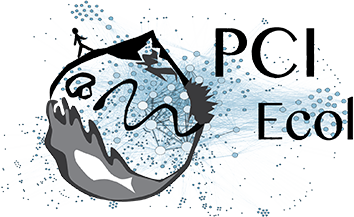
Uncovering the ecology in big-data by hierarchizing multi-scale environmental effects

Hierarchizing multi-scale environmental effects on agricultural pest population dynamics: a case study on the annual onset of Bactrocera dorsalis population growth in Senegalese orchards
Abstract
Recommendation: posted 13 June 2024, validated 14 June 2024
Vercken, E. (2024) Uncovering the ecology in big-data by hierarchizing multi-scale environmental effects. Peer Community in Ecology, 100634. 10.24072/pci.ecology.100634
Recommendation
Along with the generalization of open-access practices, large, heterogeneous datasets are becoming increasingly available to ecologists (Farley et al. 2018). While such data offer exciting opportunities for unveiling original patterns and trends, they also raise new challenges regarding how to extract relevant information and actually improve our knowledge of complex ecological systems, beyond purely descriptive correlations (Dietze 2017, Farley et al. 2018).
In this work, Caumette et al. (2024) develop an original ecoinformatics approach to relate multi-scale environmental factors to the temporal dynamics of a major pest in mango orchards. Their method relies on the recent tree-boosting method GPBoost (Sigrist 2022) to hierarchize the influence of environmental factors of heterogeneous nature (e.g., orchard composition and management; landscape structure; climate) on the emergence date of the oriental fruit fly, Bactrocera dorsalis. As boosting methods allows the analysis of high-dimensional data, they are particularly adapted to the exploration of such datasets, to uncover unexpected, potentially complex dependencies between ecological dynamics and multiple environmental factors (Farley et al. 2018). In this article, Caumette et al. (2024) make a special effort to guide the reader step by step through their complex analysis pipeline to make it broadly understandable to the average ecologist, which is no small feat. I particularly welcome this commitment, as making new, cutting-edge analytical methods accessible to a large community of science practitioners with varying degrees of statistical or programming expertise is a major challenge for the future of quantitative ecology.
The main result of Caumette et al. (2024) is that temperature and humidity conditions both at the local and regional scales are the main predictors of B. dorsalis emergence date, while orchard management practices seem to have relatively little influence. This suggests that favourable climatic conditions may allow the persistence of small populations of B. dorsalis over the dry season, which may then act as a propagule source for early re-infestations. However, as the authors explain, the resulting regression model is not designed for predictive purposes and should not at this stage be used for decision-making in pest management. Its main interest rather resides in identifying potential key factors favoring early infestations of B. dorsalis, and help focusing future experimental field studies on the most relevant levers for integrated pest management in mango orchards.
In a wider perspective, this work also provides a convincing proof-of-concept for the use of boosting methods to identify the most influential factors in large, multivariate datasets in a variety of ecological systems. It is also crucial to keep in mind that the current exponential growth in high-throughput environmental data (Lucivero 2020) could quickly come into conflict with the need to reduce the environmental footprint of research (Mariette et al. 2022). In this context, robust and accessible methods for extracting and exploiting all the information available in already existing datasets might prove essential to a sustainable pursuit of science.
References
Caumette C, Diatta P, Piry S, Chapuis M-P, Faye E, Sigrist F, Martin O, Papaïx J, Brévault T, Berthier K. 2024. Hierarchizing multi-scale environmental effects on agricultural pest population dynamics: a case study on the annual onset of Bactrocera dorsalis population growth in Senegalese orchards. bioRxiv 2023.11.10.566583, ver. 3 peer-reviewed and recommended by Peer Community in Ecology. https://doi.org/10.1101/2023.11.10.566583
Dietze MC. 2017. Ecological Forecasting. Princeton University Press
Farley SS, Dawson A, Goring SJ, Williams JW. 2018. Situating Ecology as a Big-Data Science: Current Advances, Challenges, and Solutions. BioScience, 68, 563–576, https://doi.org/10.1093/biosci/biy068
Lucivero F. 2020. Big Data, Big Waste? A Reflection on the Environmental Sustainability of Big Data Initiatives. Science and Engineering Ethics 26, 1009–1030. https://doi.org/10.1007/s11948-019-00171-7
Mariette J, Blanchard O, Berné O, Aumont O, Carrey J, Ligozat A-L, Lellouch E, Roche P-E, Guennebaud G, Thanwerdas J, Bardou P, Salin G, Maigne E, Servan S, Ben-Ari T 2022. An open-source tool to assess the carbon footprint of research. Environmental Research: Infrastructure and Sustainability, 2022. https://dx.doi.org/10.1088/2634-4505/ac84a4
Sigrist F. 2022. Gaussian process boosting. The Journal of Machine Learning Research, 23, 10565-10610. https://jmlr.org/papers/v23/20-322.html
The recommender in charge of the evaluation of the article and the reviewers declared that they have no conflict of interest (as defined in the code of conduct of PCI) with the authors or with the content of the article. The authors declared that they comply with the PCI rule of having no financial conflicts of interest in relation to the content of the article.
