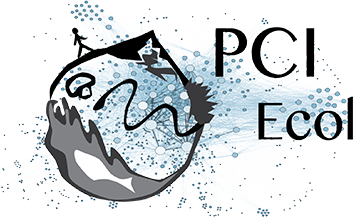
Combining epidemiological models with statistical inference can detect parasite interactions
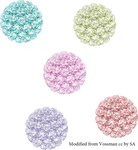
Detecting within-host interactions using genotype combination prevalence data
Abstract
Recommendation: posted 03 October 2018, validated 10 October 2018
Brisson, D. (2018) Combining epidemiological models with statistical inference can detect parasite interactions. Peer Community in Ecology, 100006. 10.24072/pci.ecology.100006
Recommendation
There are several important topics in the study of infectious diseases that have not been well explored due to technical difficulties. One such topic is pursued by Alizon et al. in “Modelling coinfections to detect within-host interactions from genotype combination prevalences” [1]. Both theory and several important examples have demonstrated that interactions among co-infecting strains can have outsized impacts on disease outcomes, transmission dynamics, and epidemiology. Unfortunately, empirical data on pathogen interactions and their outcomes is often correlational making results difficult to decipher.
The analytical framework developed by Alizon et al. [1] infers the presence and strength of pathogen interactions through their impact on transmission dynamics using a novel application of Approximate Bayesian Computation (ABC)-regression to epidemiological data. Traditional analytic approaches identify pathogen interactions when the observed distribution of pathogens among hosts differ from ‘neutral’ expectations. However, deviations from this expectation are not only a result of inter-strain interactions but can be caused by many ecological interactions, such as heterogeneity in host contact networks. To overcome this difficulty, Alizon et al [1] develop an analytical framework that incorporates explicit epidemiological models to allow inference of interactions among strains of Human Papillomaviruses (HPV) even with other ecological interactions that impact the distribution of strains among hosts. Alizon et al also demonstrate that using more of the available data, including the specific combination of strains present in hosts and knowledge of the connectivity of the hosts (i.e., super-spreaders), leads to more accurate inferences of the strength and direction of within-host interactions among coinfecting strains. This method successfully identified data generated from models with high and moderate inter-strain interaction intensity when the host population was homogeneous and was only slightly less successful when the host population was heterogeneous (super-spreaders present). By comparison, some previously published analytical methods could identify only some inter-strain interactions in datasets generated from models with homogeneous host populations, but host heterogeneity obscured these interactions.
This manuscript makes seamless connections between basic viral biology and its epidemiological consequences by tying them together with realistic models, illustrating the fundamental utility of biological modeling. This analytical framework provides crucial tools for experimentalists, facilitating collaborations with theoreticians to better understand the epidemiological consequences of co-infections. In addition, the method is simple enough to be applied by a broad base of experimentalists to the many pathogens where co-infections are common. Thus, this paper has the potential to impact several research fields and public health practice. Those attempting to apply this method should note the potential limitations noted by the authors. For example, it is not designed to detect the mechanisms of inter-strain interactions (there is no within host component of the models) but to identify the existence of interactions through patterns indicative of these interactions while ruling out other sources that could cause the pattern. This approach is likely to be most accurate when strain identification within hosts is precise and unbiased - which is unlikely in many systems where samples are taken only from symptomatic cases and strain detection is not sufficiently sensitive – and when host contact networks can be reasonably estimated. Importantly, a priori knowledge of the set of possible epidemiological models is needed for accurate parameter estimates, which may be true for several prominent pathogens, but not be so for many other pathogens and symbionts. We look forward to future extensions of this framework where this restriction is relaxed. Alizon et al. [1] have provided a framework that will facilitate theoretical and empirical work on the impact of coinfections on infectious disease and should shape future public health data collection standards.
References
[1] Alizon, S., Murall, C.L., Saulnier, E., & Sofonea, M.T. (2018). Detecting within-host interactions using genotype combination prevalence data. bioRxiv, 256586, ver. 3 peer-reviewed and recommended by PCI Ecology. doi: 10.1101/256586
The recommender in charge of the evaluation of the article and the reviewers declared that they have no conflict of interest (as defined in the code of conduct of PCI) with the authors or with the content of the article. The authors declared that they comply with the PCI rule of having no financial conflicts of interest in relation to the content of the article.
no declaration
Reviewed by Samuel Díaz Muñoz, 06 Sep 2018
This new revision is much improved and very readable. I anticipate it will allow a broad readership to appreciate and understand all the work that went into this paper and its significance within and beyond the field.
