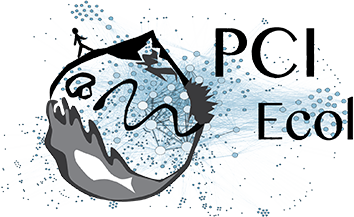
Understanding the interplay between host-specificity, environmental conditions and competition through the sound application of Joint Species Distribution Models
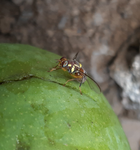
Joint species distributions reveal the combined effects of host plants, abiotic factors and species competition as drivers of species abundances in fruit flies
Abstract
Recommendation: posted 23 April 2021, validated 27 April 2021
Hortal, J. (2021) Understanding the interplay between host-specificity, environmental conditions and competition through the sound application of Joint Species Distribution Models. Peer Community in Ecology, 100080. 10.24072/pci.ecology.100080
Recommendation
Understanding why and how species coexist in local communities is one of the central questions in ecology. There is general agreement that species distribution and coexistence are determined by a number of key mechanisms, including the environmental requirements of species, dispersal, evolutionary constraints, resource availability and selection, metapopulation dynamics, and biotic interactions (e.g. Soberón & Nakamura 2009; Colwell & Rangel 2009; Ricklefs 2015). These factors are however intricately intertwined in a scale-structured fashion (Hortal et al. 2010; D’Amen et al. 2017), making it particularly difficult to tease apart the effects of each one of them. This could be addressed by the novel field of Joint Species Distribution Modelling (JSDM; Okasvainen & Abrego 2020), as it allows assessing the effects of several sets of factors and the co-occurrence and/or covariation in abundances of potentially interacting species at the same time (Pollock et al. 2014; Ovaskainen et al. 2016; Dormann et al. 2018). However, the development of JSDM has been hampered by the general lack of good-quality detailed data on species co-occurrences and abundances (see Hortal et al. 2015).
Facon et al. (2021) use a particularly large compilation of field surveys to study the abundance and co-occurrence of Tephritidae fruit flies in c. 400 orchards, gardens and natural areas throughout the island of Réunion. Further, they combine such information with lab data on their host-selection fundamental niche (i.e. in the absence of competitors), codifying traits of female choice and larval performances in 21 host species. They use Poisson Log-Normal models, a type of mixed model that allows one to jointly model the random effects associated with all species, and retrieve the covariations in abundance that are not explained by environmental conditions or differences in sampling effort. Then, they use a series of models to evaluate the effects on these matrices of ecological covariates (date, elevation, habitat, climate and host plant), species interactions (by comparing with a constrained residual variance-covariance matrix) and the species’ host-selection fundamental niches (through separate models for each fly species).
The eight Tephritidae species inhabiting Réunion include both generalists and specialists in Solanaceae and Cucurbitaceae with a known history of interspecific competition. Facon et al. (2021) use a comprehensive JSDM approach to assess the effects of different factors separately and altogether. This allows them to identify large effects of plant hosts and the fundamental host-selection niche on species co-occurrence, but also to show that ecological covariates and weak –though not negligible– species interactions are necessary to account for all residual variance in the matrix of joint species abundances per site. Further, they also find evidence that the fitness per host measured in the lab has a strong influence on the abundances in each host plant in the field for specialist species, but not for generalists. Indeed, the stronger effects of competitive exclusion were found in pairs of Cucurbitaceae specialist species. However, these analyses fail to provide solid grounds to assess why generalists are rarely found in Cucurbitaceae and Solanaceae. Although they argue that this may be due to Connell’s (1980) ghost of competition past (past competition that led to current niche differentiation), further data on the evolutionary history of these fruit flies is needed to assess this hypothesis.
