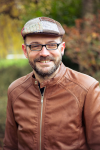
SUEUR Cédric
- Institut Pluridisciplinaire Hubert Curien, Université de Strasbourg, Strasbourg, France
- Behaviour & Ethology, Coexistence, Epidemiology, Evolutionary ecology, Host-parasite interactions, Interaction networks, Preregistrations, Social structure, Zoology
- recommender
Recommendations: 3
Reviews: 0
Recommendations: 3
Flexible reproductive seasonality in Africa-dwelling papionins is associated with low environmental productivity and high climatic unpredictability
Reproductive flexibility shapes primate survival in a changing climate driven by environmental unpredictability
Recommended by Cédric Sueur based on reviews by 2 anonymous reviewersAs seasonal cycles become increasingly disrupted, our understanding of the ecology and evolution of reproductive seasonality in tropical vertebrates remains limited (Bronson 2009). To predict how changes in seasonality might impact these animals, it is crucial to identify which elements of their varied reproductive patterns are connected to the equally varied patterns of rainfall seasonality (within-year fluctuations) or the significant climatic unpredictability (year-to-year variations) characteristic of the intertropical region.
Dezeure et al. (2024) provide a comprehensive examination of reproductive seasonality in papionin monkeys across diverse African environments. By investigating the ecological and evolutionary determinants of reproductive timing, the authors offer novel insights into how climatic factors, particularly environmental unpredictability, shape reproductive strategies in these primates. This study stands out not only for its methodological rigour but also for its contribution to our understanding of how primates adapt their reproductive behaviours to varying environmental pressures. The findings have broad implications, particularly in the context of ongoing climate change, which is expected to increase environmental unpredictability globally. The innovative approach of this paper lies in its multifaceted examination of reproductive seasonality, which integrates data from 21 wild populations of 11 papionin species. The study employs a robust statistical framework, incorporating Bayesian phylogenetic generalised linear mixed models to control for phylogenetic relatedness among species. This methodological choice is crucial because it allows the authors to disentangle the effects of environmental variables from evolutionary history, providing a more accurate picture of how current ecological factors influence reproductive strategies.
The study’s focus on environmental unpredictability as a determinant of reproductive seasonality is particularly noteworthy. While previous research has established the importance of environmental seasonality (Janson and Verdolin 2005), this paper breaks new ground by showing that the magnitude of year-to-year variation in rainfall – rather than just the seasonal distribution of rainfall – plays a critical role in determining the intensity of reproductive seasonality. This finding is supported by the significant negative correlation between reproductive seasonality and environmental unpredictability, which the authors demonstrate across multiple populations and species. The results of this study are important for several reasons. First, they challenge the traditional view that reproductive seasonality is primarily driven by within-year environmental fluctuations. By showing that inter-annual variability in rainfall is a stronger predictor of reproductive timing than intra-annual variability, the authors suggest that primates, like papionins, have evolved flexible reproductive strategies to cope with the unpredictable availability of resources. This flexibility is likely an adaptive response to the highly variable environments that many African primates inhabit, where food availability can vary dramatically not just within a year but from year to year. Second, the study highlights the role of reproductive flexibility in the evolutionary success of papionins. The authors provide compelling evidence that species within the Papio genus, for example, exhibit significant variability in reproductive timing both within and between populations. This variability suggests that these species possess a remarkable ability to adjust their reproductive strategies in response to local environmental conditions, which may have contributed to their widespread distribution across diverse habitats in Africa. This finding aligns with the work of Brockman and Schaik (2005), who argued that reproductive flexibility is a key factor in the success of primates in unpredictable environments.
The study also contributes to our understanding of the evolutionary transition from seasonal to non-seasonal breeding in primates. The authors propose that the loss of strict reproductive seasonality in some papionin species may represent an adaptive shift toward greater reproductive flexibility. This shift could be driven by the need to maximise reproductive success in environments where the timing of resource peaks is difficult to predict. The authors’ findings support this hypothesis, as they show that populations living in more unpredictable environments tend to have lower reproductive seasonality. The broader implications of this study (Dezeure et al. 2024) extend beyond the specific case of papionin monkeys. The findings have relevance for the study of reproductive strategies in other long-lived, tropical mammals that face similar environmental challenges. As climate change is expected to increase the frequency and intensity of environmental unpredictability, understanding how species have historically adapted to such conditions can provide valuable insights into their potential resilience or vulnerability to future changes.
