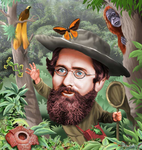
BRISSON Dustin
- Evolution and Ecology of Disease Systems Laboratory, University of Pennsylvania, Philadelphia, United States of America
- Dispersal & Migration, Eco-evolutionary dynamics, Evolutionary ecology, Host-parasite interactions, Human impact, Microbial ecology & microbiology, Molecular ecology, Parasitology, Phylogeny & Phylogeography, Population ecology
- recommender
Recommendation: 1
Reviews: 0
Recommendation: 1
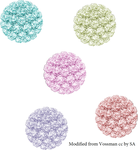
Detecting within-host interactions using genotype combination prevalence data
Combining epidemiological models with statistical inference can detect parasite interactions
Recommended by Dustin Brisson based on reviews by Samuel Díaz Muñoz, Erick Gagne and 1 anonymous reviewerThere are several important topics in the study of infectious diseases that have not been well explored due to technical difficulties. One such topic is pursued by Alizon et al. in “Modelling coinfections to detect within-host interactions from genotype combination prevalences” [1]. Both theory and several important examples have demonstrated that interactions among co-infecting strains can have outsized impacts on disease outcomes, transmission dynamics, and epidemiology. Unfortunately, empirical data on pathogen interactions and their outcomes is often correlational making results difficult to decipher.
The analytical framework developed by Alizon et al. [1] infers the presence and strength of pathogen interactions through their impact on transmission dynamics using a novel application of Approximate Bayesian Computation (ABC)-regression to epidemiological data. Traditional analytic approaches identify pathogen interactions when the observed distribution of pathogens among hosts differ from ‘neutral’ expectations. However, deviations from this expectation are not only a result of inter-strain interactions but can be caused by many ecological interactions, such as heterogeneity in host contact networks. To overcome this difficulty, Alizon et al [1] develop an analytical framework that incorporates explicit epidemiological models to allow inference of interactions among strains of Human Papillomaviruses (HPV) even with other ecological interactions that impact the distribution of strains among hosts. Alizon et al also demonstrate that using more of the available data, including the specific combination of strains present in hosts and knowledge of the connectivity of the hosts (i.e., super-spreaders), leads to more accurate inferences of the strength and direction of within-host interactions among coinfecting strains. This method successfully identified data generated from models with high and moderate inter-strain interaction intensity when the host population was homogeneous and was only slightly less successful when the host population was heterogeneous (super-spreaders present). By comparison, some previously published analytical methods could identify only some inter-strain interactions in datasets generated from models with homogeneous host populations, but host heterogeneity obscured these interactions.
This manuscript makes seamless connections between basic viral biology and its epidemiological consequences by tying them together with realistic models, illustrating the fundamental utility of biological modeling. This analytical framework provides crucial tools for experimentalists, facilitating collaborations with theoreticians to better understand the epidemiological consequences of co-infections. In addition, the method is simple enough to be applied by a broad base of experimentalists to the many pathogens where co-infections are common. Thus, this paper has the potential to impact several research fields and public health practice. Those attempting to apply this method should note the potential limitations noted by the authors. For example, it is not designed to detect the mechanisms of inter-strain interactions (there is no within host component of the models) but to identify the existence of interactions through patterns indicative of these interactions while ruling out other sources that could cause the pattern. This approach is likely to be most accurate when strain identification within hosts is precise and unbiased - which is unlikely in many systems where samples are taken only from symptomatic cases and strain detection is not sufficiently sensitive – and when host contact networks can be reasonably estimated. Importantly, a priori knowledge of the set of possible epidemiological models is needed for accurate parameter estimates, which may be true for several prominent pathogens, but not be so for many other pathogens and symbionts. We look forward to future extensions of this framework where this restriction is relaxed. Alizon et al. [1] have provided a framework that will facilitate theoretical and empirical work on the impact of coinfections on infectious disease and should shape future public health data collection standards.
References
[1] Alizon, S., Murall, C.L., Saulnier, E., & Sofonea, M.T. (2018). Detecting within-host interactions using genotype combination prevalence data. bioRxiv, 256586, ver. 3 peer-reviewed and recommended by PCI Ecology. doi: 10.1101/256586