Finding evidence for the effects of competitive interactions on species’ occurrences and spatial distributions is often difficult, perhaps because these effects occur over longer time scales than the ones usually studied by ecologists (Yackulic 2017). The work by Facon and colleagues shows that weak effects of competition can be detected also at the short ecological timescales that determine coexistence in local communities, under the virtuous combination of good-quality data and sound analytical designs that account for several aspects of species’ niches, their biotopes and their joint population responses. This adds a new dimension to the application of Hutchinson’s (1978) niche framework to understand the spatial dynamics of species and communities (see also Colwell & Rangel 2009), although further advances to incorporate dispersal-driven metacommunity dynamics (see, e.g., Ovaskainen et al. 2016; Leibold et al. 2017) are certainly needed. Nonetheless, this work shows the potential value of in-depth analyses of species coexistence based on combining good-quality field data with well-thought out JSDM applications. If many studies like this are conducted, it is likely that the uprising field of Joint Species Distribution Modelling will improve our understanding of the hierarchical relationships between the different factors affecting species coexistence in ecological communities in the near future.
References
Colwell RK, Rangel TF (2009) Hutchinson’s duality: The once and future niche. Proceedings of the National Academy of Sciences, 106, 19651–19658. https://doi.org/10.1073/pnas.0901650106
Connell JH (1980) Diversity and the Coevolution of Competitors, or the Ghost of Competition Past. Oikos, 35, 131–138. https://doi.org/10.2307/3544421
D’Amen M, Rahbek C, Zimmermann NE, Guisan A (2017) Spatial predictions at the community level: from current approaches to future frameworks. Biological Reviews, 92, 169–187. https://doi.org/10.1111/brv.12222
Dormann CF, Bobrowski M, Dehling DM, Harris DJ, Hartig F, Lischke H, Moretti MD, Pagel J, Pinkert S, Schleuning M, Schmidt SI, Sheppard CS, Steinbauer MJ, Zeuss D, Kraan C (2018) Biotic interactions in species distribution modelling: 10 questions to guide interpretation and avoid false conclusions. Global Ecology and Biogeography, 27, 1004–1016. https://doi.org/10.1111/geb.12759
Facon B, Hafsi A, Masselière MC de la, Robin S, Massol F, Dubart M, Chiquet J, Frago E, Chiroleu F, Duyck P-F, Ravigné V (2021) Joint species distributions reveal the combined effects of host plants, abiotic factors and species competition as drivers of community structure in fruit flies. bioRxiv, 2020.12.07.414326. ver. 4 peer-reviewed and recommended by Peer community in Ecology. https://doi.org/10.1101/2020.12.07.414326
Hortal J, de Bello F, Diniz-Filho JAF, Lewinsohn TM, Lobo JM, Ladle RJ (2015) Seven Shortfalls that Beset Large-Scale Knowledge of Biodiversity. Annual Review of Ecology, Evolution, and Systematics, 46, 523–549. https://doi.org/10.1146/annurev-ecolsys-112414-054400
Hortal J, Roura‐Pascual N, Sanders NJ, Rahbek C (2010) Understanding (insect) species distributions across spatial scales. Ecography, 33, 51–53. https://doi.org/10.1111/j.1600-0587.2009.06428.x
Hutchinson, G.E. (1978) An introduction to population biology. Yale University Press, New Haven, CT.
Leibold MA, Chase JM, Ernest SKM (2017) Community assembly and the functioning of ecosystems: how metacommunity processes alter ecosystems attributes. Ecology, 98, 909–919. https://doi.org/10.1002/ecy.1697
Ovaskainen O, Abrego N (2020) Joint Species Distribution Modelling: With Applications in R. Cambridge University Press, Cambridge. https://doi.org/10.1017/9781108591720
Ovaskainen O, Roy DB, Fox R, Anderson BJ (2016) Uncovering hidden spatial structure in species communities with spatially explicit joint species distribution models. Methods in Ecology and Evolution, 7, 428–436. https://doi.org/10.1111/2041-210X.12502
Pollock LJ, Tingley R, Morris WK, Golding N, O’Hara RB, Parris KM, Vesk PA, McCarthy MA (2014) Understanding co-occurrence by modelling species simultaneously with a Joint Species Distribution Model (JSDM). Methods in Ecology and Evolution, 5, 397–406. https://doi.org/10.1111/2041-210X.12180
Ricklefs RE (2015) Intrinsic dynamics of the regional community. Ecology Letters, 18, 497–503. https://doi.org/10.1111/ele.12431
Soberón J, Nakamura M (2009) Niches and distributional areas: Concepts, methods, and assumptions. Proceedings of the National Academy of Sciences, 106, 19644–19650. https://doi.org/10.1073/pnas.0901637106
Yackulic CB (2017) Competitive exclusion over broad spatial extents is a slow process: evidence and implications for species distribution modeling. Ecography, 40, 305–313. https://doi.org/10.1111/ecog.02836
The recommender in charge of the evaluation of the article and the reviewers declared that they have no conflict of interest (as defined in the code of conduct of PCI) with the authors or with the content of the article. The authors declared that they comply with the PCI rule of having no financial conflicts of interest in relation to the content of the article.