The authors declare that they have received no specific funding for this study
Evaluation round #1
DOI or URL of the preprint: https://doi.org/10.1101/2023.11.10.566583
Version of the preprint: 2
Author's Reply, 24 May 2024
Decision by Elodie Vercken, posted 21 Feb 2024, validated 21 Feb 2024
Dear Authors,
Thank you very much for submitting your work for recommendation in PCI Ecology. Your manuscript has been evaluated by 2 reviewers and myself. We all concur that the subject of the study is of definite interest for the management of fruit fly populations, while the methods are likely to be of interest to a wider community of field ecologists.
However, in its current state, the manuscript is oriented more towards methodological issues and the use of innovative statistical methods, and seems to be aimed at an audience with a certain degree of statistical expertise. We all feel that the manuscript would benefit from some degree of rewriting to allow a wider audience of ecologists to understand the rationale of the analyses, even if they don’t intend to replicate the methods.
I would be most willing to recommend this work for PCI Ecology, provided you can address the concerns raised by the different evaluators.
Please pay particular attention to the following points that appear most critical for improvement of the manuscript:
- more detailed ecological context in the introduction
- justification of the use of male trapping data to infer pest pressure in orchards
- justification of the interest of the developed method relative to other existing methods
- justification of the 500-replication of each data point
- use of independent data for cross-validation
- avoid statistical jargon as much as possible, always try to explain the principle of each analysis before going into technical details of the method, explain the results not only in statistical terms but also in biological/ecological ones
Don’t hesitate to ask if any of the reviewers’ comments are unclear to you, or if I can help in any way. I sincerely hope our comments are helpful to you and contribute to improving the manuscript further.
Recommender’s comments:
1/ My main concern is related to the resampling 500 times each estimate of t0. The distributions are rather narrow (see Figure 4), with an average range of 0.7 weeks, which is inferior to the unit of temporal resolution. I would think intuitively that it does create pseudo-replication within the dataset. One of the reviewer was surprised with this approach as well, so please try to clarify this point.
2/ I am also puzzled by the partitioning of the 500 replicates are partitioned into building and training datasets, as they are not independent and values are all really close : basically you use more or less the same date for both steps, which does not really count as validation. You could rather use different orchards, picked from the different biogeographic areas.
3/ There are about 200 data points, nested within 69 orchards. How can you be sure of having enough power to test the combined effects of about 30 explanatory variables?
4/ What do you use the Popfit model if you are only interested in the initial growth phase? Would a simple, exponential-type model be enough?
5/ Could you identify on Figure 3 which were the data points that were excluded from the analysis?
6/ On Fig 7, the gam-smoothed curves seem overfitted. How did you choose the fitting parameters you used? Have you tried different degrees of constraint on the fitting? Please give more details in the text on the fitting method.
7/ You interpret the influence of LU13 landscape class as possibly arising from re-infestation from urban micro-gardens. If this is the case, I would expect the LU4 class (orchards) to also stand out in the analysis?
Reviewed by Kévin Tougeron
, 12 Jan 2024
Review for PCI Ecology: Hierarchizing multi-scale environmental effects on agricultural pest population dynamics: a case study on the annual onset of Bactrocera dorsalis population growth in Senegalese orchards
General comments
In the introduction, it would be great to have a bit more description of the ecology of the fruit fly; how many generation per year, is there a dormancy phase at any moment of the cycle, what is the phenology of the species, how long is the development time, what are the main and alternative food sources, what are the main natural enemies and competitors in Senegal, etc.
As regards to the analyses carried out, I do not have the necessary expertise to judge the relevance of the methods used. I do, however, find that the part concerning the methods is well described and makes it easy to understand what was done.