The responses to the review comments were thorough and straightforward, with the authors being refreshingly candid about the limitations of the study. The response also directly addressed the reviewer questions, providing reviewers with more confidence on their interpretations and improving the quality of our suggestions so the readership can understand the findings. In this way, the revision became a dialogue between peers, which is what peer review should ideally be.
The authors must be commended for being very receptive to reviewer comments and performing a dramatic reorganization of the paper. There are still competing demands of journal formatting requirements that can get in the way of the clearest presentation of science, but the text now strongly favors the latter.
I am happy to recommend this paper. I reiterate the statement in my initial review: this paper continues to push modeling towards embracing the full range of interactions among coinfecting viruses, which empirical studies are increasingly uncovering. In doing so, it provides crucial tools for experimentalists to focus their efforts and collaborate with theoreticians to better understand coinfections and their epidemiological consequences. This paper will be an outstanding contribution to the epidemiological literature and to the emerging coinfection (viral and parasite) research community.
Below I include some final minor comments and wording suggestions.
Abstract Suggestion: Parasite genetic diversity can provide information on disease transmission dynamics but most methods ignore the exact combinations of genotypes in infections.
Suggestion (check if intended meaning is preserved): Using genital infections by different types of Human Papillomaviruses (HPVs) as a test case, we show that within-host parasite interactions can be detected from epidemiological data and that this detection is robust even in the face of host heterogeneity in behaviour.
Lines 3-5 “Over the last decades,…”: Elegant and very useful statement to contextualize the paper!
Line 9 - “in the following text.” OR “which we hereafter refer to as the ‘genotype combination’.”
Line 17 - “..exceptions..”
Line 67 - “..for reasons other than the nature of the genotype(s) infecting them.”
Line 119-115 - Excellent summary.
Line 244 - Good clarification for the reader.
Line 370 - “Overall, removing the heterogeneity in the data due to differences in host behaviour does increase our ability to detect competitive interactions.”
Reviewed by Erick Gagne, 31 Aug 2018
The authors have done a substantial revision of the text that has greatly improved the manuscript. Particularly, they well addressed the clarity of writing which now guides the reader more clearly through the purpose and goals of the modeling. This is an important contribution to the literature and the model does an impressive job of starting to untangle the complicated issues with co-infections.
I have a remaining comment pertaining to figure 5. Although I think the addition of figure 5 is helpful, it appears to me that the decrease in error with increased sampling is only pronounced with the all summary statistics. The statements regarding this are a bit overstated, and in fact, a valid conclusion is that although increased sample sizes reduce the error the effects are minimal. The paper correctly identifies that using all summary statistics has the most pronounced effect.
Minor edit:
Line 12: “to any system of multiple infectious by different parasites”
Evaluation round #1
DOI or URL of the preprint: 10.1101/256586
Version of the preprint: 1
Author's Reply, 27 Jul 2018
Dear reviewers,
we would like to thank you for your detailed evaluation of our manuscript and the numerous suggestions.
Based on these, we have greatly modified the manuscript. In particular, we now consider scenario without host behavioral heterogeneity. This allows us to show that existing methods perform reasonably well in such a setting and also that our method is robust to (limited) model mispecification.
We thank you in advance for your time and look forward hearing back from you.
Best regards, - Samuel
Decision by Dustin Brisson, posted 27 Jul 2018
Editor type comments
Two experts and I have reviewed the preprint entitled “Modelling coinfections to detect within-host interactions from genotype combination prevalences” and all have come to similar conclusions. First, all of us appreciate the topic and think that the work itself has value. There were several noted areas where additional analyses would improve the impact, although most of these were minor. The primary area that the authors should focus on to improve the manuscript is on the presentation. All of the reviewers believed that the much of the work was under-presented (not enough detail), ambiguously described, and in several areas confusing. It is important to note that none of the reviewers identified all or even most of the areas where the presentation needs alteration, instead noting some examples of the types of presentation that caused confusion. Globally, we found this an important piece of work and hope to see an improved version in the near future. Below you will find my personal review for what it is worth. Dustin
Review
There are several important academic areas in the study of infectious diseases that have not been well explored, often because of technical difficulty. The topic of the current work, “Modelling coinfections to detect within-host interactions from genotype combination prevalences,” is certainly one of the. Both theory and several important examples have demonstrated that co-infection dynamics can have outsized impacts on epidemiology. Unfortunately, for the outcome of pathogen interactions are difficult to decipher from correlational data. The current work aims to identify the impacts of pathogen interactions on transmission dynamics using epidemiological data. This is important and would be a major advance. The primary issue with the current manuscript is in the presentation, which I found to be ambiguous in some places and confusing in others. A potential issue is that you have assumed that I am smarter than I am, but I should point out that those like me will be the primary audience of papers that introduce analytical approaches for empirical data. I would suggest more hand holding in the presentation. Below I try to provide some examples to help guide the resubmission. At the end, I also point out some areas where additional analyses could be useful.