Many primate species are already facing significant threats from habitat loss, hunting, and climate change. By identifying the environmental factors that influence reproductive success, Dezeure et al. (2024) study can help inform conservation strategies aimed at protecting the most vulnerable populations. For example, conservation efforts could focus on maintaining or restoring habitat features that promote reproductive flexibility, such as access to a variety of food resources that peak at different times of the year (Chapman et al.).
References
Brockman D, Schaik C (2005) Seasonality in Primates: Studies of Living and Extinct Human and Non-Human Primates. Cambridge University Press. https://doi.org/10.1017/CBO9780511542343
Bronson FH (2009) Climate change and seasonal reproduction in mammals. Philos Trans R Soc B Biol Sci 364:3331–3340. https://doi.org/10.1098/rstb.2009.0140
Chapman CA, Gogarten JF, Golooba M, et al Fifty+ years of primate research illustrates complex drivers of abundance and increasing primate numbers. Am J Primatol n/a:e23577. https://doi.org/10.1002/ajp.23577
Jules Dezeure, Julie Dagorrette, Lugdiwine Burtschell, Shahrina Chowdhury, Dieter Lukas, Larissa Swedell, Elise Huchard (2024) Flexible reproductive seasonality in Africa-dwelling papionins is associated with low environmental productivity and high climatic unpredictability. bioRxiv, ver.2 peer-reviewed and recommended by PCI Ecology https://doi.org/10.1101/2024.05.01.591991
Janson C, Verdolin J (2005) Seasonality of primate births in relation to climate. In: Schaik CP van, Brockman DK (eds) Seasonality in Primates: Studies of Living and Extinct Human and Non-Human Primates. Cambridge University Press, Cambridge, pp 307–350 https://doi.org/10.1017/CBO9780511542343.012
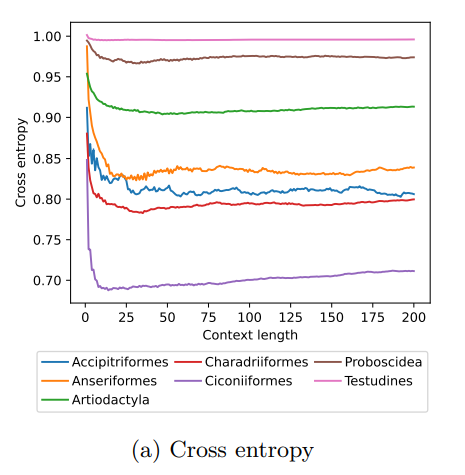
MoveFormer: a Transformer-based model for step-selection animal movement modelling
A deep learning model to unlock secrets of animal movement and behaviour
Recommended by Cédric Sueur based on reviews by Jacob Davidson and 1 anonymous reviewerThe study of animal movement is essential for understanding their behaviour and how ecological or global changes impact their routines [1]. Recent technological advancements have improved the collection of movement data [2], but limited statistical tools have hindered the analysis of such data [3–5]. Animal movement is influenced not only by environmental factors but also by internal knowledge and memory, which are challenging to observe directly [6,7]. Routine movement behaviours and the incorporation of memory into models remain understudied.
Researchers have developed ‘MoveFormer’ [8], a deep learning-based model that predicts future movements based on past context, addressing these challenges and offering insights into the importance of different context lengths and information types. The model has been applied to a dataset of over 1,550 trajectories from various species, and the authors have made the MoveFormer source code available for further research.
Inspired by the step-selection framework and efforts to quantify uncertainty in movement predictions, MoveFormer leverages deep learning, specifically the Transformer architecture, to encode trajectories and understand how past movements influence current and future ones – a critical question in movement ecology. The results indicate that integrating information from a few days to two or three weeks before the movement enhances predictions. The model also accounts for environmental predictors and offers insights into the factors influencing animal movements.
Its potential impact extends to conservation, comparative analyses, and the generalisation of uncertainty-handling methods beyond ecology, with open-source code fostering collaboration and innovation in various scientific domains. Indeed, this method could be applied to analyse other kinds of movements, such as arm movements during tool use [9], pen movements, or eye movements during drawing [10], to better understand anticipation in actions and their intentionality.
