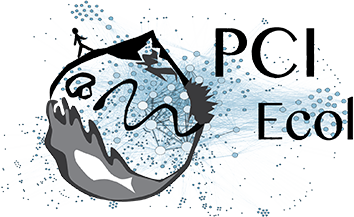
Are condition indices positively related to each other and to fitness?: a test with grackles
Validating morphological condition indices and their relationship with reproductive success in great-tailed grackles
Abstract
Recommendation: posted 12 December 2019, validated 18 December 2019
Mendez, M. (2019) Are condition indices positively related to each other and to fitness?: a test with grackles. Peer Community in Ecology, 100035. 10.24072/pci.evolbiol.100035
Recommendation
Reproductive succes, as a surrogate of individual fitness, depends both on extrinsic and intrinsic factors [1]. Among the intrinsic factors, resource level or health are considered important potential drivers of fitness but exceedingly difficult to measure directly. Thus, a host of proxies have been suggested, known as condition indices [2]. The question arises whether all condition indices consistently measure the same "inner state" of individuals and whether all of them similarly correlate to individual fitness. In this preregistration, Berens and colleagues aim to answer this question for two common condition indices, fat score and scaled mass index (Fig. 1), using great-tailed grackles as a model system. Although this question is not new, it has not been satisfactorily solved and both reviewers found merit in the attempt to clarify this matter.
Figure 1. Hypothesized relationships between two condition indices and reproductive success. Single arrow heads indicate causal relationships; double arrow heads indicate only correlation. In a best case scenario, all relationships should be positive and linear.
A problem in adressing this question with grackles is limited population, ergo sample, size and limited possibilites of recapture individuals. Some relationships can be missed due to low statistical power. Unfortunately, existing tools for power analysis fall behind complex designs and the one planned for this study. Thus, any potentially non significant relationship has to be taken cautiously. Nevertheless, even if grackles will not provide a definitive answer (they never meant to do it), this preregistration can inspire broader explorations of matches and mismatches across condition indices and species, as well as uncover non-linear relationships with reproductive success.
References
[1] Roff, D. A. (2001). Life history evolution. Oxford University Press, Oxford.
[2] Labocha, M. K.; Hayes, J. P. (2012). Morphometric indices of body condition in birds: a review. Journal of Ornithology 153: 1–22. doi: 10.1007/s10336-011-0706-1
The recommender in charge of the evaluation of the article and the reviewers declared that they have no conflict of interest (as defined in the code of conduct of PCI) with the authors or with the content of the article. The authors declared that they comply with the PCI rule of having no financial conflicts of interest in relation to the content of the article.
Evaluation round #2
DOI or URL of the preprint: https://github.com/corinalogan/grackles/blob/master/Files/Preregistrations/gcondition.Rmd
Version of the preprint: of the 28 August 2019
Author's Reply, 11 Nov 2019
Dear Dr.’s Marcos Mendez and Javier Seoane,
Thank you very much for taking a second look at this submission and providing more super helpful feedback! We are happy to have the opportunity to revise and resubmit. We responded to your comment below.
Our preregistration is at http://corinalogan.com/Preregistrations/gcondition.html. Note that the version-tracked version of this preregistration is in rmarkdown at GitHub: https://github.com/corinalogan/grackles/blob/master/Files/Preregistrations/gcondition.Rmd. In case you want to see the history of track changes for this document at GitHub, click the previous link and then click the “History” button on the right near the top. From there, you can scroll through our comments on what was changed for each save event and, if you want to see exactly what was changed, click on the text that describes the change and it will show you the text that was replaced (in red) next to the new text (in green).
Many thanks for your generous feedback throughout this process!
All our best,
Jennifer, Corina, Melissa, Luisa and Kelsey
Validating morphological condition indices and their relationship with reproductive success in great-tailed grackles
Jennifer M. Berens, Corina J. Logan, Melissa Folsom, Luisa Bergeron, Kelsey B. McCune
http://corinalogan.com/Preregistrations/gcondition.html version v1.8
Submitted by Kelsey McCune 2019-08-05 20:05
Abstract
Morphological variation among individuals has the potential to influence multiple life history characteristics such as dispersal, migration, reproductive fitness, and survival (Wilder, Raubenheimer, and Simpson (2016)). Theoretically, individuals that are in better “condition” (i.e. fat reserves, Labocha and Hayes (2012)) should be able to disperse or migrate further or more successfully, have greater reproductive fitness, and survive for longer (Wilder, Raubenheimer, and Simpson (2016)). Researchers have used a variety of morphological proxy variables to quantify condition (i.e., fat score, weight, ratio of weight to tarsus length, ratio of weight to wing chord length, Labocha, Schutz, and Hayes (2014)), however, there is mixed support regarding whether these proxy variables relate to life history characteristics (Wilder, Raubenheimer, and Simpson (2016); Labocha, Schutz, and Hayes (2014)). Additionally, although some researchers use multiple morphological proxy variables for condition (i.e. Warnock and Bishop (1998)), rarely has there been direct comparisons among proxies to validate that they measure the same trait. In this investigation, we will compare two condition proxies (fat score and the ratio of weight to tarsus length) to validate whether they measure the same trait in our study system, the great-tailed grackle (Quiscalus mexicanus). We will then test whether our morphological proxy variables correlate with reproductive success, measured as whether a female had a fledgling or not and whether a male held a territory containing nests or not. Results will improve our understanding of measures of condition in grackles, and birds in general, and the importance of condition for reproductive success - a necessary component for selection to act.
Keywords: birds, great-tailed grackles, condition indices, reproductive success
Round #2
Your recommendation
by Marcos Mendez, 2019-11-03 09:01
Manuscript: http://corinalogan.com/Preregistrations/gcondition.html version v1.8
Decision on "Validating morphological condition indices and their relationship with reproductive success in great-tailed grackles"
Dear Dr. Berens and coauthors, I am glad to inform you that your preprint has now been accepted by PCI Ecology. Together with this letter you will find the final comments by one of the reviewers. Sincerely,
Marcos Méndez
Author response: thank you so much! We are very excited to hear this great news!
Reviews
Reviewed by Javier Seoane, 2019-11-01 16:45
I really think the authors have improved the preprint following the reviewers' suggestions with clearer rationale, methods and explanations.