First, the method section must come before the results and discussion as one must read the methods to make sense of the results For example, parameter set #3 is referred to in the results without a description of what that is and why it may be important. Additionally, there are definitions that are in the methods that are needed to understand the results (ie “target runs”, “competition intensity”).
Second, in many descriptions there is an absence of explicit purpose which seems to affect the structure of the manuscript. That is, it is not always clear what is trying to be accomplished with each section and several sections that is necessary. This is true for the overall paper as well. By the end it is somewhat that the purpose is to introduce and validate a method to assess transmission dynamics from epidemiological data given potential coinfections (this is my inference anyway), but it is difficult to infer this from the abstract or the introduction. Another potential point or subpoint is that there is information that cannot be used by other methods so you have less precise results. Anyway, being more explicit throughout will help structure the manuscript and help the reader.
One of the major issues seems to be a lack of precision in the descriptions, again throughout. Take the first paragraph of the Discussion as an example (“This is due to the fact that when sharing a host, parasites can interact in various ways [42]. The goal of this study was to determine to what extent the prevalence of parasite combinations can inform us on such interactions.”). It is hard to get a solid footing on what the interactions are, what is meant by parasite combinations, and what information you are looking for. Similarly, better descriptions of the figures in the text, especially with regard to what the reader is supposed to learn from them, is essential. For example, Fig 3 is intense but it is never explicitly stated what the take home message of the figure is. I am also not clear on what the numbers in the circles of Fig 2A are or what is meant by “different prevalences” mean. The methods are overall pretty clear, but more hand-holding would be helpful. For example, the summary statistics used seem important – spending greater time describing them and what we learn from the different types would be useful. In addition, as the ABC is the centerpiece, a fuller description is probably warranted.
Some comments on the science itself.
The ABC is used to infer parameters of the model, but what if you assume the incorrect underlying model, is it substantially worse than the other heuristic methods where there are fewer assumptions. I would like to see what occurs if you assumptions about the epidemiology are incorrect.
Can you explain how “we assumed that interactions between 303 HPV types take place through the recovery rates.” affects either model or biological inference? I think you may mean how coinfection affects clearance rates, but I still cannot quite figure out how this impacts the interpretation.
It is not clear what happens to the clearance rates if there are 3 strains present. That is, is recovery (1+k)^2 or is it still 1+k.
I don’t understand why a in (2) denotes assortment between host types rather than within host types What is the upper case delta in the updated master equation (w/ two host classes)?
Some other things to consider
Why the paper is centered on HPV is not clear to me. It applies to many potential pathogens and you did not actually use any data from HPV.
The sentence describing 6D is confusing.
A definition of what a significant association between parasites is should be explicitly stated in the results section for each test. Currently it is a bit confusing “Depending on whether k is greater or lower than 1, we expect host classes containing genotypes from the second group to be under- or over-represented respectively” - why is there not a positive correlation between interaction intensity and the probability that the test is significant for the combination network?
Reviewed by anonymous reviewer 1, 12 Mar 2018
This paper presents a model along with methods to infer the strength and direction of within-host interactions of coinfecting parasites from epidemiological data. The manuscript generates simulated data sets using an explicit epidemiological model, of the SIS type that allows multiple parasite genotypes and cotransmission (unlike previous models). The text implements previously published tests on data generated from the SIS model and then proceeds to implement an ABC regression to infer interactions between parasite genotypes. The key results are that 1) using an mechanistic epidemiological model improves the inference of previous heuristic methods, but that their ability to infer parasite interactions are limited in the presence of host heterogeneity and 2) that including information on the parasite genotype combinations in addition to rank (i.e. genotypes per host) and host heterogeneity (i.e. superspreaders or not) greatly improves inference of within host interactions from epidemiological data. I note that my expertise in epidemiological theory is very limited and I cannot assess the technical merit of the models. Therefore, I deliver this review from the perspective of an (very interested) experimentalist.