-
Reviewed by Joaquín Calatayud, 13 Apr 2021
The authors did an excellent job in addressing my comments. I have nothing else to add.
Reviewed by Carsten Dormann, 11 Apr 2021
The revised version of this manuscript addresses all points I made on the previous draft.
Again, I think this is a very good paper and would happily accept it for publication if I were in a position to do so.
Well done!
Evaluation round #1
DOI or URL of the preprint: https://www.biorxiv.org/content/10.1101/2020.12.07.414326v1
Author's Reply, 03 Mar 2021
Dear Joaquin Hortal,
Thank you for evaluating the manuscript and thereby offering us the opportunity to submit an improved version. We now have taken into account and/or discussed the concerns raised by the two reviewers. Following remarks made by other colleagues, we have also slightly modified some parts of the introduction. We hope that you will find our revised version suitable for recommendation.
Looking forward to your response
On behalf of all coauthors,
Regards,
Benoit Facon
Decision by Joaquín Hortal, posted 19 Jan 2021
This is really a superb piece of science; I join both reviewers in their congratulations for one of the best JSDM studies I've seen so far. Really good data, good design, and top-notch interpretation of the results. We all three liked it a lot.
All that said, both reviewers have a number of concerns about the current version of your work. Most of them are minor comments and/or related to clarity, but Carsten Dormann raises a good point about the potential bias in the selection of model parameters caused by the focus on the best models that you may want to consider. As he admits, this is mainly a phylosophical question, but if your study is going to set up a higher standard in JSM, a better account of the uncertainty in model selection would help building a stronger discipline.
Also, both reviewers point to the possibility that there may be some spatial and temporal structure in your data that could be due to unaccounted-for processes or factors. I believe that an additional assessment of whether there is some structure remaining in the residuals of the association matrix could be informative about the existence or not of other processes. Indeed, Joaquín Calatayud highlights that these structured effects may be negligible once you account for environment and co-existence processes, but you need to at least discuss that - and having supplementary analyses to support such discussion would round up your work.
In any case, this is really a great work, and I am looking forward for the resubmission of a new version that accounts and/or discuss the concerns raised by both reviewers. If their comments are properly addressed or discussed it is most likely that I can recommend your preprint in PCI Ecology.
Reviewed by Joaquín Calatayud, 13 Jan 2021
This is a brilliant piece of science: well written, carefully conducted, based on an outstanding dataset, using a thorough and sophisticated methodology, and presenting timely and very exciting results. I really enjoyed reading it! I have only few very minor questions and suggestions.
I could only fully understand the abstract after reading the completed manuscript. I would suggest rewording small details so that the abstract is clearer. Some points where I found difficulties:
“Community structure was mainly determined by…” Here, I found “community structure” to be somehow vague. Moreover, in a previous sentence you mentioned that network inference was used. After reading this, I was expecting you characterized the community structure via network properties, which is not the case. This may nevertheless be a matter of personal bias, but I guess others may have the same problem. I would suggest to change “community structure” by “species abundances”.
“The relative importance of these factors was mildly modulated by host plants.” This sentence was also difficult to follow to me without reading the full manuscript. I would say something like: “The relative importance of these factors mildly varied when we used particular host plant groups” o something alike. This may be again a matter of taste.
