
HUETTMANN Falk
Recommendations: 0
Review: 1
Review: 1
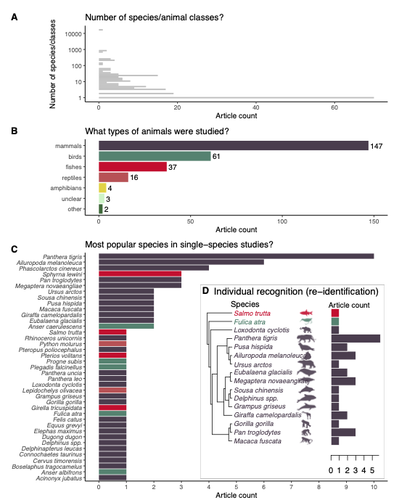
Rapid literature mapping on the recent use of machine learning for wildlife imagery
Review of machine learning uses for the analysis of images on wildlife
Recommended by Olivier Gimenez based on reviews by Falk Huettmann and 1 anonymous reviewerIn the field of ecology, there is a growing interest in machine (including deep) learning for processing and automatizing repetitive analyses on large amounts of images collected from camera traps, drones and smartphones, among others. These analyses include species or individual recognition and classification, counting or tracking individuals, detecting and classifying behavior. By saving countless times of manual work and tapping into massive amounts of data that keep accumulating with technological advances, machine learning is becoming an essential tool for ecologists. We refer to recent papers for more details on machine learning for ecology and evolution (Besson et al. 2022, Borowiec et al. 2022, Christin et al. 2019, Goodwin et al. 2022, Lamba et al. 2019, Nazir & Kaleem 2021, Perry et al. 2022, Picher & Hartig 2023, Tuia et al. 2022, Wäldchen & Mäder 2018).
In their paper, Nakagawa et al. (2023) conducted a systematic review of the literature on machine learning for wildlife imagery. Interestingly, the authors used a method unfamiliar to ecologists but well-established in medicine called rapid review, which has the advantage of being quickly completed compared to a fully comprehensive systematic review while being representative (Lagisz et al., 2022). Through a rigorous examination of more than 200 articles, the authors identified trends and gaps, and provided suggestions for future work. Listing all their findings would be counterproductive (you’d better read the paper), and I will focus on a few results that I have found striking, fully assuming a biased reading of the paper. First, Nakagawa et al. (2023) found that most articles used neural networks to analyze images, in general through collaboration with computer scientists. A challenge here is probably to think of teaching computer vision to the generations of ecologists to come (Cole et al. 2023). Second, the images were dominantly collected from camera traps, with an increase in the use of aerial images from drones/aircrafts that raise specific challenges. Third, the species concerned were mostly mammals and birds, suggesting that future applications should aim to mitigate this taxonomic bias, by including, e.g., invertebrate species. Fourth, most papers were written by authors affiliated with three countries (Australia, China, and the USA) while India and African countries provided lots of images, likely an example of scientific colonialism which should be tackled by e.g., capacity building and the involvement of local collaborators. Last, few studies shared their code and data, which obviously impedes reproducibility. Hopefully, with the journals’ policy of mandatory sharing of codes and data, this trend will be reversed.
REFERENCES
Besson M, Alison J, Bjerge K, Gorochowski TE, Høye TT, Jucker T, Mann HMR, Clements CF (2022) Towards the fully automated monitoring of ecological communities. Ecology Letters, 25, 2753–2775. https://doi.org/10.1111/ele.14123
Borowiec ML, Dikow RB, Frandsen PB, McKeeken A, Valentini G, White AE (2022) Deep learning as a tool for ecology and evolution. Methods in Ecology and Evolution, 13, 1640–1660. https://doi.org/10.1111/2041-210X.13901
Christin S, Hervet É, Lecomte N (2019) Applications for deep learning in ecology. Methods in Ecology and Evolution, 10, 1632–1644. https://doi.org/10.1111/2041-210X.13256
Cole E, Stathatos S, Lütjens B, Sharma T, Kay J, Parham J, Kellenberger B, Beery S (2023) Teaching Computer Vision for Ecology. https://doi.org/10.48550/arXiv.2301.02211
Goodwin M, Halvorsen KT, Jiao L, Knausgård KM, Martin AH, Moyano M, Oomen RA, Rasmussen JH, Sørdalen TK, Thorbjørnsen SH (2022) Unlocking the potential of deep learning for marine ecology: overview, applications, and outlook†. ICES Journal of Marine Science, 79, 319–336. https://doi.org/10.1093/icesjms/fsab255
Lagisz M, Vasilakopoulou K, Bridge C, Santamouris M, Nakagawa S (2022) Rapid systematic reviews for synthesizing research on built environment. Environmental Development, 43, 100730. https://doi.org/10.1016/j.envdev.2022.100730
Lamba A, Cassey P, Segaran RR, Koh LP (2019) Deep learning for environmental conservation. Current Biology, 29, R977–R982. https://doi.org/10.1016/j.cub.2019.08.016
Nakagawa S, Lagisz M, Francis R, Tam J, Li X, Elphinstone A, Jordan N, O’Brien J, Pitcher B, Sluys MV, Sowmya A, Kingsford R (2023) Rapid literature mapping on the recent use of machine learning for wildlife imagery. EcoEvoRxiv, ver. 4 peer-reviewed and recommended by Peer Community in Ecology. https://doi.org/10.32942/X2H59D
Nazir S, Kaleem M (2021) Advances in image acquisition and processing technologies transforming animal ecological studies. Ecological Informatics, 61, 101212. https://doi.org/10.1016/j.ecoinf.2021.101212
Perry GLW, Seidl R, Bellvé AM, Rammer W (2022) An Outlook for Deep Learning in Ecosystem Science. Ecosystems, 25, 1700–1718. https://doi.org/10.1007/s10021-022-00789-y
Pichler M, Hartig F Machine learning and deep learning—A review for ecologists. Methods in Ecology and Evolution, n/a. https://doi.org/10.1111/2041-210X.14061
Tuia D, Kellenberger B, Beery S, Costelloe BR, Zuffi S, Risse B, Mathis A, Mathis MW, van Langevelde F, Burghardt T, Kays R, Klinck H, Wikelski M, Couzin ID, van Horn G, Crofoot MC, Stewart CV, Berger-Wolf T (2022) Perspectives in machine learning for wildlife conservation. Nature Communications, 13, 792. https://doi.org/10.1038/s41467-022-27980-y
Wäldchen J, Mäder P (2018) Machine learning for image-based species identification. Methods in Ecology and Evolution, 9, 2216–2225. https://doi.org/10.1111/2041-210X.13075