The capture method of BD flies is the use of pheromones to attract males. Yet, females and eggs are the main issue for orchards. I wonder if there is any proof that male and female abundances are well correlated. If not, it could be an issue regarding the selected method.
I find the results section quite hard to follow, especially as the methods used and the metrics calculated are not the most common. Care should be taken to avoid statistical jargon as much as possible, and to explain the results obtained in biological terms. For example, without going into interpretation, what does it mean that SHAP values correlate with PCA Axis 3 values?
This also applies to the discussion, which is—for some parts—still quite complex to understand. It is interesting to discuss the new methods put forward in this article, but the authors should also take care to make their article accessible to a wider audience of ecologists and entomologists, as the subject covered by the article is also highly applied to biological control.
Specific comments
L37: It is not clear in the abstract what “Gradient boosting” is. I am not sure the term should appear here.
L57-58: It may be an issue but is has been largely addressed in agroecology studies in the past decade. I would suggest to temper a bit this statement.
L53-59: The term “spatio-temporal heterogeneity” should appear in this paragraph.
L89: Please give full taxonomic description and authority of B. dorsalis
L96-98: Has this hypothesis been formulated anywhere else in a research paper or technical report before?
L107: Precise what you mean by “environmental features”
L128: In Fig 2, does the “orchard” land cover only represent mango orchards?
L143: So the time serie is at a daily scale, right?
L154: What threshold did you chose to asses if the model was poorly fitted to the data?
L168: It is not clear; are the orchards all mixed-species orchards?
L178-179: At some point it would be necessary to mention the name of the species other than mango that can host the fly and their respective phenology.
L192-199: This method is interesting and well-described.
L268: How so, exactly?
L269-273: I am not convinced that Figure 4 is essential to present here in the main document. It could be put in supplementary material. The figure is quite difficult to understand and does not bring much added value to the text.
L344 and onwards: What is the potential role of temperature variations along with humidity? In phenological models that are usually constructed for temperate regions, temperature is usually the main factor affecting insect pullulation and early arrival in the fields. It's understandable that humidity is a major factor here, but how is it linked or correlated to temperature?
L365 and onwards: NDWI is generally related to canopy density, but also to the semi-natural elements present around the orchard. The buffering effect of certain elements on temperature or humidity has already been demonstrated, as has the effect on crop pests and their natural enemies, and would certainly merit further consideration in this discussion.
Is the NDWI calculation a reliable source for measuring a microclimatic effect on the scale of an orchard plot?
L390: Could a link be made here with other insect models, such as Drosophila suzukii for instance?
L397-401: What about the potential role of refuges or suitable habitats for natural enemies of the fly?
L405: Is there any mango variety known to better resist BD?
L454-464: Those limitations are worth being mentioned, but maybe not at the very end of the discussion. The previous paragraph (L442-453) would fit better as a conclusion paragraph.
Reviewed by Jianqiang Sun
, 07 Feb 2024
I have written below about some of the questions/comments/suggestions I had when I read this manuscript.
Authors developed a flexible analysis pipeline to hierarchize the effects of multiscale env variables on the timing of annual BD population growth. However, there is a lack of validation of the methodology. The authors should indicate how much better the developed method is compared to existing methods/pipelines. Is the performance of conventional methods (e.g., random forests, LASSO) definitely lower than the proposed method?
Finally, for each orchard and year, 500 values of ...[L154]: I couldn't catch "500 values of the estimated t0 (L154)". The data consists of 69 orchards over 3 years. To my understanding, at most 69*3=207 models can be obtained from all data. Please explain simply here.
results clearly suggest that humidity conditions are the primary driver ... [L345]: Is the humidity the primary factor? Is it possible that pests start to increase in line with the time of year when fruit starts to ripen? Is there a pseudo-correlation between the time of fruit ripening and the time of increased humidity, with increased humidity leading to an increase in pests? What happens when humidity is removed from the model? Does prediction performance deteriorate significantly?
Please consider using cross-validation for time-series data. For example, training with 2012 and validating with 2013, training with 2012-2013 and validating with 2014.
Sahelian climate [L132]: If possible, please visualize some important meteorological data (e.g., temperature, precipitation, humidity) with charts from 2011 to 2014 or the average of three years (better to merge in Figure 3). This may help readers who are not familiar with Sahelian climate to easily understand the characters of climate variables.
Fig 3 [L148]: Please consider using jittered points over boxplot (or use violin plot) to visualize the data density.