As for response 3: "Response 3: You are correct that so far the range of fat scores is narrow, and we like the suggestion to consider it a factor instead of a count. Consequently, we have changed the family specification in our model for P1 to “ordinal”. We are unclear on what you mean by ANOVA-like linear model, though. Could you please provide us with further clarification if you think this is the more appropriate model?"
I just meant to consider the response variable as a factor such as 'low fat score' (meaning Kaiser's scores from 0 to 1) vs 'high fat scores' (meaning scores from 2 to 3). I agree that you may use an ordinal model for P1, which is better but more difficult to build and interpret.
Author response:
Thank you for clarifying your comment - that makes sense. We checked the data we have so far and there are only seven data points where fat score is 2 or 3 (and 3 was the highest recorded so far). It seems like this might be too small of a subset to rigorously analyze. We decided to stick with the change we made in the first revision by changing the variable to ordinal, especially because you appear to agree with this change. We will make sure to be careful about the interpretation of the model. Thank you again!
Decision by Marcos Mendez, posted 07 Nov 2019
Dear Dr. Berens and coauthors
Thank you for your answers and modifications. Some minor revisions are proposed by one of the referees (see these criticisms below). Once these changes have been taken into account, I am ready to recommend this preregistration for PCI Ecology and I will send my recommendation text to the managing board.
Yours sincerely,
Marcos Méndez.
PS:Additional message from the managing board
We ask you to modify your article according to this list of modifications:
Mandatory modifications.
As indicated in the 'How does it work?’ section and in the code of conduct, please make sure that:
-Authors have no financial conflict of interest relating to the article. The article must contain a "Conflict of interest disclosure" paragraph before the reference section containing this sentence: "The authors of this preprint declare that they have no financial conflict of interest with the content of this article." If appropriate, this disclosure may be completed by a sentence indicating that some of the authors are PCI recommenders: “XXX is one of the PCI XXX recommenders.”
In order to reach a better referencing and greater visibility of your recommended paper, we suggest you to do the following modifications :
(i) add the following sentence in the acknowledgements: "Version XX of this ms has been peer-reviewed and recommended by Peer Community In Ecology (https://doi.org/10.24072/pci.ecology.100035) »
Optional modifications.
==> Third, (if you wish) we advise you to use templates (word docx template and a latex template) to format your preprint in a PCI style. This is optional. Here is the links of the templates:
https://peercommunityin.org/templates/
Please be careful to correctly update all text in these templates (doi, authors’ names, address, title, date, recommender first name and family name …). Please be careful to also choose the badge “Open Code” if appropriate (in addition to the “Open access”, “Open data” and “Open Peer-Review” badges).
Indicate in the “cite as” box the version of the article that you are currently formatting. This should be version 3.
If some of the reviewers are anonymous, indicate for example “Albert Ayler and two anonymous reviewers”.
Reviewed by Javier Seoane, 01 Nov 2019
I really think the authors have improved the preprint following the reviewers' suggestions with clearer rationale, methods and explanations.
As for response 3: "Response 3: You are correct that so far the range of fat scores is narrow, and we like the suggestion to consider it a factor instead of a count. Consequently, we have changed the family specification in our model for P1 to “ordinal”. We are unclear on what you mean by ANOVA-like linear model, though. Could you please provide us with further clarification if you think this is the more appropriate model?"
I just meant to consider the response variable as a factor such as 'low fat score' (meaning Kaiser's scores from 0 to 1) vs 'high fat scores' (meaning scores from 2 to 3). I agree that you may use an ordinal model for P1, which is better but more difficult to build and interpret.
Evaluation round #1
DOI or URL of the preprint: https://github.com/corinalogan/grackles/blob/master/Files/Preregistrations/gcondition.Rmd
Version of the preprint: of the 5 August 2019
Author's Reply, 30 Oct 2019
Dear Dr.’s Marcos Mendez and Javier Seoane, Thank you very much for taking a second look at this submission and providing more super helpful feedback! We are happy to have the opportunity to revise and resubmit. We responded to your comment below. Our preregistration is at http://corinalogan.com/Preregistrations/gcondition.html. Note that the version-tracked version of this preregistration is in rmarkdown at GitHub: https://github.com/corinalogan/grackles/blob/master/Files/Preregistrations/gcondition.Rmd. In case you want to see the history of track changes for this document at GitHub, click the previous link and then click the “History” button on the right near the top. From there, you can scroll through our comments on what was changed for each save event and, if you want to see exactly what was changed, click on the text that describes the change and it will show you the text that was replaced (in red) next to the new text (in green). Many thanks for your generous feedback throughout this process! All our best, Jennifer, Corina, Melissa, Luisa and Kelsey
Validating morphological condition indices and their relationship with reproductive success in great-tailed grackles Jennifer M. Berens, Corina J. Logan, Melissa Folsom, Luisa Bergeron, Kelsey B. McCune http://corinalogan.com/Preregistrations/gcondition.html version v1.8 Submitted by Kelsey McCune 2019-08-05 20:05 Abstract Morphological variation among individuals has the potential to influence multiple life history characteristics such as dispersal, migration, reproductive fitness, and survival (Wilder, Raubenheimer, and Simpson (2016)). Theoretically, individuals that are in better “condition” (i.e. fat reserves, Labocha and Hayes (2012)) should be able to disperse or migrate further or more successfully, have greater reproductive fitness, and survive for longer (Wilder, Raubenheimer, and Simpson (2016)). Researchers have used a variety of morphological proxy variables to quantify condition (i.e., fat score, weight, ratio of weight to tarsus length, ratio of weight to wing chord length, Labocha, Schutz, and Hayes (2014)), however, there is mixed support regarding whether these proxy variables relate to life history characteristics (Wilder, Raubenheimer, and Simpson (2016); Labocha, Schutz, and Hayes (2014)). Additionally, although some researchers use multiple morphological proxy variables for condition (i.e. Warnock and Bishop (1998)), rarely has there been direct comparisons among proxies to validate that they measure the same trait. In this investigation, we will compare two condition proxies (fat score and the ratio of weight to tarsus length) to validate whether they measure the same trait in our study system, the great-tailed grackle (Quiscalus mexicanus). We will then test whether our morphological proxy variables correlate with reproductive success, measured as whether a female had a fledgling or not and whether a male held a territory containing nests or not. Results will improve our understanding of measures of condition in grackles, and birds in general, and the importance of condition for reproductive success - a necessary component for selection to act. Keywords: birds, great-tailed grackles, condition indices, reproductive success
Round #2
Your recommendation by Marcos Mendez, 2019-11-03 09:01 Manuscript: http://corinalogan.com/Preregistrations/gcondition.html version v1.8 Decision on "Validating morphological condition indices and their relationship with reproductive success in great-tailed grackles" Dear Dr. Berens and coauthors, I am glad to inform you that your preprint has now been accepted by PCI Ecology. Together with this letter you will find the final comments by one of the reviewers. Sincerely, Marcos Méndez Author response: thank you so much! We are very excited to hear this great news!