“… specialists and generalists flies almost behaved as separate communities…” I found “behaved as separate communities” difficult to understand here and when mentioned throughout the text. I would try to use a term more clearly connected with the results. Again a matter of taste and totally up to the authors to follow this suggestion.
In the second paragraph of the introduction, it may be worth mentioning that facilitation can also occur between phytophagous arthropods (e.g. Godinho et al. 2016. Oecologia 180: 161-167.)
“Since species interactions mostly occur in/on plant organs, they may be modulated by plant species identity…” Here, it is not totally clear whether “species interactions” refers to intraguild interactions or to fly-plant interactions.
Are the 8 species used all the species of Tephritidae present in the island? If so, I would explicitly state it. If not, I think it would be worth mentioning in the discussion the potential influence of other unevaluated species in the abundance of the used species and model outputs.
“Of the 12872 initial samples, only those with GPS coordinates, with at least one individual fly and belonging to one of the 21 host…” As a layman in the modelling used, it is not clear to me why you didn’t use the 0s (i.e. the samples without individuals). Moreover, how many host plants are there in the island? If there are much more than the 21 used, how do you think this could affect subsequent interpretations of assembly mechanisms? This might deserve a line in the discussion.
“… all previous models were reevaluated on the datasets excluding D. ciliatus (Models 2-0 to 2-6)” To facilitate the reading I would say: “… all previous models were reevaluated on the datasets excluding the species lacking fundamental host use estimates (D. ciliatus ; Models 2-0 to 2-6)” or something similar.
“Among plants inferred as possible hosts from species abundance patterns (i.e., those with high coefficient values), coefficients correlated positively with fly laboratory-measured fitness for specialists but not for generalists (Figure 3B)” I found this result super interesting! Still, I would better justify why you only used the plants with high coefficients. Moreover, while the modelling approach used seems evident, I would explicitly specify it (perhaps in the figure caption), explaining also the meaning of the shadowed areas in Fig. 3B. Finally, could this result be mostly driven by only one generalist and/or specialist species?
“Accounting for environmental covariates strongly improved model fit and made all residual covariances almost completely vanish, particularly among groups, suggesting that no important environmental factor structuring the community has been missed”. Completely agree. Yet, your data is temporally and spatially structured, which might contain interesting information on assembly mechanisms (e.g. the effects of dispersal processes and interannual and/or seasonal dynamics not linked to climate). While testing this may be out of the scope of your work, I also think that it would deserve a line in the discussion. At least, to avoid criticisms from autocorrelation purists, I would explicitly mention that your results suggest that the influence of temporal and spatial autocorrelation is negligible (besides the influence of other important environmental factors).
While I really enjoyed “The ghost of competition past” section in the discussion, I’m not totally sure the term perfectly fits here, at least as described by Connell (1980). To my knowledge Connell was referring to the coevolution of competitors and thus to evolutionary changes in the fundamental niches. That is, I agree that by nicely comparing realized and fundamental niches you detected a “ghost of competition”, but I would rather say that it is a current (ecological) ghost rather than a past (evolutionary) one. Perhaps it would be worth to add a few lines explaining this, mentioning also that eco-evolutionary approaches are required to truly address changes in fundamental niches due to competition and their consequences to the assembly of species.
Finally, regarding also the fundamental niches, by looking at figures 3A and 1B, it seems that in some situations flies are able to colonize plant species in which they show a very reduced fitness (close to 0, if not 0). I kept wondering how it is this possible. Perhaps, there are differences in host use among (sub)populations and fundamental niche estimates are based on individuals of a reduced number of (sub)populations. I’m just not sure, but this would perhaps deserve a brief mention in the discussion.
Hope this is of any help and congratulations for this excellent study!
Joaquín Calatayud
Reviewed by Carsten Dormann, 11 Dec 2020
Facon et al.: Joint species distributions reveal the combined effects of host plants, …
This study investigates fruit fly communities on different host fruits on la Réunion over an 18 year period. Abundances of fruit flies were predicted using both climatic variables and host plant species in a joint species distribution model. This approach yields correlations among fruit fly species as a side-effect (the variance-covariance matrix of the residuals of the k x k species, also sometimes called the association matrix), which may represent species interactions.