References
1. Méndez, V.; Campos, D.; Bartumeus, F. Stochastic Foundations in Movement Ecology: Anomalous Diffusion, Front Propagation and Random Searches; Springer Series in Synergetics; Springer: Berlin, Heidelberg, 2014; ISBN 978-3-642-39009-8.
https://doi.org/10.1007/978-3-642-39010-4
2. Fehlmann, G.; King, A.J. Bio-Logging. Curr. Biol. 2016, 26, R830-R831.
https://doi.org/10.1016/j.cub.2016.05.033
3. Jacoby, D.M.; Freeman, R. Emerging Network-Based Tools in Movement Ecology. Trends Ecol. Evol. 2016, 31, 301-314.
https://doi.org/10.1016/j.tree.2016.01.011
4. Michelot, T.; Langrock, R.; Patterson, T.A. moveHMM: An R Package for the Statistical Modelling of Animal Movement Data Using Hidden Markov Models. Methods Ecol. Evol. 2016, 7, 1308-1315.
https://doi.org/10.1111/2041-210X.12578
5. Wang, G. Machine Learning for Inferring Animal Behavior from Location and Movement Data. Ecol. Inform. 2019, 49, 69-76.
https://doi.org/10.1016/j.ecoinf.2018.12.002
6. Noser, R.; Byrne, R.W. Change Point Analysis of Travel Routes Reveals Novel Insights into Foraging Strategies and Cognitive Maps of Wild Baboons. Am. J. Primatol. 2014, 76, 399-409.
https://doi.org/10.1002/ajp.22181
7. Fagan, W.F.; Lewis, M.A.; Auger‐Méthé, M.; Avgar, T.; Benhamou, S.; Breed, G.; LaDage, L.; Schlägel, U.E.; Tang, W.; Papastamatiou, Y.P. Spatial Memory and Animal Movement. Ecol. Lett. 2013, 16, 1316-1329.
https://doi.org/10.1111/ele.12165
8. Cífka, O.; Chamaillé-Jammes, S.; Liutkus, A. MoveFormer: A Transformer-Based Model for Step-Selection Animal Movement Modelling. bioRxiv 2023, ver. 4 peer-reviewed and recommended by Peer Community in Ecology.
https://doi.org/10.1101/2023.03.05.531080
9. Ardoin, T.; Sueur, C. Automatic Identification of Stone-Handling Behaviour in Japanese Macaques Using LabGym Artificial Intelligence. 2023, https://doi.org/10.13140/RG.2.2.30465.02402
10. Martinet, L.; Pelé, M. Drawing in Nonhuman Primates: What We Know and What Remains to Be Investigated. J. Comp. Psychol. Wash. DC 1983 2021, 135, 176-184, doi:10.1037/com0000251.
https://doi.org/10.1037/com0000251
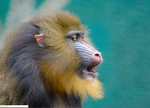
A reply to “Ranging Behavior Drives Parasite Richness: A More Parsimonious Hypothesis”
Does elevated parasite richness in the environment affect daily path length of animals or is it the converse? An answer bringing some new elements of discussion
Recommended by Cédric Sueur based on reviews by 2 anonymous reviewersIn 2015, Brockmeyer et al. [1] suggested that mandrills (Mandrillus sphinx) may accept additional ranging costs to avoid heavily parasitized areas. Following this paper, Bicca-Marques and Calegaro-Marques [2] questioned this interpretation and presented other hypotheses. To summarize, whilst Brockmeyer et al. [1] proposed that elevated daily path length may be a consequence of elevated parasite richness, Bicca-Marques and Calegaro-Marques [2] viewed it as a cause. In this current paper, Charpentier and Kappeler [3] respond to some of the criticisms by Bicca-Marques and Calegaro-Marques and discuss the putative parsimony of the two competing scenarios. The manuscript is interesting and focuses on an important question concerning the discussion about the social organization and home range use in wild mandrills. This answer helps to move this debate forward and should stimulate more empirical studies of the role of environmentally-transmitted parasites in shaping ranging and movement patterns of wild vertebrates. Given the elements this paper brings to the topics, it should have been published in American Journal of Primatology, the journal that published the two previous articles.
References
[1] Brockmeyer, T., Kappeler, P. M., Willaume, E., Benoit, L., Mboumba, S., & Charpentier, M. J. E. (2015). Social organization and space use of a wild mandrill (Mandrillus sphinx) group. American Journal of Primatology, 77(10), 1036–1048. doi: 10.1002/ajp.22439
[2] Bicca-Marques, J. C., & Calegaro-Marques, C. (2016). Ranging behavior drives parasite richness: A more parsimonious hypothesis. American Journal of Primatology, 78(9), 923–927. doi: 10.1002/ajp.22561
[3] Charpentier, M. J., & Kappeler, P. M. (2018). A reply to “Ranging Behavior Drives Parasite Richness: A More Parsimonious Hypothesis.” ArXiv:1805.08151v2 [q-Bio]. Retrieved from http://arxiv.org/abs/1805.08151