Reviews Reviewed by Javier Seoane, 2019-11-01 16:45 I really think the authors have improved the preprint following the reviewers' suggestions with clearer rationale, methods and explanations. As for response 3: "Response 3: You are correct that so far the range of fat scores is narrow, and we like the suggestion to consider it a factor instead of a count. Consequently, we have changed the family specification in our model for P1 to “ordinal”. We are unclear on what you mean by ANOVA-like linear model, though. Could you please provide us with further clarification if you think this is the more appropriate model?" I just meant to consider the response variable as a factor such as 'low fat score' (meaning Kaiser's scores from 0 to 1) vs 'high fat scores' (meaning scores from 2 to 3). I agree that you may use an ordinal model for P1, which is better but more difficult to build and interpret.
Author response: Thank you for clarifying your comment - that makes sense. We checked the data we have so far and there are only seven data points where fat score is 2 or 3 (and 3 was the highest recorded so far). It seems like this might be too small of a subset to rigorously analyze. We decided to stick with the change we made in the first revision by changing the variable to ordinal, especially because you appear to agree with this change. We will make sure to be careful about the interpretation of the model. Thank you again!
Round #1
Dear Dr.’s Marcos Mendez, Javier Seoane, and Isabel López-Rull, We greatly appreciate the time you have taken to give us such useful feedback! We are very thankful for your willingness to participate in the peer review of preregistrations, and we are happy to have the opportunity to revise and resubmit. We revised our preregistration and associated files at http://corinalogan.com/Preregistrations/gcondition.html, and we responded to your comments below. Note that the version-tracked version of this preregistration is in rmarkdown at GitHub: https://github.com/corinalogan/grackles/blob/master/Files/Preregistrations/gcondition.Rmd. In case you want to see the history of track changes for this document at GitHub, click the previous link and then click the “History” button on the right near the top. From there, you can scroll through our comments on what was changed for each save event and, if you want to see exactly what was changed, click on the text that describes the change and it will show you the text that was replaced (in red) next to the new text (in green). We think the revised version is much improved due to your generous feedback! All our best, Jennifer, Corina, Melissa, Luisa and Kelsey
Validating morphological condition indices and their relationship with reproductive success in great-tailed grackles Jennifer M. Berens, Corina J. Logan, Melissa Folsom, Luisa Bergeron, Kelsey B. McCune
Comment 0: Dear Dr. Berens and coauthors, Your preprint has been carefully reviewed by two experts and myself. Both experts find value in your proposal but suggest several modifications and additions. I concur with their suggestions and therefore the current version cannot be recommended, but I invite you to submit a revised version that incorporates the suggestions made by the reviewers. In particular, the new version should pay attention to a proper definition of condition, to consider additional literature in the abstract, suggested by one of the reviewers. The election of the two conditions indexes addressed should be motivated, as one of the experts suggests that CMI may be more adequate. Consider to reframe or reword your hypotheses, to distinguish them from your predictions. Clarify the current and future sample size (I add here that even if 57 individuals are finally available, splitting the data into male and female grackles still may be a relatively low sample size), and the repeatability of the measures. In the statistical analysis, the new version should clarify how repeated measures (expectedly few) will be handled, correct some mistakes in the models (Poisson rather than binomial for testing H1) and, given your limited sample size, I may add that a power analysis would be advisable. Details are provided in the comments by the reviewers. I look forward to a new version of the proposal. Sincerely, Marcos Méndez
Response 0: Thank you Dr. Mendez for the time you committed to reviewing and handling our preregistration. We address below the reviewers’ helpful comments regarding clarification of our definition of condition, using the CMI, our hypotheses and predictions, sample size, repeatability and the errors in the statistical analysis. We found the additional literature the reviewers suggested to be particularly appropriate and enlightening, so we have added those citations. Per your suggestion, we’ve also added a power analysis for each of the analyses (P1 and P2 - please see the text in the document for details), as well as a description in the main Analysis Plan section for justification:
“Ability to detect actual effects: To begin to understand what kinds of effect sizes we will be able to detect given our sample size limitations, we used G*Power (v.3.1, @faul2007g, @faul2009statistical) to conduct power analyses based on confidence intervals. G*Power uses pre-set drop down menus and we chose the options that were as close to our analysis methods as possible (listed in each analysis below). Note that there were no explicit options for GLMMs, thus the power analyses are only an approximation of the kinds of effect sizes we can detect. We realize that these power analyses are not fully aligned with our study design and that these kinds of analyses are not appropriate for Bayesian statistics (e.g., our MCMCglmm below), however we are unaware of better options at this time. Additionally, it is difficult to run power analyses because it is unclear what kinds of effect sizes we should expect due to the lack of data on this species for these particular research questions.”
Reviewer 1 - Javier Seoane
Comment 1: This study addresses an old but still incompletely resolved research question, namely the relationship between body condition indexes and fitness. There has been some previous attempts to relate common body condition indexes (either based on morphological traits or on physiological measures) in birds to some proxies of fitness, such as reproductive success, with varying results. Often, a simple relationship has been questioned, and some studies have suggested (1) to consider multivariate indexes —instead of relying on a simple one—, (2) to look for non-linear patterns and (3) to regard indexes as proxies for short-term success (for example, see discussion in: Milenkaya et al 2015 [https://journals.plos.org/plosone/article?id=10.1371/journal.pone.0136582]; this is a reference that you may consider including on your bibliography). My first general recommendation to the authors is to consider these points.
Response 1: Thank you for this suggestion, and pointing us to this useful paper. We have incorporated the Milenkaya et al. 2015 paper, and we included the possibility of a nonlinear relationship, with a supporting citation, into the Abstract: “... However, there is mixed support regarding whether these condition indices relate to life history characteristics (@wilder2016moving; @labocha2014body), and whether the relationship shows a linear trend (@mcnamara2005theoretical; @milenkaya2015success)...”