This paper tackles a very interesting subject matter. As someone that studies viral coinfections as a main focus, it is really exciting to see that viral interactions can, in theory, be inferred from epidemiological data. Moreover, it is very gratifying to see that there are researchers trying to do just that. In certain sections, the manuscript does a very good job of explaining the methods and results, but these are sometimes not in the right place within the manuscript (see Major comments below). The paper takes a very even-handed and judicious approach, by giving proper credit/inclusion to prior work and opting to model data that can be realistically obtained, respectively. The paper represents another leap forward in rebooting epidemiological modeling to include the complexities of viral coinfection.
This manuscript could be greatly improved by guiding the reader more through the results and their biological interpretation, given the selected Intro/Results/Discussion/Methods format. In particular, the results section should include brief, basic methodological explanations and state the biological interpretation of results to guide readers. The most important example of this is the nature of the viral interaction being modeled (see Major Comment #2). The paper has a number of findings that are of great relevance to clinicians and experimentalists, but they are not mentioned in the discussion. I think this undersells the impact of this work beyond epidemiological modeling. Additionally, there are some issues with the figures that warrant attention.
Overall, I find the work to be highly interesting and potentially impactful, but the text hard to follow in some places. Providing more clarity will allow the paper to highlight and communicate these exciting results. This paper makes seamless connections between basic viral biology and its epidemiological consequences, tying them together with realistic models, thus illustrating the fundamental utility of biological modeling. More generally, I think this paper continues to push modeling towards embracing the full range of interactions among coinfecting viruses, which empirical studies are increasingly uncovering. In doing so, it provides crucial tools for experimentalists to focus their efforts and collaborate with theoreticians to better understand coinfections and their epidemiological consequences. With some revisions, this paper will be an outstanding contribution to the epidemiological literature and to the emerging coinfection (viral and parasite) research community.
Major comments: 1. This paper follows an Introduction/Results format, which usually is used to appeal to a broader readership by guiding readers through the results including the most essential background on methodological procedures. However, a lot of this basic information is not present in the results, but appears in the methods. These essential bits of information should be included in the results, even if they make the Results text longer, because they serve to guide the reader that is outside the subfield that is unlikely to peruse the methods in detail.
There are many examples of brief, elegant explanations that in the methods text that would have greatly aided the understanding of the results. A few examples of these explanations in the methods that should be in the results:
-Line 323 First sentence -Lines 334-337 -A summary of lines 352-370, especially the three sets of summary statistics and the rationale for selecting them. -Line 303-306 (see #2 below) -Lines 341-346, especially the statement “...simulating many datasets, for which by definition the underlying parameters are known, and comparing them to the target dataset the parameters of which we want to estimate.”
- The interactions between the genotypes that are a focus of this study were initially expressed as “...we assume that any interaction between HR and LR types takes place through the recovery rate.” Upon first reading it was not clear to me what was meant by this statement. I believe it corresponds to the following example given in the methods: “e.g. how the presence of genotype A affect the clearance rate of genotype B.”
It is very, very important that this crucial interaction (the main focus of the study!) be crystal clear to the reader, upon first mention - more so with such a good, brief example explanation provided already in the methods.
There are some inconsistencies in how terms or parameters are mentioned in the text versus the figures. Rectifying these inconsistencies can greatly improve the readability of the paper. See comments below on Figures S1, 4, 5, and 6.
The discussion seemed very centered around the modeling, but contained little information for epidemiologists or experimentalists. This despite the fact that the model results have practical implications that could advance research; for instance, the need for sensitive testing that distinguishes multiple HPV types.
The use of grayscale in several graphs limited contrast and hindered the interpretations offered in the text. I suggest different shapes or colors as alternatives (see below for specific comments).
Minor Comments: Line 49 - “specific functional response” is vague and leaves the reader guessing. Response of the host to the parasite? Response of the parasite to coinfection? I gather from the references cited that the text is attempting to refer to biological interactions broadly speaking, but not sure.
Line 56 - Suggestion: "This is consistent with a key result of the study, which identifies the ‘number of lifetime sex..."
Line 95 - “have a competitive advantage (or disadvantage) when competing with non-oncogenic types”
Since this is a hypothesis, it is very confusing for some readers to put in parentheses the opposite prediction within the same statement.
Line 97 - “interaction between HR and LR types takes place through the recovery rate.”
A little explanation of the biological implication of the interaction occurring through the recovery rate would greatly aid readers not steeped in epidemiology. I presume that this assumption means that the interaction between HR and LR occurs via the immune system as the host will have been infected and recovered before infection with the next type. It is important to have this point be clear because it goes to one of the key aspects of the paper, the interaction between the viruses.
