practice.
My primary suggestions for improvement revolve around presentation and documentation of both the modelling framework itself and the workflow in which it is embedded, and are summarised in the following “major comments” section. Following that, I also provide some line-specific minor comments aimed at improving readability and accessibility of the text.
MAJOR COMMENTS
1. The study includes not just one but several (implementations of the same) models, but these are not very well delineated when described in the text. First, the different components of detection seem to have been modelled independently and separately (possibly even using frequentist approaches as indicated by the reporting of delta AIC). Next, we are introduced to the “main” model, which integrates estimation of two detection components with estimation of population sizes. From the model code and results, I pieced together that this model must have been a closed population model, which was applied – independently – for each year/season in the data, but this is not clearly described. Then, there is another model that was used to estimate environmental covariates on one detection component, and finally, we are also introduced to a final model for estimating abundance trends. This latter one was a state-space model, which integrated data across years but did not account for detectability the same way as the main model. At present, figuring out what the different models were and how they are related from the text is not trivial, and readers would benefit a lot from clearer description of each model, its data likelihood and hierarchical structures (see minor comments for specific suggestions), and how it fits into the larger framework together with the other component models. The latter is particularly important, because the reader is left with some big open questions regarding modelling choices. For example, I cannot help but wonder why your main model was applied separately for each year, instead of linking the data together across years (which would increase statistical power by a fair bit, I imagine). I also cannot see why covariates were modelled in a separate model, instead of the main framework. I am sure there are good reasons for all these modelling decisions, but providing some background information would make it much easier for readers to navigate and understand the framework.
2. Code is provided for only two models, presumably the main integrated model and the state-space model for abundance. Annotation of the code, especially for the latter model, could be improved a lot. At present, annotation is sparse, and the fact that different variable names are used in the text and the code (without a key or further explanations) turned reading the code into a guessing game. Your modelling approach holds value not just for your particular study, but could be potentially useful in many situations. Hence, it would be such a shame if your framework ended up challenging to reuse/adapt due to lack of annotation, and I highly recommend improving documentation and making it easier to connect formulas and results in the text to the model code.
3. There is another aspect of the way you present your code that will make reusing and/or adapting it rather difficult: your model code is in your pre-print’s supplementary, pasted into a word document. While quite a few Bayesian models in Ecology have been (and maybe still are) published this way, it’s important to be clear over that this is neither reproducible nor particularly useful to anyone. Most of us implement our analyses using R (or similar software) and sharing code in the format it is used in in practice is the preferred option here. To ensure reproducibility, it is also best to share not only the model code itself. The BUGS/JAGS code tells very little about how to implement a model in practice. Questions like “what is the format of the input data?”, “how were initial values simulated?”, “how were post-hoc analyses implemented?”, “how were the results converted for making Figure X?” inevitably arise when picking up someone else’s code, but they will remain forever unanswered if the BUGS code is the only thing provided. I encourage you to consider to what degree you may be able to supplement your model code with scripts for the other parts of your workflow, and make your code workflow available using a suitable repository (e.g. GitHub). This would give you better means do document code, and make your modelling framework much more applicable, reusable, and reproducible.
MINOR COMMENTS
# Line 35: I assume this “r” is population growth rate. As this is the abstract, I recommend spelling that out.
# Line 36: Unclear whether “variance”/”variation” in this sentence applies to detection or biological processes.
# Lines 64-66: “However […] population of interest.” Isn’t the variable exposure what leads to mismatch in the first place (i.e. is this a chicken-or-egg problem)?
# Lines 76-83: Consider whether you could give one illustrative example each for both of the reasons here. This would help the reader conceptualize better here.
# Line 105: “nor” instead of “or”
# Line 107: “habitats are too complex”. In what way(s)?
# Line 117: Feels like there is a “linking” sentence missing here which states that such cases lead to mismatches between biological and statistical populations.
# Line 120: Here, it feels like we should have a sentence that conceptually descrbes how decomposing detection van help with handling mismatch. It may not follow “naturally” for your reader.
# Line 132: Suggest rephrasing as “[…] provided that data for estimating components are available”.
# Line 163: “variation” across space? Time? Both?
# Lines 195+: What I miss throughout the data collection section is some information on how the data were formatted to prepare them from analysis (reference could be made to relevant data wrangling code too, should you choose to make that available).
# Line 198: Why was there only one survey in 2009?
# Line 214: How did you determine if individuals were older than 2 years?
# Line 263: It may make sense to take p_a after p_s, as they are mentioned in that order on line 257 and are the two components that you did not further explore in the integrated model.
# Line 277: Re-define probability, as you do in the other 3 corresponding paragraphs?
# Lines 328-330: The formula here does not correspond to a “model for p_{p,i} on the logit scale”. That would be “logit(p_{p,i}) <- beta_i” as in the code. The formula on line 330 seems to be a data likelihood (involving the parameter p_{p,i}) for some data y which you do not clearly define in the text. It may help with readability to define all variables in formulas in text, and to disctinguish between data likelihoods and variable definitions / hierarchical models for parameters.
# Lines 367-376: There seems to be no script for this part of the analysis?
# Lines 380-381: “[…] weighted the response variable (log abundance) by the inverse of its variance.” I may be missing something obvious, but it does not become clear why this makes sense / was done.
# Line 393: Wide normal priors (as you use for "beta" variables") on the logit scale are not "weakly informative" on the probability scale. The resulting probability priors have most of the weight close to 0 and close to 1. You have plenty of data for estimating the relevant parameters in this case, so I doubt this makes a difference in practice (but if you wanted to make sure, you could always test if you get the same results when using Uniform[0, 1] on the probability scales instead, e.g. like your data augmentation parameters)
# Lines 399+: There seems to be some inconsistency in how results are reported. Sometimes credible intervals are used, sometimes standard errors (are they standard errors or standard deviations), and sometimes no uncertainty is reported. It might be best to be consistent.
# Lines 442-443: It did seem a bit odd to me that the CV of the 2009 estimate from the state-space model was so low. There was less data, so there should be more uncertainty. How was the “missing survey” dealt with in the state-space model?
# Lines 450-452: I am admittedly not very familiar with RMSE, but I cannot quite see how you arrive at this conclusion here. Also how can uncertainty in r decrease, but uncertainty in lambda (=exp(r)) go up? Perhaps this could be supplemented with a short explanation for other readers like me?
# Line 463: This is a bit related to comment 1. above but it seems to me that this was not a study that used “a single analytical framework” but rather a combination of different models…
# Line 488: How are mean(r) and trend different? Perhaps these could be clearly defined somewhere.
# Line 545: Suggestion: “Despite our poor ability to predict p_p from environmental covariates” (you estimated it fine otherwise).
# Line 623: I miss your in-text reference to the code. This could be changed to “Data and code availability statement” and proper reference to the code added here, for example.
# Figure 4: It’s difficult for me (and probably most readers) to read any relationships (or lack thereof) from this kind of graph. Could it be an idea to add the model prediction (mean with uncertainty band) to the graphs?