Secondly, we added to our methods that we will plot our raw data to determine if there is evidence for a non-linear relationship between reproductive success and our body condition variable:
ANALYSIS PLAN > P2: “Previous research found a non-linear relationship between reproductive success and body condition variables (@milenkaya2015success). To check whether this is occuring in our data, we will first plot our raw data to determine if we need to include a non-linear body condition independent variable into our model (i.e. FatScore2). Our dependent variable is binary, so to more clearly see the trends in the data, on the x-axis we will bin our condition scores into 5 categories based on standard deviations (sd) around the mean (low = < 2 sd, moderately low = -2 sd to -1 sd, moderate = -1 sd to +1 sd, moderately high = +1 sd to +2 sd, high = > 2 sd). Then on the y-axis we will use the proportion of individuals in each category that had successful nests.”
The grackles are very difficult to re-catch to get repeated estimates of condition (as in Milenkaya et al. 2014, referenced in the discussion section that you suggested). As a result, we will have a gradient of time lags between the collection of our condition indices (measures of fat, weight and structural body size), and observations of reproductive success. The longest time period between these measures would be 1 year and 9 months, while the shortest time period could be less than a month. For example, we were still catching grackles for the first two months of the 2019 breeding season (April and May). We plan to model whether our condition indices systematically vary by season (Independent variables > P2 > Note 2). If so, this may indicate that condition indices relate to short-term success and we will only use data on condition indices of individuals measured during the breeding season.
Comment 2: I find the set of hypothesis is clearly stated and feasible to test, the methods suitable for the questions at hand. I was concerned about sampling size, though. The text states that “As of 30 July 2019, we have fledgling data for 14 females that exhibited breeding behavior (…) and breeding territory status for 9 males”. That is a worryingly low sample size. However, the text reads below that “The minimum sample size for H2 will be 57 marked grackles because that is how many individuals we have biometric and breeding behavior data for so far”. I am confused with this apparent contradiction (first sample size is given as 9+14=23 individuals and afterwards as 57) but think the authors are aware and will increment those numbers.
Response 2: Thank you for pointing this out. Currently, our sample size for reproductive success is small. However, we plan to augment our sample size by collecting more data during the 2020 breeding season. We clarified the sample size numbers for H1 and H2.
METHODS > Planned sample:
“Individuals included in this sample will be those for which we have measures of condition when they were adults. We will not include data that was collected on juvenile individuals. As of 30 July 2019, we have fledgling data for 14 females that exhibited breeding behavior (5 had 1+ fledgling, 9 had no fledglings) and breeding territory status for 10 males (7 territory holders, 3 non-territory holders, 2 not observed so not part of this sample). Therefore, the minimum sample size for H2 will be 24. The minimum sample size for H1 will be 72, because that is how many marked individuals we have biometric data for so far. However, we expect to be able to add to the sample sizes for both H1 and H2 before the end of this investigation in Tempe, Arizona.”
Furthermore, we revised METHODS > Sample size rationale as follows:
“We will continue to color mark as many grackles as possible, and collect biometric data and fat scores. Our current sample of reproductive success is small because the grackles in Tempe nest in very tall palm trees, making it difficult to determine nest status. However, we plan to collect additional reproductive success data during the breeding season in summer 2020.”
Comment 3: As for the statistical analyses, (1) I think it is likely that the range of fat scores measured on grackles will be quite narrow. Kaiser’s (1993) scores range from 0 to 8, but in my banding experience resident species or breeding birds —such as the grackles under study, I guess— tend to have fat scores within a limited range, say from 0 to 2 or 3. This may cause some difficulties to the correlations between fat and ratio weight/tarsus (maybe consider doing an anova-like linear model). If so, fat score should be best considered a factor (a categorical variable) for the models relating condition and reproductive success.
Response 3: You are correct that so far the range of fat scores is narrow, and we like the suggestion to consider it a factor instead of a count. Consequently, we have changed the family specification in our model for P1 to “ordinal”. We are unclear on what you mean by ANOVA-like linear model, though. Could you please provide us with further clarification if you think this is the more appropriate model?
We updated our preregistration in ANALYSIS PLAN > P1 analysis to read: “We use a Generalized Linear Mixed Model (GLMM; MCMCglmm function in the MCMCglmm package of @hadfield2010mcmcglmm) with an ordinal distribution (for categorical variables in MCMCglmm) and probit link using 130,000 iterations with a thinning interval of 10, a burnin of 30,000, and minimal priors (V=1, nu=0) (@hadfield2014coursenotes). We will ensure the GLMM shows acceptable convergence (lag time autocorrelation values less than 0.01 [@hadfield2010mcmcglmm]), and adjust parameters if necessary to meet this criterion. We will determine whether an independent variable had an effect or not using the estimate in the full model.”
Comment 4: (2) In the models relating condition and reproductive success it seems sensible to use Bird ID as a random effect. But the problem is if you end up with a set of data where most birds are sampled just once and a few are sampled two or, at most, three times. The estimate of the variance of the random effect may be imprecise and this could affect the standard errors (and correspondingly the p-values) of the fixed component of the model. If my description of that final set of data is correct, I suggest to build a linear model with just one observation per bird, no random effect, and check whether the results (the estimates of coefficients for the fixed effect) are similar in both models.
Response 4: Thank you for this comment, it has drawn our attention to the unusual characteristics of reproductive success as a dependent variable. In this model our dependent variable is nest success (yes or no), and 62% of the birds that we monitored that ever built a nest ultimately had multiple nests, potentially because the breeding season is long and nest failure rates seemed high. However, in response to your comment, we more deeply investigated the most appropriate way to model reproductive success by talking with a colleague that is more familiar with this type of data. They directed us to logistic exposure models (e.g., Shaffer 2004 https://www.jstor.org/stable/4090416?seq=1#page_scan_tab_contents). In typical logistic regression models, the survival rates are overestimated because nests that fail quickly are rarely found. In contrast, logistic exposure models use a different link function to determine the daily probability of nest survival while accounting for the fact that the probability of surviving depends on the interval length between nest checks. There is some concern that random effects included in these models are not yet appropriately specified (see https://rpubs.com/bbolker/logregexp).