Line 110 - “These have been tested by generating distributions but without any epidemiological model.”
A brief description about how these distributions were generated would guide the reader. For example - Were the distributions generated using a statistical distribution? This would aid readers not familiar with the literature.
Figure 3 - The grayscale is very difficult to distinguish.
Line 117 - This paragraph would benefit from a wrap up sentence that explains the statistical result in light of what is being investigated. What does “most combinations lead to significant tests” mean? My understanding is that with a 1k sample size (and more so with a 5k sample size) this test can detect interactions even when their intensity is low.
Line 118 - “the positive association between interaction intensity and test significance”. Again, what this statistical finding means should be outlined. I would presume that if interaction intensity is high, it would be more easily detected by any test. So ideally one would want a test that could deliver significant results even at a low interaction intensity, correct?
Line 122 - Brief explanation of connectance may be beneficial to the reader. Most readers will understand ChiSq significance pretty intuitively (as a departure of expected values, which suggests an interaction between the parasite types), but connectance in this context may be less familiar to many readers.
Upon reading the methods there is an elegant and brief explanation of connectance that should be included in this section of the text: “that is the proportion of observed edges relative to the number of edges. Here, individuals are connected if they share the same 336 parasite (parasite network) or the same combination of parasites (combination network).” Similarly, the association screening approach explanation from the methods can be also be placed in this section.
Line 122-135 - Combination network (earlier called coinfection combination network) and parasite network are dropped in this paragraph with no definition whatsoever. My understanding until reading this statement was that the data being discussed were parasite coinfection combinations.
Figure S1 - The text mentions this figure depicts the “correlation between interaction intensity and the prevalence of each host combination”, but the figure states it has the “Correlation between interaction intensity and combination, rank or genotype prevalence”. Presumably, Class# corresponds to host combination, Rank# corresponds to rank, and Tot# corresponds to genotype prevalence. These labels should be clearly indicated in the figure legend and ideally would correspond to the names in the text, i.e. Prev# for genotype prevalence and Combination# for host combination.
Line 141 - This sentence should be reworded to avoid the use of prediction twice: “The fraction of predictions that match our expectation is generally close” OR “The fraction of correct predictions is generally close” (Also sentence needs a period)
Line 144 - contact structure is sufficient to blur the effect of within-host interactions OR is sufficient to blur the ability of this test to detect within host interactions? I thought the contact structure and within-host interactions are set (or at least constant) in these simulated data sets, no?
Line 148 - k should be defined in the text at first appearance. I see k is defined in Figure 5, but this text is referencing Figure 4, where k is not defined either.
Line 149-152 and Figure 4 - The grayscale that indicates the different ranks cannot be distinguished (even on a high resolution screen), making it difficult to see the clear pattern described in the text. This is a really important figure in the paper so the ranks should be more distinct. Different symbols would work well if non-color figures are desired.
Line 154 - Suggestion: “... same prevalence in single infection (see rank 1 data points).”
Line 157 - At the end of the paragraph, the text states the goal is to infer parameter k, but at the beginning of the paragraph there are two graphs with known k parameters. I did not understand this discrepancy. Is it that the first model doesn’t have HR and LR genotypes? Some clarification will aid the reader. Upon reading the methods, the statement on line 343 greatly clarifies this paragraph: “It consists in simulating many datasets, for which by definition the underlying parameters are known, and comparing them to the target dataset the parameters of which we want to estimate.” Including this statement in the results would broaden accessibility of the text to readers outside the field. This is a general comment throughout the manuscript that I outline in Major Comments.
Line 162 - TYPO: “We assessed the performance of..” (no ’s’ after performance)
Line 165 - This paragraph, together with the figure is well written and the results are very clear.
Line 175 - It is not clear to me what is meant by “runs”. Readers may benefit from a little more explanation regarding how analyses differ.
Line 198 - If “proportion of errors” in the text is the same as “error probability” in Figure 6D, as I believe it is, the text and figure should match each other for increased clarity.
Line 228 - These seem like important results, that (contrary to the statement) are being reported in the discussion and should be included in the results. Moreover, they are important results that justify the focus of the paper on k, which increases the accuracy of inference more than many other parameters. Minimally they should be included in a supplement.
Line 303 - Again, this statement “interactions between HPV types take place through the recovery rates” is not very clear in biological terms to me. Now reading further, if this statement: “how the presence of genotype A affect the clearance rate of genotype B” is what is meant, this should be stated in the methods and the main text. This is a crucial explanation of the biological process that is being modeled and is not clear in the text.