Accounting for environment, the association matrix became almost diagonal, indicating no apparent associations among fruit fly species. This is in contrast to the raw-data association matrix, which showed a strong difference in host use between generalists and specialists. The distinction between generalists and specialists was based on a fly x host laboratory choice experiment (published elsewhere by members of the same group). In the discussion, the authors carefully interpret the few remaining (all negative) correlations in the residuals as indication of competition among some generalists and some specialists. In particular, they expand on the problem of not seeing many competitive interactions due to evolution having led to niche displacement among similar species (aka the ghost of competition past).
Overall, I find this one of the best jSDM studies I have seen in the literature so far. The relatively small number of species (only fruit fly species) and the huge number of observations (5000 samples with nearly 100,000 individuals) is hard to improve upon. Of course, there are always a few things that remain unclear (at least to me). For example, competition is likely to leave an effect only when resources are scarce, which requires either high population densities of the flies, and/or low availability of fruits. The authors do not provide data on either. Thus, for any given sample, it is unclear whether we would actually expect any trace of competition.
Also I am not very impressed by the model selection approach used, although admittedly this is almost a philosophical issue and their practice is in line with common analytical strategies (more on this below).
I am particularly happy to see the substantial effect of relatively coarse environmental predictors on the association matrix. It could be argued that the small remaining covariance could actually be explained by other predictors, such as acidity of the fruit or something like that. If so, no covariance would indicate no competition, expounding the problem of witnessing the outcome of hundreds of generations undergoing niche separation without being able to see the selection in action. Only extensive laboratory studies with monospecific and paired flies over generations could test for a widening and hence overlapping host niches when released from the invisible competition.
As far as observation studies go, I don’t think there is anything more we could ask for.
Model selection bias
I wouldn’t quite call it a secret, but it seems that the well-known effect of model selection causing a bias in model parameter estimates is unknown to ecologists. If, however, we are interested in model parameters, not only their prediction, we need to be aware of this. In a nutshell, the selection of models leads to a final (few) “best models”, which are then interpreted statistically (“significant effects”) and their parameters are estimated alongside their standard error. Now, since the computer does not “know” that this best model is the result of investigating dozens to hundreds of models on the same data before, it naively assumes this is the only model fitted to the data. It “ignores” the model selection uncertainty that results from variability of the data. As a result, the standard errors are too small, as they do not take into account the model selection procedure’s uncertainty. Also, the estimates are biased, as all models that show non-significant effects of this predictor are removed through model selection, making the remaining models more likely to have large (absolute) estimates than small.
It is relatively simple to show this through simulation ([http://joshualoftus.com/post/model-selection-bias-invalidates-significance-tests]), but Harrell (2001, Springer: Regression Modeling Strategies) writes about it, and it is found (as obvious introductory statement) in [https://pubmed.ncbi.nlm.nih.gov/18189162] or “well known” in [https://academic.oup.com/aje/article/167/5/523/212182]. Parameter shrinkage has been advocated as strategy to counteract the selection bias (see last link for review).
Now, in the present study I do not actually think that model selection bias is a big issue: the data set is large (reducing the problem of model selection leading to different models during bootstrapping); also, the BIC difference between models is very large, indicating little ambiguity in the model ranking. Still, I would have preferred a presentation of few fuller models than the model selection outcome (as indeed Burnham & Anderson themselves argue for).
Model diagnostics
I did not find any statements on how well the model structure meets distributional assumptions and independence of residuals. While I guess that the PLN approach is relatively robust to overdispersion, I think it can be expected of the authors to provide a statement on whether the data were actually well modelled assuming a Poisson distribution. My experience is that fast reproducing and flying beasts, such as fruit flies, tend to clump, requiring a negative binomial to represent the variance in the data. (The DHARMa package may not readily work on this model type, but the principle applies and the authors should be able to simulate the residuals themselves, as demonstrated in that package’s vignette.)
Similarly, the spatial nature of the sampling warrants an assessment of spatial autocorrelation in the model residuals. In fact, it could be that the remaining association signal can be partly explained by spatial effects.