Therefore, in our revised analysis for P2 we will use a mixed-effect logistic regression model with the typical logit-link as has been done by other researchers modeling reproductive success (e.g, Milenkaya et al. 2015), and we will additionally model the effect of condition on the probability that the nest survives for one day with the logistic exposure link function to validate whether the two analysis methods yield similar results. We’ve made these changes to the preregistration as follows:
ANALYSIS PLAN > P2 Analysis: “To model the effect of body condition on reproductive success, we will use two types of logistic mixed-effect models. Both types are supported in the literature, but are slightly different in the way in which the link function is specified. First, we will model reproductive success using a generalized linear mixed model framework with a logit link function (i.e. @milenkaya2015success). We will also use a logistic exposure model that has a link function which accounts for the time interval between nest checks when estimating the probability of daily nest survival (@shaffer2004unified).”
We added the following code:
```{r}
Mixed-effect logistic regression
Females
m1 <- MCMCglmm(Fledglings ~ FatScore + Aviary, random = ~Year + ID, family = "categorical", data = d, verbose = F, prior = prior, nitt = 130000, thin = 10, burnin = 30000)
summary(m1)
autocorr(m1$Sol) Did fixed effects converge?
autocorr(m1$VCV) Did random effects converge?
Males
m2 <- MCMCglmm(Territory ~ FatScore + Aviary, random = ~Year + ID, family = "categorical", data = d, verbose = F, prior = prior, nitt = 130000, thin = 10, burnin = 30000)
summary(m2)
autocorr(m2$Sol) Did fixed effects converge?
autocorr(m2$VCV) Did random effects converge?
Logistic exposure model, where “Exposure” is the number of days between nest checks
First run code for the exposure link function
library(MASS)
logexp <- function(exposure = 1) { get_exposure <- function() { if (exists("..exposure", env=.GlobalEnv)) return(get("..exposure", envir=.GlobalEnv)) exposure }
linkfun <- function(mu) qlogis(mu^(1/getexposure())) linkinv <- function(eta) plogis(eta)^getexposure() logitmueta <- function(eta) { ifelse(abs(eta)>30,.Machine$double.eps, exp(eta)/(1+exp(eta))^2) }
mu.eta <- function(eta) {
getexposure() * plogis(eta)^(getexposure()-1) *
logitmueta(eta)
}
valideta <- function(eta) TRUE link <- paste("logexp(", deparse(substitute(exposure)), ")", sep="") structure(list(linkfun = linkfun, linkinv = linkinv, mu.eta = mu.eta, valideta = valideta, name = link), class = "link-glm") }
Females
m3 <- glm(Fledglings ~ FatScore + Nest number + Aviary + (1|Year) + (1|ID), family = binomial, family=binomial(link=logexp(d$Exposure)), data = d, start=c(1,0))
summary(m3)
Males
m4 <- glm(Territory ~ FatScore + Aviary + (1|Year) + (1|ID), family = binomial, family=binomial(link=logexp(d$Exposure)), data = d, start=c(1,0))
summary(m4)
```
Comment 5: (3) P1 analysis. The description mentions the GLMM will use ‘binomial’ distribution, which is incorrect. However, the code shows that the GLMM will be built with ‘poisson’ distribution. This is acceptable as long as the ‘FatScore’ variable is a positive integer. Poisson distribution is used to model counts. Despite fat scores are really not counts, I think the resulting model could be sensible.
Response 5: Thank you for catching this, we have revised the text per your comment 3, which we copied and pasted here as well.
ANALYSIS PLAN > P1 Analysis: “We use a Generalized Linear Mixed Model (GLMM; MCMCglmm function in the MCMCglmm package of @hadfield2010mcmcglmm) with an ordinal distribution (for categorical variables in MCMCglmm) and probit link using 130,000 iterations with a thinning interval of 10, a burnin of 30,000, and minimal priors (V=1, nu=0) (@hadfield2014coursenotes). We will ensure the GLMM shows acceptable convergence (lag time autocorrelation values less than 0.01 [@hadfield2010mcmcglmm]), and adjust parameters if necessary to meet this criterion. We will determine whether an independent variable had an effect or not using the estimate in the full model.”
Comment 6: (4) P2 analysis. I think the following line to check whether body condition variables vary by season: “bs <- glm(Body ~ Season, family = "poisson", data = d)” Should be changed to “bs <- glm(Body ~ Season, family = “gaussian”, data = d)” Because the ‘Body’ variable is the ratio between weight and tarsus length, which is unlikely Poisson distributed.
Response 6: Thank you for this suggestion! We changed that line as you indicated.
Comment 7: I hope this helps.
Response 7: Your comments have been extremely helpful! We appreciate your help in making this a higher quality investigation.
Reviewer 2 - Isabel Lopez-Rull Comment 8: This is a nice proposal that aims to 1) compare two common methods of estimating body condition in birds and 2) evaluate whether body condition relates to reproductive success in the great-tailed grackle (Quiscalus mexicanus). The topic is attractive since many studies of animal ecology rely on different measures of body condition that are indicative of individual quality and are assumed to be fitness-related. On this basis I encourage the authors to collect the necessary data to accomplish their objectives. I have some comments and suggestions.
Response 8: Thank you for these positive comments, we look forward to addressing your suggestions.
Comment 9: Abstract: - The definition of “condition” is missing. Individual condition may not only refer to fat reserves but also to nutritional state, health, etc. It is important to define the key concept of the study and to highlight the importance of such a measure in animal ecology. - Change along the text “reproductive fitness” for “reproductive success” - “Theoretically, individuals that are in better condition should be able to disperse or migrate further or more successfully, have greater reproductive fitness, and survive for longer”. Not just “theoretically”, there is plenty of evidence that authors should review.
Response 9: This is good feedback, we clarified our definition of condition in the abstract, and incorporated additional background literature. For your convenience we’ve copied the changed and additional text below:
“… Research has shown that individuals that are in better "condition" can disperse or migrate further or more successfully, have greater reproductive success, and survive for longer (@wilder2016moving; @heidinger2010patch; @liao2011fat), particularly in years where environmental conditions are harsh (@milenkaya2015success). An individual's body condition can be defined in various ways, but is most often considered an individual's energetic or immune state (@milenkaya2015success). Since these traits are hard to measure directly, researchers have instead used a variety of morphological proxy variables to quantify condition such as … a scaled mass index (@pieg2009new), as well as hematological indices for immune system function (@fleskes2017body, @kraft2019developmental). … In this investigation, we will define condition as represented by an individual's energetic state to compare two indices (fat score and the scaled mass index) to validate whether they measure the same trait in our study system, the great-tailed grackle (Quiscalus mexicanus)...”
Comment 10: “Researchers have used a variety of morphological proxy variables to quantify condition…” Currently, most works use the ‘scaled mass index’ (CMI) when estimating body condition. CMI is a useful tool for ecologists because it is based on the central principle of scaling making the measurement more reliable. In fact, I suggest authors to work with CMI instead of using the ratio of weight to tarsus length. For more details on this method see Pieg&Green (2009) Oikos 118: 1883-1891.
Response 10: We appreciate you directing us towards this resource. The CMI does seem like an appropriate measure to use. We updated the abstract and the text throughout to include this measure, and stated in our methods:
METHODS > Independent Variables > P1:
“1) Scaled mass index using measures of body weight and tarsus length or flattened wing length (average of left and right as in @bleeker2005body). We will choose the measure that is most correlated with body weight (@pieg2009new).”
ANALYSIS PLAN > P1 Analysis > Analysis:
“We will calculate the scaled mass index as described by Pieg and Green (2009) using either tarsus or flattened wing length - whichever measure is most correlated with body weight (@pieg2009new).”
Comment 11: Please underline or italicize the scientific name of the species of study
Response 11: Thank you for catching that mistake! We made this change.
Comment 12: Hypotheses: “H1: There is a relationship between two different morphological indices of condition: fat score and the ratio of body weight to tarsus length” Prediction 1: Fat score and the ratio of weight to tarsus length will be positively correlated. This would indicate that these two indices measure the same trait, and it is likely they both are proxies for fat content. -As it is written, hypothesis 1 is not different from prediction 1. The underlined sentences are quite similar. The hypothesis would be that the two indices of body condition are measuring similar qualities. So, if both indices are similar we can predict that there would be a positive correlation between them. I suggest to re-write.
Response 12: We apologize if this was confusing! We made sure to use the word “relationship” in the hypothesis so that we could make predictions for any direction the relationship might take (positive, negative, or no correlation). While some predictions might be more plausible than others, we wanted to make sure that we could a priori account for any other outcomes that might arise.
Comment 13: “H2: Condition (as measured by fat score and the ratio of weight to tarsus length) relates to reproductive success (measured as a binary variable of whether a female had one or more fledglings (1) or not (0), and whether a male defended a territory containing nests (1) or not (0)). Prediction 2: Morphological indices of condition (fat score and the ratio of weight to tarsus length) will correlate positively with reproductive success. This would indicate that individuals with more fat, and therefore higher energy reserves, are better able to acquire the resources necessary for reproduction.” -Again, as it is written, hypothesis 2 is not different from prediction 2. The underlined sentences are quite similar. The hypothesis would be that the two individuals with more fat, and therefore higher energy reserves, are better able to acquire the resources necessary for reproduction. If so we can predict that there would be a positive correlation between reproductive success and indices of condition. I suggest to re-write.
Response 13: Please see our Response 12 - we used the neutral word “relates” in this instance.
Comment 14: I am not familiar with what authors call “alternative predictions”. In my opinion there is only one prediction that emerges from the prediction and, depending on the result, it may be or not, supported by data. In case the prediction is not supported two scenarios may arise, one is that the correlation occurs in the opposite sense of the prediction, and the other one is that there is no correlation. Depending on the results authors should interpret their data. I recognize that making “alternative predictions” is a good exercise to visualize the different results that can arise, but I personally don’t like the idea of presenting them in the manuscript.
Response 14: For each hypothesis, there are a number of results that could occur (e.g., positive, negative, or no correlations) and we wanted to make a priori predictions about how we would interpret every potential result from a given hypothesis. This prevents us from HARKing (Hypothesizing After Results are Known; see Kerr 1998), which could occur if we get a result that we weren’t expecting. In this case, we could then make up a post hoc story about why that result might have occurred. By a priori accounting for as many variations of the results that we can think of, it places our focus on being predictive in advance, which allows us to test these predictions in this study (see Nosek et al. 2019). If we didn’t list the alternatives at the pre-data collection stage, and we ended up encountering a result that was not in our predictions, we would be providing an interpretation post hoc, which would require us to conduct a new study to determine whether that prediction was supported. Another advantage to listing multiple alternatives in advance and having automated version tracking at GitHub with time and date stamps and track changes for all edits to the document is that readers can verify for themselves whether we were HARKing or not. Listing all potential predictions in advance allows us to explore the whole logical space that we are working in, rather than just describing one outcome possibility.
Nosek, B. A., Beck, E. D., Campbell, L., Flake, J. K., Hardwicke, T. E., Mellor, D. T., ... & Vazire, S. (2019). Preregistration Is Hard, And Worthwhile. Trends in cognitive sciences, 23(10), 815-818. Kerr, N. L. (1998). HARKing: Hypothesizing after the results are known. Personality and Social Psychology Review, 2(3), 196-217.
Comment 15: Methods: - It would be important to report the repeatability of the measurements, so I suggest that whenever possible authors should measure some individuals twice.
Response 15: We agree that obtaining repeatability of our measurements would be ideal. However, it is very difficult to catch these grackles even one time, and afterwards they are even less willing to go near our traps. Usually, the only individuals where we take two measures are those that we hold in aviaries for up to 6 months for behavioral tests (described in other preregistrations), and so we are able to measure them again before their release. While in the aviaries they receive a regular diet of nutritious food, which potentially alters their fat scores. However, we can use this subset of individuals to calculate the repeatability of our tarsus and flattened wing length measures. We have updated the preregistration as follows (changes noted in italics):
ANALYSIS PLAN:
“We will exclude data that was collected from the grackles when they were released from the aviaries to avoid any confounds due to their time in the aviary (e.g., perhaps unlimited nutritious food in the aviaries decreased their fat score). However, to validate that our measures of structural body size (tarsus length or wing length) are precise and accurate, we will measure twice a subset of grackles brought into aviaries - once when they are initially caught, and again up to 6 months later when we release them. We will then calculate the repeatability of these multiple measures. All other data included in this study will come only from wild-caught grackles (including the birds that were brought into the aviaries on their first capture).”
ANALYSIS PLAN > P1 analysis > Analysis:
“Where we have multiple measures of tarsus or flattened wing length, we will check that our measurements are repeatable using the rptR package (@stoffel2017rptr).”
We also added code for this analysis:
```{r}
which structural body size measure shows a higher correlation with body mass?
cor.test(tarsus,mass)
cor.test(wing,mass)
repeatability of structural body mass measurements ("Body" represents either wing length or tarsus length, whichever was more correlated with body mass)
rpt(log(Body) ~ (1|ID), grname = "ID", data = d, datatype = "Gaussian", nboot = 500, npermut = 500)
```
Comment 16: Given that the great-tailed grackle is a polygynous species and that there is considerable variation in the reproductive success among individuals, I wonder why do authors categorize the dependent variable in a binary way (whether a female had a fledgling or not and whether a male held a territory containing nests or not) instead of working with the number of fledglings/nests?
Response 16: Great-tailed grackles in our population in Arizona nest really high in palm trees. Therefore, we are unable to consistently determine how many eggs and nestlings are in the nest, or even how many active nests are in a tree. Additionally, sometimes we were never able to find the nest for a female, but then observed her later feeding a fledgling. Based on observations of individually color-marked females with known nesting status, we know that females do not feed fledglings that are not their own. As such, we use a binary variable for reproductive success to maximize our sample size.
Comment 17: It would be important to describe how are the male territories assigned? When authors evaluate if a male has a territory containing nests or not, I suggest to include in the analysis the size of the territory.
Response 17: During the breeding season males spend the majority of their time sitting and singing in one to three tall date palms that the females like to nest in. The majority of males in our sample only defended one palm as their breeding territory. Males that defended multiple palms occurred in areas where the nesting palms were clumped, with only 2 males defending palms that were at most 40m apart.
When a color-marked male is seen in the same palm(s) for more than one day, we determine that he is defending that palm as his breeding territory. Males rarely change the location of their breeding palms. In our two breeding seasons of observing color-marked males, observing them singing in a palm on two consecutive days tends to be predictive of their territorial behavior for the rest of the breeding season.
We clarified this in our definition of the reproductive success variables as follows (changed text noted in italics):
METHODS > Dependent variables > P2:
“2) Male held territory consisting of 1 to 3 clumped palms containing at least one active nest (yes, no)”
Comment 18: Analyses plan: - “We will exclude data that was collected from the grackles when they were released from the aviaries to avoid any confounds due to their time in the aviary (e.g., perhaps unlimited nutritious food in the aviaries decreased their fat score)”. If so, I don’t understand why “Temporarily held in aviaries for behavioral testing at any point during this study (yes, no)” is included as an independent variable in the analysis.
Response 18: Thank you for catching this, we see that the wording is confusing. We mean that we will exclude measures of fat score and mass that are taken when we release grackles from the aviaries. However, we will include all nest success and tarsus or wing length (see Response 15) data from these individuals. Therefore, we decided to account for any behavioral changes that may affect reproductive success, occurring as a result of time spent in aviaries, with this independent variable.
To modify this in the preregistration, we removed “Temporarily held in the aviaries” from the independent variables for P1. We also clarified this independent variable in P2 as:
2) Temporarily held in aviaries for behavioral testing at any point during this study, because this may affect breeding behavior (yes, no)
Comment 19: “P1 analysis: correlation between fat and the ratio of weight to tarsus length Analysis: We use a Generalized Linear Mixed Model (GLMM; MCMCglmm function, MCMCglmm package; (Hadfield 2010)) with a binomial distribution (called “categorical” in MCMCglmm) and log link…” I think is logit link not log link.
Response 19: Thank you for noting this, you are correct. However, we changed this model to use an ordinal distribution in response to Comment 3, above.
Comment 20: Other comments: - Finally, style and grammar must be checked along the manuscript.
Response 20: To address this comment we have reviewed all writing in the manuscript and asked someone to edit it that has not reviewed it before. We hope you will find it improved.
Decision by Marcos Mendez, posted 28 Sep 2019
Dear Dr. Berens and coauthors,
Your preprint has been carefully reviewed by two experts and myself. Both experts find value in your proposal but suggest several modifications and additions. I concur with their suggestions and therefore the current version cannot be recommended, but I invite you to submit a revised version that incorporates the suggestions made by the reviewers. In particular, the new version should pay attention to a proper definition of condition, to consider additional literature in the abstract, suggested by one of the reviewers. The election of the two conditions indexes addressed should be motivated, as one of the experts suggests that CMI may be more adequate. Consider to reframe or reword your hypotheses, to distinguisth them from your predictions. Clarify the current and future sample size (I add here that even if 57 individuals are finally available, splitting the data into male and female grackles still may be a relatively los sample size), and the repeatability of the measures. In the statistical analysis, the new version should clarify how repeated measures (expectedly few) will be handled, correct some mistakes in the models (Poisson rather than binomial for testing H1) and, given your limited sample size, I may add that a power analysis would be advisable. Details are provided in the comments by the reviewers.
I look forward to a new version of the proposal.
Sincerely,
Marcos Méndez
Reviewed by Javier Seoane, 09 Sep 2019
This study addresses an old but still incompletely resolved research question, namely the relationship between body condition indexes and fitness. There has been some previous attempts to relate common body condition indexes (either based on morphological traits or on physiological measures) in birds to some proxies of fitness, such as reproductive success, with varying results. Often, a simple relationship has been questioned, and some studies have suggested (1) to consider multivariate indexes —instead of relying on a simple one—, (2) to look for non-linear patterns and (3) to regard indexes as proxies for short-term success (for example, see discussion in: Milenkaya et al 2015 [https://journals.plos.org/plosone/article?id=10.1371/journal.pone.0136582]; this is a reference that you may consider including on your bibliography). My first general recommendation to the authors is to consider these points.
I find the set of hypothesis is clearly stated and feasible to test, the methods suitable for the questions at hand. I was concerned about sampling size, though. The text states that “As of 30 July 2019, we have fledgling data for 14 females that exhibited breeding behavior (…) and breeding territory status for 9 males”. That is a worryingly low sample size. However, the text reads below that “The minimum sample size for H2 will be 57 marked grackles because that is how many individuals we have biometric and breeding behavior data for so far”. I am confused with this apparent contradiction (first sample size is given a as 9+14=23 individuals and afterwards as 57) but think the authors are aware and will increment those numbers.
As for the statistical analyses, (1) I think it is likely that the range of fat scores measured on grackles will be quite narrow. Kaiser’s (1993) scores range from 0 to 8, but in my banding experience resident species or breeding birds —such as the grackles under study, I guess— tend to have fat scores within a limited range, say from 0 to 2 or 3. This may cause some difficulties to the correlations between fat and ratio weight/tarsus (maybe consider doing an anova-like linear model). If so, fat score should be best considered a factor (a categorical variable) for the models relating condition and reproductive success. (2) In the models relating condition and reproductive success it seems sensible to use Bird ID as a random effect. But the problem is if you end up with a set of data where most birds are sampled just once and a few are sampled two or, at most, three times. The estimate of the variance of the random effect may be imprecise and this could affect the standard errors (and correspondingly the p-values) of the fixed component of the model. If my description of that final set of data is correct, I suggest to build a linear model with just one observation per bird, no random effect, and check whether the results (the estimates of coefficients for the fixed effect) are similar in both models. (3) P1 analysis. The description mentions the GLMM will use ‘binomial’ distribution, which is incorrect. However, the code shows that the GLMM will be built with ‘poisson’ distribution. This is acceptable as long as the ‘FatScore’ variable is a positive integer. Poisson distribution is used to model counts. Despite fat scores are really not counts, I think the resulting model could be sensible. (4) P2 analysis. I think the following line to check whether body condition variables vary by season: “bs <- glm(Body ~ Season, family = "poisson", data = d)” Should be changed to “bs <- glm(Body ~ Season, family = “gaussian”, data = d)” Because the ‘Body’ variable is the ratio between weight and tarsus length, which is unlikely Poisson distributed.
I hope this helps.
Reviewed by Isabel López-Rull, 25 Sep 2019
This is a nice proposal that aims to 1) compare two common methods of estimating body condition in birds and 2) evaluate whether body condition relates to reproductive success in the great-tailed grackle (Quiscalus mexicanus). The topic is attractive since many studies of animal ecology rely on different measures of body condition that are indicative of individual quality and are assumed to be fitness-related. On this basis I encourage the authors to collect the necessary data to accomplish their objectives. I have some comments and suggestions.
Abstract: - The definition of “condition” is missing. Individual condition may not only refer to fat reserves but also to nutritional state, health, etc. It is important to define the key concept of the study and to highlight the importance of such a measure in animal ecology. - Change along the text “reproductive fitness” for “reproductive success” - “Theoretically, individuals that are in better condition should be able to disperse or migrate further or more successfully, have greater reproductive fitness, and survive for longer”. Not just “theoretically”, there is plenty of evidence that authors should review. - “Researchers have used a variety of morphological proxy variables to quantify condition…” Currently, most works use the ‘scaled mass index’ (CMI) when estimating body condition. CMI is a useful tool for ecologists because it is based on the central principle of scaling making the measurement more reliable. In fact, I suggest authors to work with CMI instead of using the ratio of weight to tarsus length. For more details on this method see Pieg&Green (2009) Oikos 118: 1883-1891. - Please underline or italicize the scientific name of the species of study
Hypotheses: “H1: There is a relationship between two different morphological indices of condition: fat score and the ratio of body weight to tarsus length” Prediction 1: Fat score and the ratio of weight to tarsus length will be positively correlated. This would indicate that these two indices measure the same trait, and it is likely they both are proxies for fat content. -As it is written, hypothesis 1 is not different from prediction 1. The underlined sentences are quite similar. The hypothesis would be that the two indices of body condition are measuring similar qualities. So, if both indices are similar we can predict that there would be a positive correlation between them. I suggest to re-write. “H2: Condition (as measured by fat score and the ratio of weight to tarsus length) relates to reproductive success (measured as a binary variable of whether a female had one or more fledglings (1) or not (0), and whether a male defended a territory containing nests (1) or not (0)). Prediction 2: Morphological indices of condition (fat score and the ratio of weight to tarsus length) will correlate positively with reproductive success. This would indicate that individuals with more fat, and therefore higher energy reserves, are better able to acquire the resources necessary for reproduction.” -Again, as it is written, hypothesis 2 is not different from prediction 2. The underlined sentences are quite similar. The hypothesis would be that the two individuals with more fat, and therefore higher energy reserves, are better able to acquire the resources necessary for reproduction. If so we can predict that there would be a positive correlation between reproductive success and indices of condition. I suggest to re-write. - I am not familiar with what authors call “alternative predictions”. In my opinion there is only one prediction that emerges from the prediction and, depending on the result, it may be or not, supported by data. In case the prediction is not supported two scenarios may arise, one is that the correlation occurs in the opposite sense of the prediction, and the other one is that there is no correlation. Depending on the results authors should interpret their data. I recognize that making “alternative predictions” is a good exercise to visualize the different results that can arise, but I personally don’t like the idea of presenting them in the manuscript.
Methods: - It would be important to report the repeatability of the measurements, so I suggest that whenever possible authors should measure some individuals twice. - Given that the great-tailed grackle is a polygynous species and that there is considerable variation in the reproductive success among individuals, I wonder why do authors categorize the dependent variable in a binary way (whether a female had a fledgling or not and whether a male held a territory containing nests or not) instead of working with the number of fledglings/nests? -It would be important to describe how are the male territories assigned? When authors evaluate if a male has a territory containing nests or not, I suggest to include in the analysis the size of the territory.
Analyses plan: - “We will exclude data that was collected from the grackles when they were released from the aviaries to avoid any confounds due to their time in the aviary (e.g., perhaps unlimited nutritious food in the aviaries decreased their fat score)”. If so, I don’t understand why “Temporarily held in aviaries for behavioral testing at any point during this study (yes, no)” is included as an independent variable in the analysis. - “P1 analysis: correlation between fat and the ratio of weight to tarsus length Analysis: We use a Generalized Linear Mixed Model (GLMM; MCMCglmm function, MCMCglmm package; (Hadfield 2010)) with a binomial distribution (called “categorical” in MCMCglmm) and log link…” I think is logit link not log link.
Other comments: - Finally, style and grammar must be checked along the manuscript.